Estimates of constrained multi-class a posteriori probabilities in time series problems with neural networks
IJCNN(1999)
摘要
In time series problems, where time ordering is a crucial issue, the use of Partial Liklihood Estimation (PLE) represents a specially suitable method for the estimation of parameters in the model. We propose a new general supervised neural network algorithm, Joint Network and Data Density Estimation (XWDE), that employs PLE to approximate conditional probability density finctions for multi-class classification problems. The logistic regression analysis is generalized to multiple class problems with sofhnm regression neural network used to model the a- posteriori probabilities such that they are approximated by the network outputs. Constraints to the network architecture, as well m to the model of data, are imposed resulting in both a jlaible network architecture and distribution modeling. We consider application of JNDDE to channel equalization andpresent simulation results.
更多查看译文
关键词
neural network,neural networks,logistics,computer science,intelligent networks,statistical analysis,regression analysis,maximum likelihood estimation,network architecture,data models,parameter estimation,neural nets,time series,channel equalization,multi class classification,logistic regression analysis,amplitude modulation,density estimation,learning artificial intelligence,history,conditional probability,probability,signal processing
AI 理解论文
溯源树
样例
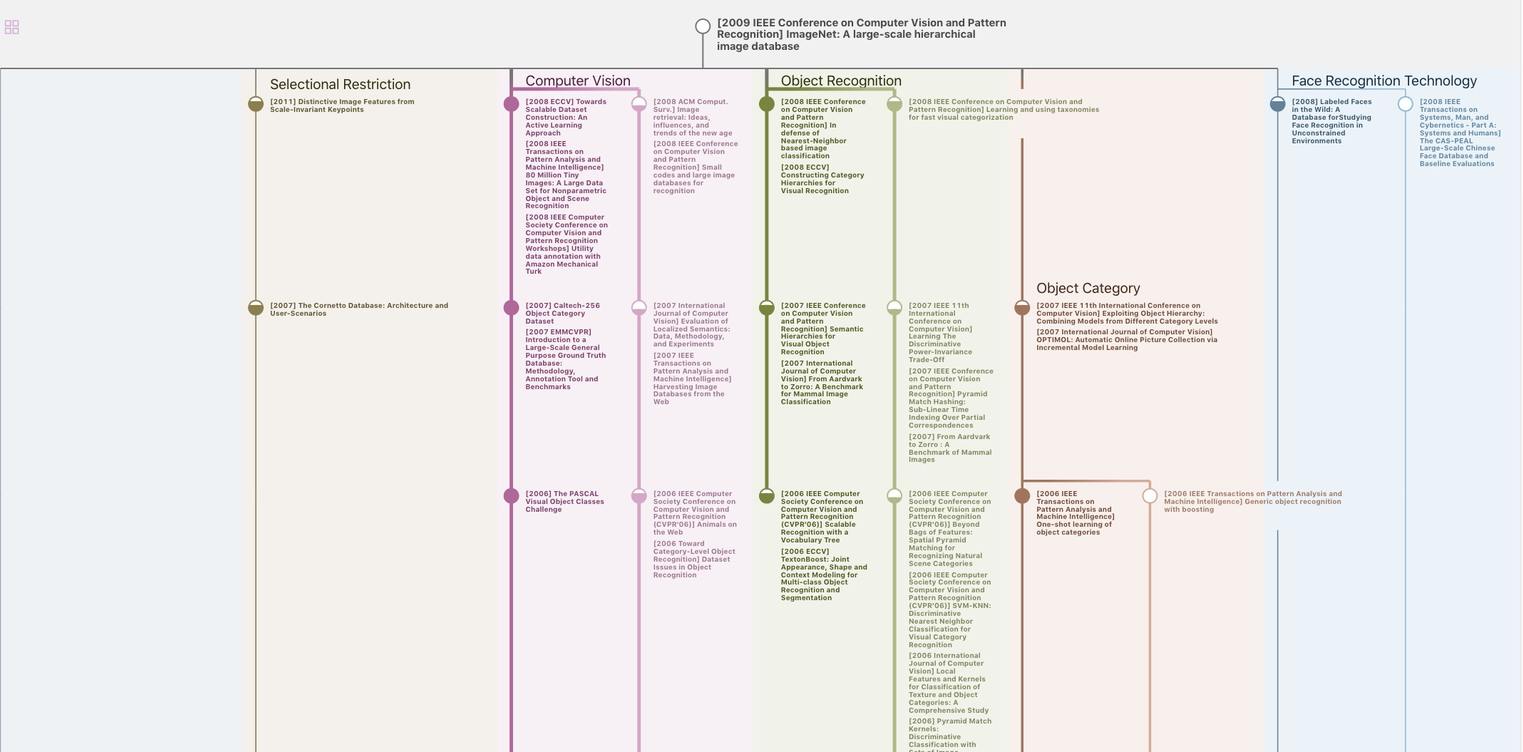
生成溯源树,研究论文发展脉络
Chat Paper
正在生成论文摘要