Multiple human tracking in high-density crowds
Image and Vision Computing(2012)
摘要
In this paper, we introduce a fully automatic algorithm to detect and track multiple humans in high-density crowds in the presence of extreme occlusion. Typical approaches such as background modeling and body part-based pedestrian detection fail when most of the scene is in motion and most body parts of most of the pedestrians are occluded. To overcome this problem, we integrate human detection and tracking into a single framework and introduce a confirmation-by-classification method for tracking that associates detections with tracks, tracks humans through occlusions, and eliminates false positive tracks. We use a Viola and Jones AdaBoost detection cascade, a particle filter for tracking, and color histograms for appearance modeling. To further reduce false detections due to dense features and shadows, we introduce a method for estimation and utilization of a 3D head plane that reduces false positives while preserving high detection rates. The algorithm learns the head plane from observations of human heads incrementally, without any a priori extrinsic camera calibration information, and only begins to utilize the head plane once confidence in the parameter estimates is sufficiently high. In an experimental evaluation, we show that confirmation-by-classification and head plane estimation together enable the construction of an excellent pedestrian tracker for dense crowds.
更多查看译文
关键词
false detection,associates detection,false positive,high-density crowd,false positive track,pedestrian detection,head plane estimation,head plane,jones adaboost detection cascade,multiple human tracking,human detection,high detection rate,particle filter,color histogram
AI 理解论文
溯源树
样例
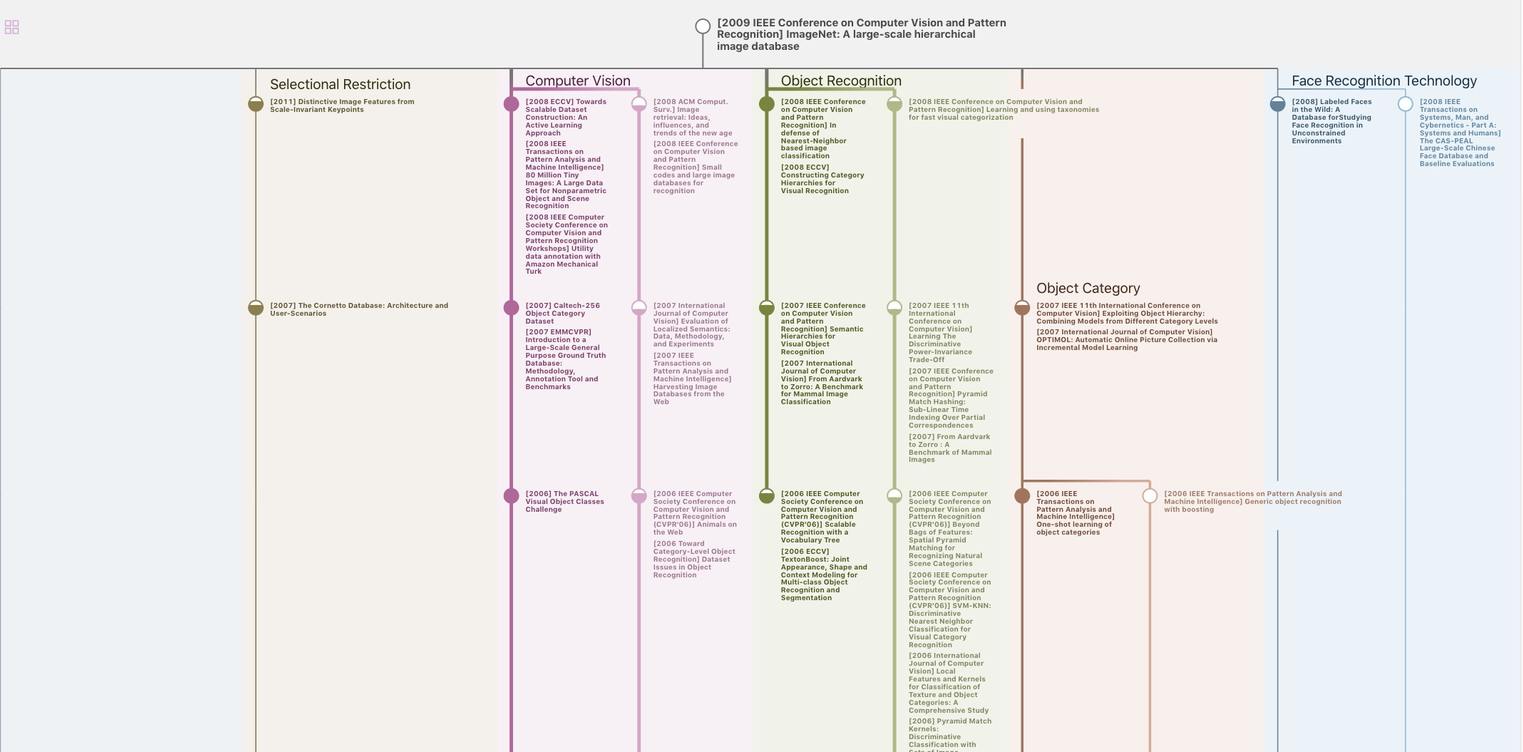
生成溯源树,研究论文发展脉络
Chat Paper
正在生成论文摘要