Recursive Computation of Value-at-Risk and Conditional Value-at-Risk using MC and QMC
MONTE CARLO AND QUASI-MONTE CARLO METHODS 2008(2009)
摘要
Value-at-Risk (VaR) and Conditional-Value-at-Risk (CVaR) are two widely-used measures in risk management. This paper deals with the problem of estimating both VaR and CVaR using stochastic approximation (with decreasing steps): we propose a first Robbins-Monro (RM) procedure based on Rockafellar-Uryasev's identity for the CVaR. The estimator provided by the algorithm satisfies a Gaussian Central Limit Theorem. As a second step, in order to speed up the initial procedure, we propose a recursive and adaptive importance sampling (IS) procedure which induces a significant variance reduction of both VaR and CVaR procedures. This idea, which has been investigated by many authors, follows a new approach introduced in Lemaire and Pages [20]. Finally, to speed up the initialization phase of the IS algorithm, we replace the original confidence level of the VaR by a deterministic moving risk level. We prove that the weak convergence rate of the resulting procedure is ruled by a Central Limit Theorem with minimal variance and we illustrate its efficiency by considering typical energy portfolios.
更多查看译文
关键词
Importance Sampling, Stochastic Approximation, Variance Reduction, Asymptotic Variance, Stochastic Approximation Algorithm
AI 理解论文
溯源树
样例
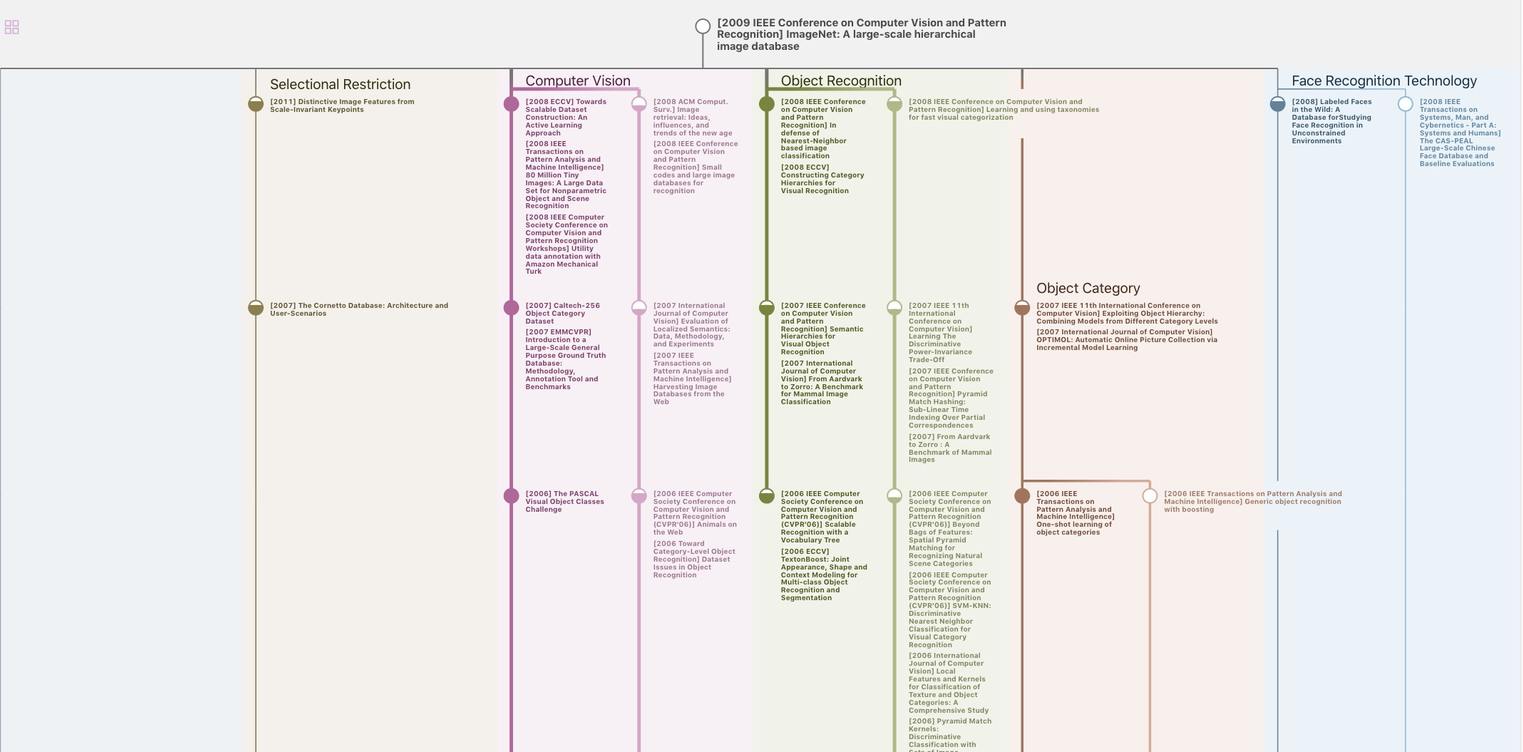
生成溯源树,研究论文发展脉络
Chat Paper
正在生成论文摘要