Inferring Users' Preferences from Crowdsourced Pairwise Comparisons: A Matrix Completion Approach.
HCOMP(2013)
摘要
Inferring user preferences over a set of items is an important problem that has found numerous applications. This work focuses on the scenario where the explicit feature representation of items is unavailable, a setup that is similar to collaborative filtering. In order to learn a user's preferences from his/her response to only a small number of pairwise comparisons, we propose to leverage the pairwise comparisons made by many crowd users, a problem we refer to as crowdranking. The proposed crowdranking framework is based on the theory of matrix completion, and we present efficient algorithms for solving the related optimization problem. Our theoretical analysis shows that, on average, only O(r log m) pairwise queries are needed to accurately recover the ranking list of m items for the target user, where r is the rank of the unknown rating matrix, r << m. Our empirical study with two real-world benchmark datasets for collaborative filtering and one crowdranking dataset we collected via Amazon Mechanical Turk shows the promising performance of the proposed algorithm compared to the state-of-the-art approaches.
更多查看译文
AI 理解论文
溯源树
样例
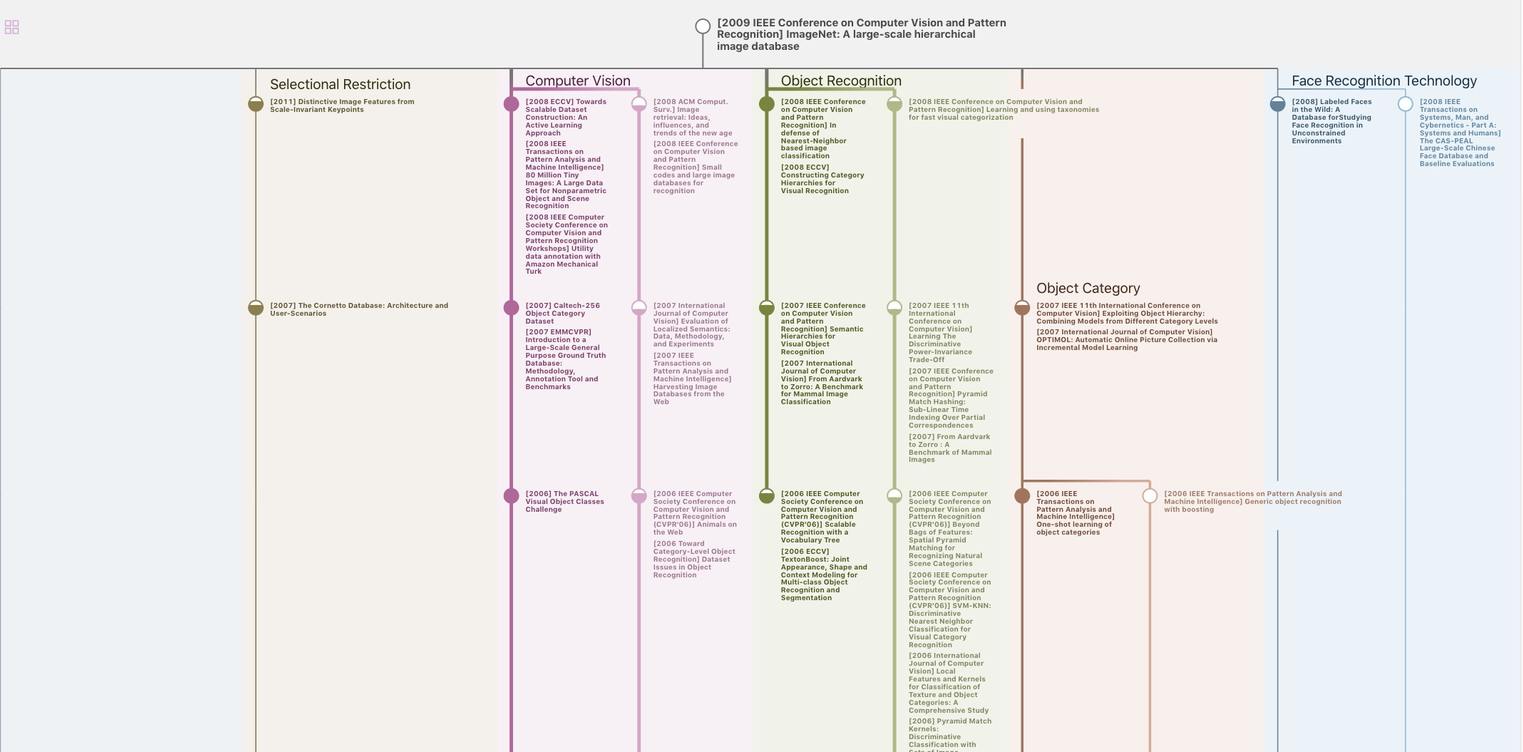
生成溯源树,研究论文发展脉络
Chat Paper
正在生成论文摘要