A neural network-based method for risk factor analysis of West Nile virus.
RISK ANALYSIS(2008)
摘要
There is a lack of knowledge about which risk factors are more important in West Nile virus (WNV) transmission and risk magnitude. A better understanding of the risk factors is of great help in developing effective new technologies and appropriate prevention strategies for WNV infection. A contribution analysis of all risk factors in WNV infection would identify those major risk factors. Based on the identified major risk factors, measures to control WNV proliferation could be directed toward those significant risk factors, thus improving the effectiveness and efficiency in developing WNV control and prevention strategies. Neural networks have many generally accepted advantages over conventional analytical techniques, for instance, ability to automatically learn the relationship between the inputs and outputs from training data, powerful generalization ability, and capability of handling nonlinear interactions. In this article, a neural network model was developed for analysis of risk factors in WNV infection. To reveal the relative contribution of the input variables, the neural network was trained using an algorithm called structural learning with forgetting. During the learning, weak neural connections are forced to fade away while a skeletal network with strong connections emerges. The significant risk factors can be identified by analyzing this skeletal network. The proposed approach is tested with the dead bird surveillance data in Ontario, Canada. The results demonstrate the effectiveness of the proposed approach.
更多查看译文
关键词
neural network,risk factor analysis,structural learning with forgetting,West Nile virus
AI 理解论文
溯源树
样例
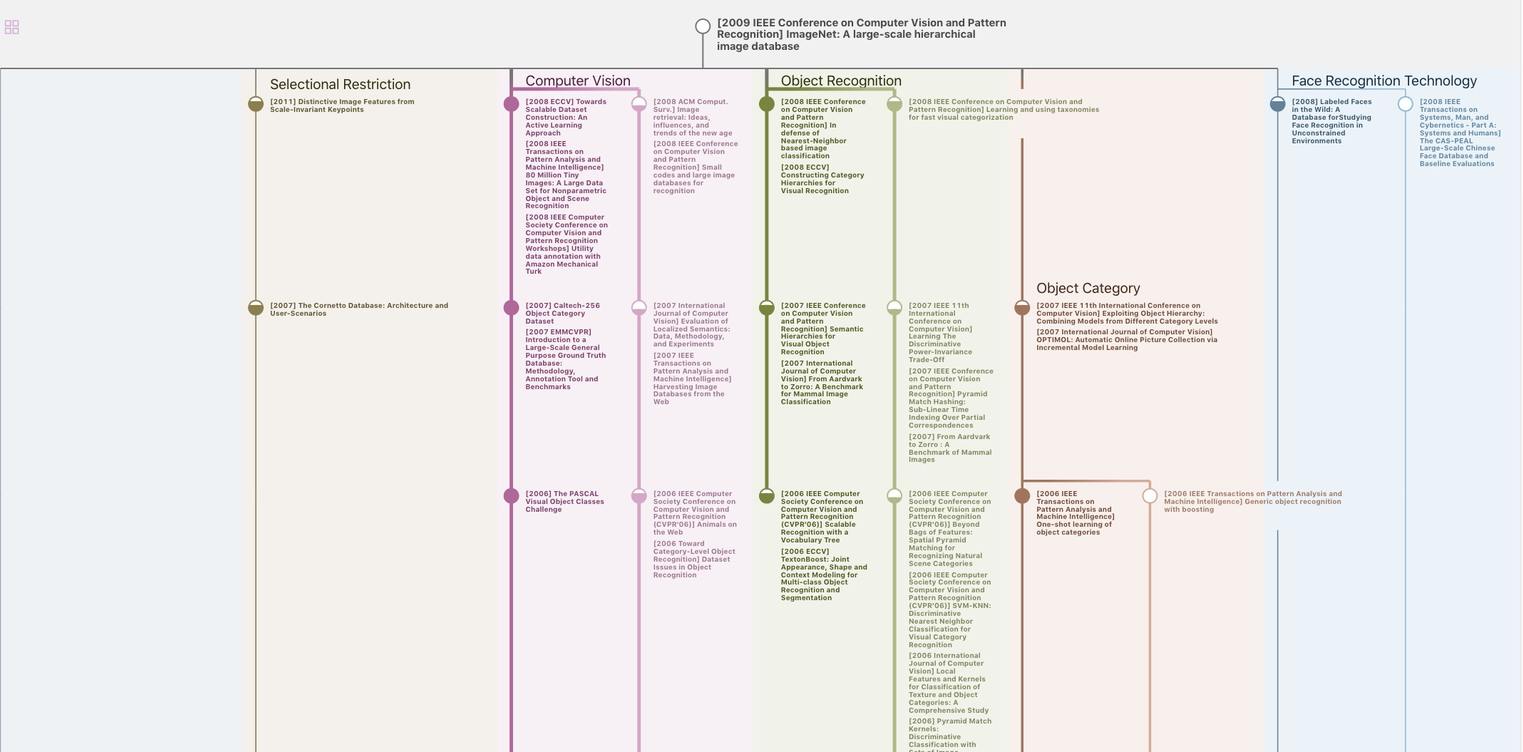
生成溯源树,研究论文发展脉络
Chat Paper
正在生成论文摘要