Aggregation of Crowdsourced Labels Based on Worker History
WIMS(2014)
摘要
Using crowdsourcing for gathering labels can be beneficial for supervised machine learning, if done in the right way. Crowdsourcing is more cost-effective and faster than employing experts for labeling the items needed as training examples. Unfortunately, the crowd produced labels are not always of a comparable quality. Therefore, different methods could be employed in order to assure label quality. One of them is redundancy, by gathering more than one label per item, from different assessors. In this paper we introduce a novel method for aggregating multiple crowdsourced binary labels, taking into account the worker's history and how well the worker agrees with the aggregated label. According to previously solved tasks, the worker expertise, or the confidence we have in his labels can be assessed. The computation of the aggregated crowd label is mutually reinforced by the assessment of the worker confidence. Besides a method for computing a hard nominal aggregated label, we also propose a soft label as an indicator of how much the labelers agree and how strong their labels are. Furthermore, we investigate whether or not worker confidence should depend on the provided label, whether discriminating between positive and negative answer quality can be beneficial. We evaluate our method on multiple datasets, covering different domains and label gathering strategies. Moreover, we compare against other state of the art methods, showing the effectiveness of our proposed approach.
更多查看译文
关键词
crowdsourcing,human computation,learning,quality control
AI 理解论文
溯源树
样例
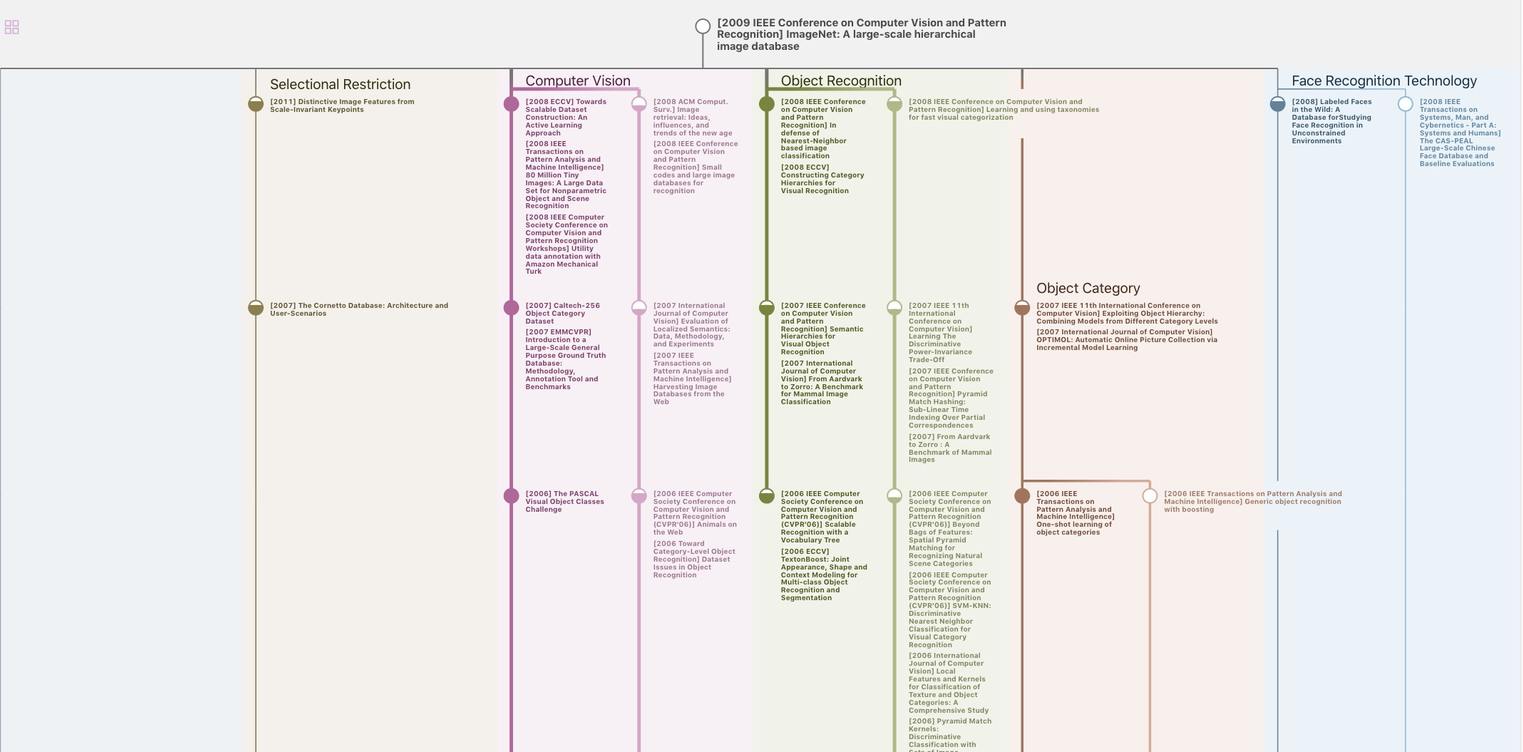
生成溯源树,研究论文发展脉络
Chat Paper
正在生成论文摘要