Conditions for Convergence in Regularized Machine Learning Objectives
CoRR(2013)
摘要
Analysis of the convergence rates of modern convex optimization algorithms can be achived through binary means: analysis of emperical convergence, or analysis of theoretical convergence. These two pathways of capturing information diverge in efficacy when moving to the world of distributed computing, due to the introduction of non-intuitive, non-linear slowdowns associated with broadcasting, and in some cases, gathering operations. Despite these nuances in the rates of convergence, we can still show the existence of convergence, and lower bounds for the rates. This paper will serve as a helpful cheat-sheet for machine learning practitioners encountering this problem class in the field.
更多查看译文
AI 理解论文
溯源树
样例
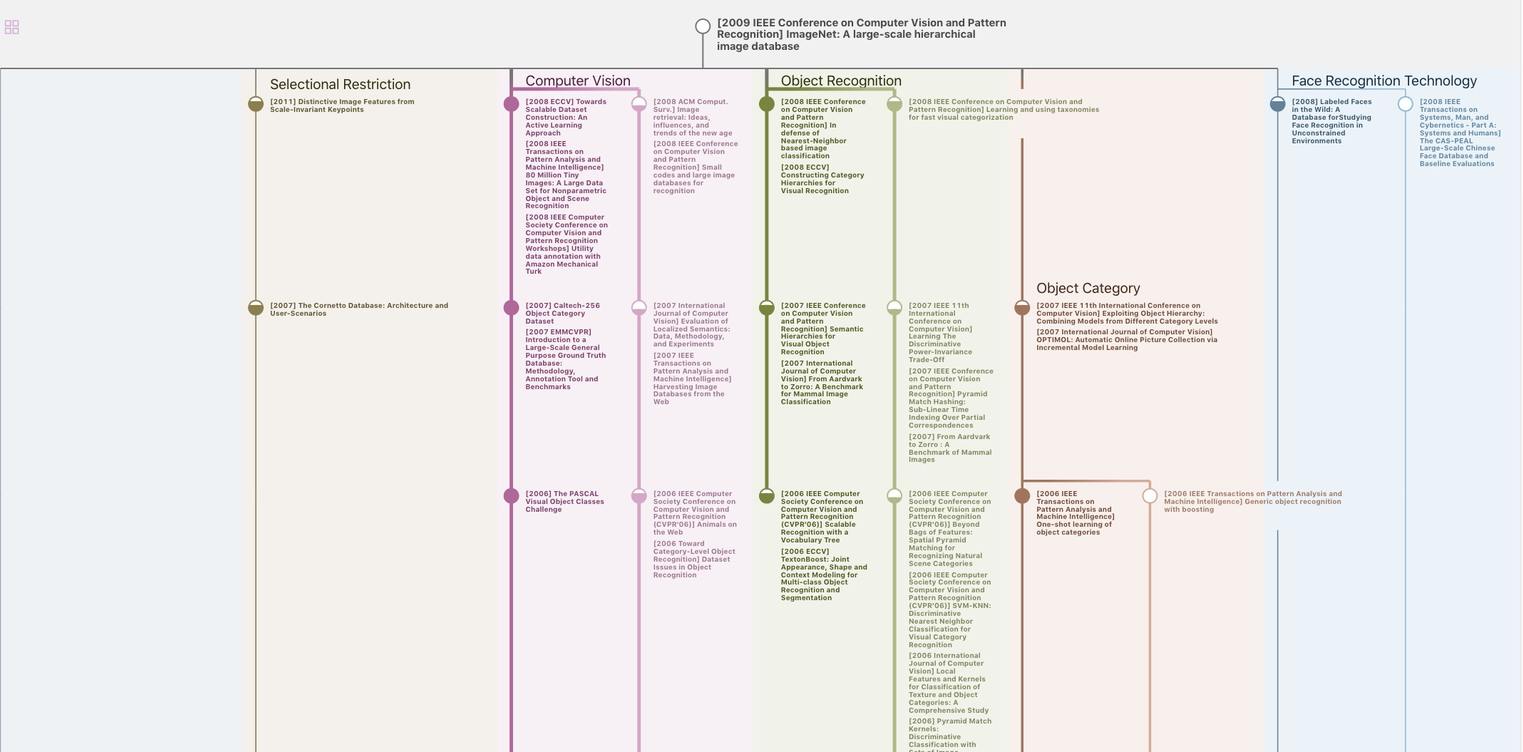
生成溯源树,研究论文发展脉络
Chat Paper
正在生成论文摘要