Exploiting weakly-labeled Web images to improve object classification: a domain adaptation approach.
NIPS'10: Proceedings of the 23rd International Conference on Neural Information Processing Systems - Volume 1(2010)
摘要
Most current image categorization methods require large collections of manually annotated training examples to learn accurate visual recognition models. The time-consuming human labeling effort effectively limits these approaches to recognition problems involving a small number of different object classes. In order to address this shortcoming, in recent years several authors have proposed to learn object classifiers from weakly-labeled Internet images, such as photos retrieved by keyword-based image search engines. While this strategy eliminates the need for human supervision, the recognition accuracies of these methods are considerably lower than those obtained with fully-supervised approaches, because of the noisy nature of the labels associated to Web data. In this paper we investigate and compare methods that learn image classifiers by combining very few manually annotated examples (e.g., 1-10 images per class) and a large number of weakly-labeled Web photos retrieved using keyword-based image search. We cast this as a domain adaptation problem: given a few strongly-labeled examples in a target domain (the manually annotated examples) and many source domain examples (the weakly-labeled Web photos), learn classifiers yielding small generalization error on the target domain. Our experiments demonstrate that, for the same number of strongly-labeled examples, our domain adaptation approach produces significant recognition rate improvements over the best published results (e.g., 65% better when using 5 labeled training examples per class) and that our classifiers are one order of magnitude faster to learn and to evaluate than the best competing method, despite our use of large weakly-labeled data sets.
更多查看译文
关键词
domain adaptation approach,object classification,web images,weakly-labeled
AI 理解论文
溯源树
样例
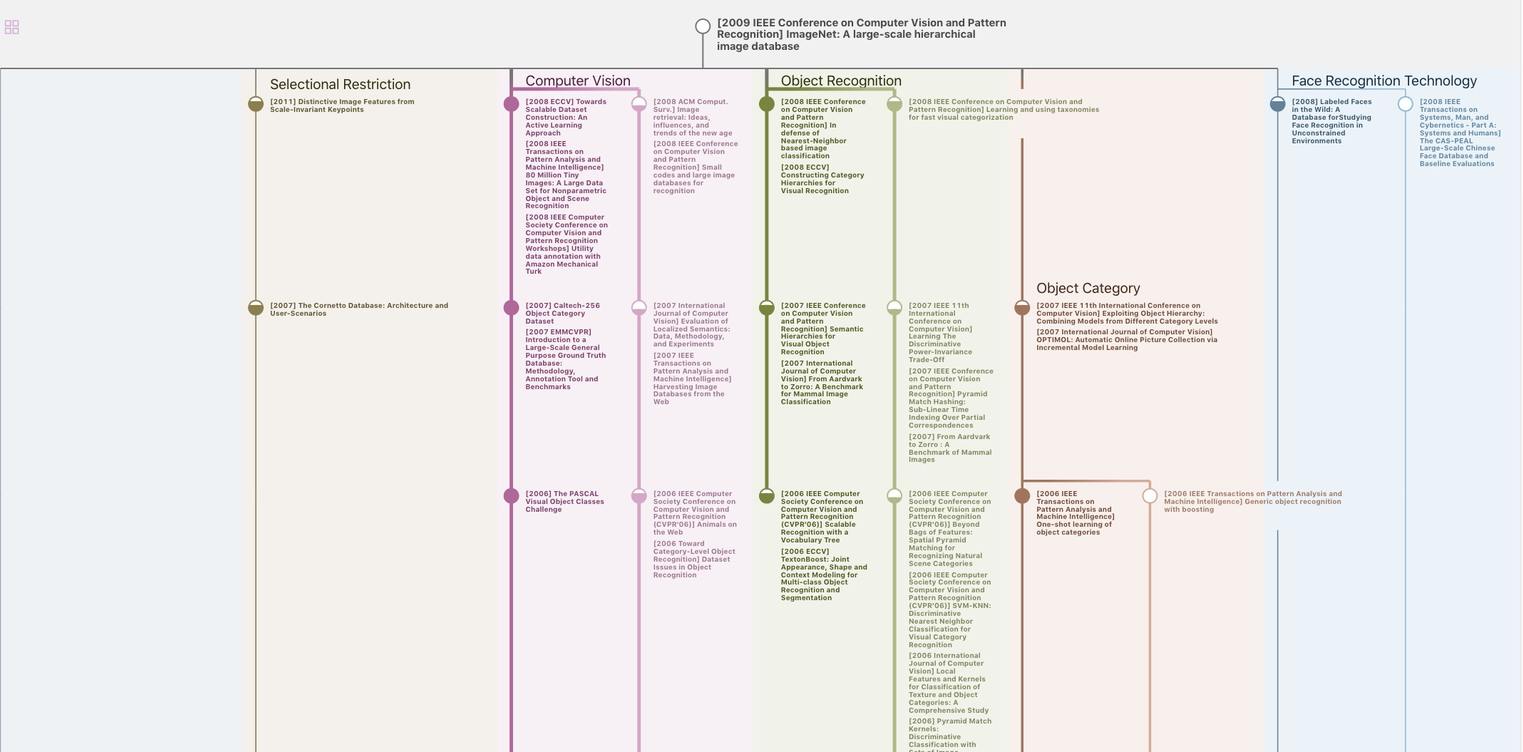
生成溯源树,研究论文发展脉络
Chat Paper
正在生成论文摘要