Maximizing Information Content In Feature Extraction
IEEE TRANSACTIONS ON SPEECH AND AUDIO PROCESSING(2005)
摘要
In this paper, we consider the problem of quantifying the amount of information contained in a set of features, to discriminate between various classes. We explore these ideas in the context of a speech recognition system, where an important classification sub-problem is to predict the phonetic class, given an observed acoustic feature vector. The connection between information content and speech recognition system performance is first explored in the context of various feature extraction schemes used in speech recognition applications. Subsequently, the idea of optimizing the information content to improve recognition accuracy is generalized to a linear projection of the underlying features. We show that several prior methods to compute linear transformations (such as linear/heteroscedastic discriminant analysis) can be interpreted in this general framework of maximizing the information content. Subsequently, we extend this reasoning and propose a new objective function to maximize a penalized mutual information (pMI) measure. This objective function is seen to be very well correlated with the word error rate of the final system. Finally experimental results are provided that show that the proposed pMI projection consistently outperforms other methods for a variety of cases, leading to relative improvements in the word error rate of 5%-16% over earlier methods.
更多查看译文
关键词
classifiers, optimal feature projections, optimum feature extraction, penalized mutual information, speech recognition
AI 理解论文
溯源树
样例
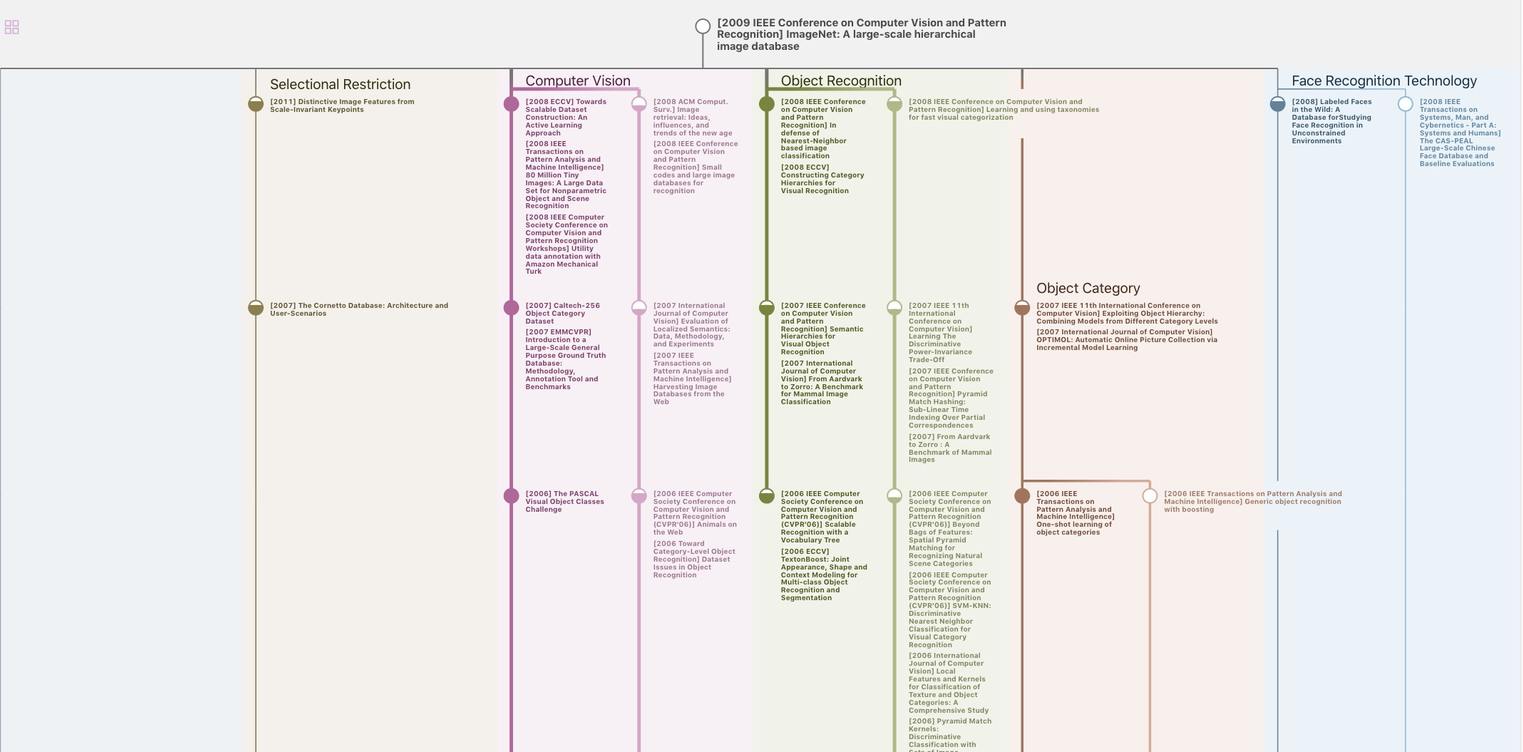
生成溯源树,研究论文发展脉络
Chat Paper
正在生成论文摘要