Asymptotic Performance of Sparse Signal Detection Using Convex Programming Method
Chinese Journal of Aeronautics(2012)
摘要
The detection of sparse signals against background noise is considered. Detecting signals of such kind is difficult since only a small portion of the signal carries information. Prior knowledge is usually assumed to ease detection. In this paper, we consider the general unknown and arbitrary sparse signal detection problem when no prior knowledge is available. Under a Neyman-Pearson hypothesis-testing framework, a new detection scheme is proposed by combining a generalized likelihood ratio test (GLRT)-like test statistic and convex programming methods which directly exploit sparsity in an underdetermined system of linear equations. We characterize large sample behavior of the proposed method by analyzing its asymptotic performance. Specifically, we give the condition for the Chernoff-consistent detection which shows that the proposed method is very sensitive to the ℓ2 norm energy of the sparse signals. Both the false alarm rate and the miss rate tend to zero at vanishing signal-to-noise ratio (SNR), as long as the signal energy grows at least logarithmically with the problem dimension. Next we give a large deviation analysis to characterize the error exponent for the Neyman-Pearson detection. We derive the oracle error exponent assuming signal knowledge. Then we explicitly derive the error exponent of the proposed scheme and compare it with the oracle exponent. We complement our study with numerical experiments, showing that the proposed method performs in the vicinity of the likelihood ratio test (LRT) method in the finite sample scenario and the error probability degrades exponentially with the number of observations.
更多查看译文
关键词
signal detection,convex programming,asymptotic analysis,signal reconstruction,sparse signals
AI 理解论文
溯源树
样例
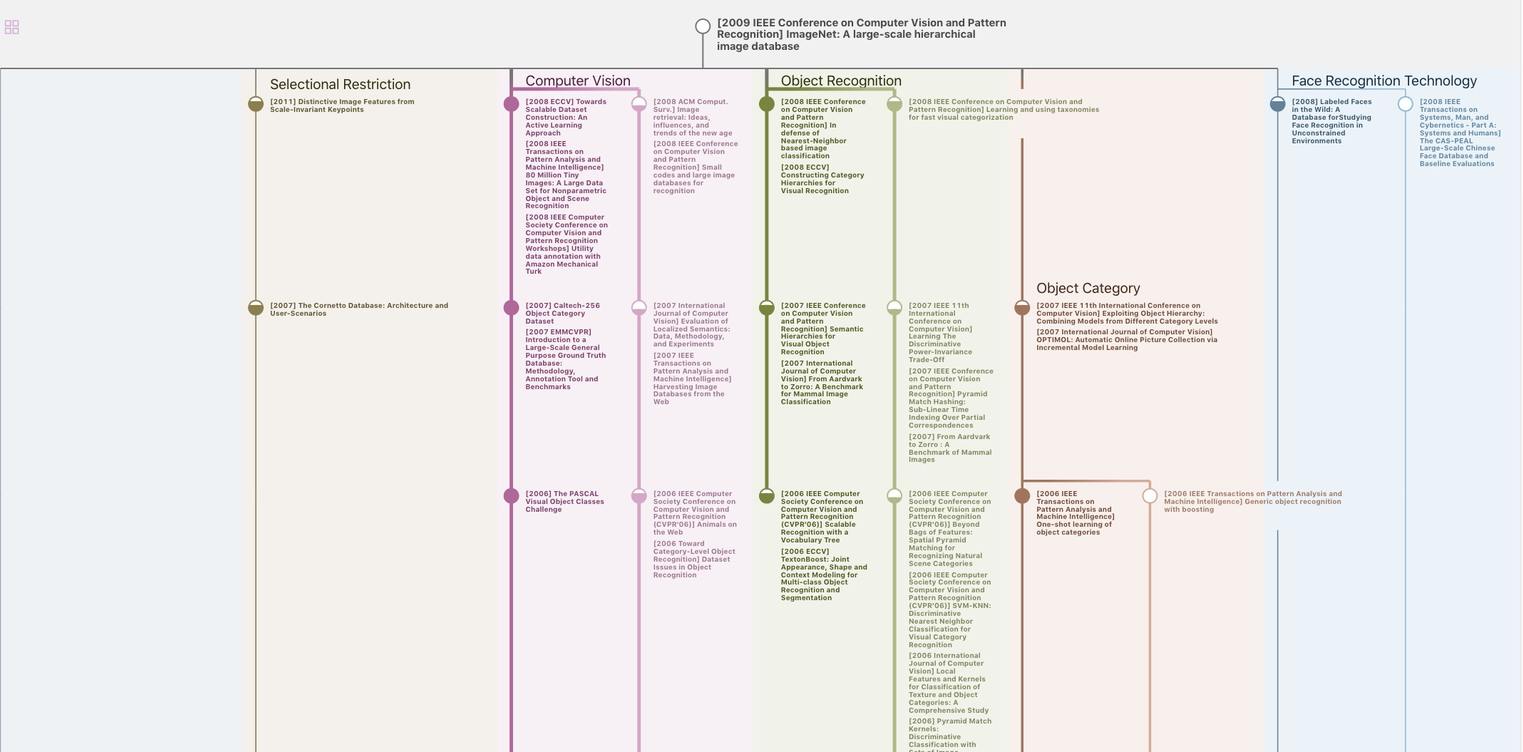
生成溯源树,研究论文发展脉络
Chat Paper
正在生成论文摘要