Motion pattern analysis in crowded scenes based on hybrid generative-discriminative feature maps
ICIP(2013)
摘要
Crowded scene analysis is becoming increasingly popular in computer vision field. In this paper, we propose a novel approach to analyze motion patterns by clustering the hybrid generative-discriminative feature maps using unsupervised hierarchical clustering algorithm. The hybrid generative-discriminative feature maps are derived by posterior divergence based on the tracklets which are captured by tracking dense points with three effective rules. The feature maps effectively associate low-level features with the semantical motion patterns by exploiting the hidden information in crowded scenes. Motion pattern analyzing is implemented in a completely unsupervised way and the feature maps are clustered automatically through hierarchical clustering algorithm building on the basis of graphic model. The experiment results precisely reveal the distributions of motion patterns in current crowded videos and demonstrate the effectiveness of our approach.
更多查看译文
关键词
posterior divergence,unsupervised hierarchical clustering algorithm,video signal processing,pattern clustering,semantical motion patterns,the hybrid generative-discriminative feature maps,crowded videos,motion pattern analysis,graphic model,motion pattern,tracklet,crowded scene analysis,computer vision,low-level features,hybrid generative-discriminative feature maps,tracklets,automatic clustering,image motion analysis
AI 理解论文
溯源树
样例
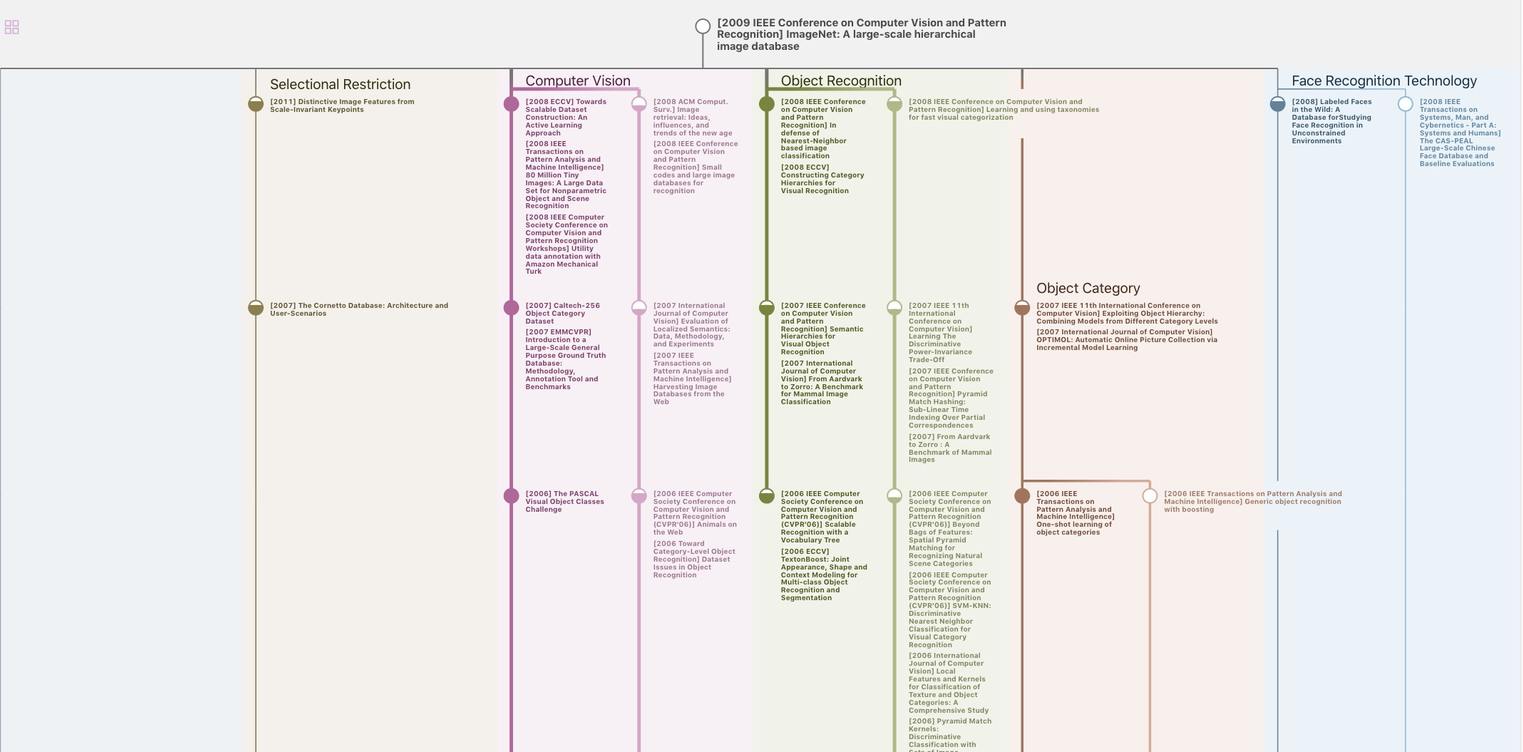
生成溯源树,研究论文发展脉络
Chat Paper
正在生成论文摘要