Improving Researcher Homepage Classification with Unlabeled Data
WWW(2015)
摘要
A classifier that determines if a webpage is relevant to a specified set of topics comprises a key component for focused crawling. Can a classifier that is tuned to perform well on training datasets continue to filter out irrelevant pages in the face of changing content on the Web? We investigate this question in the context of identifying researcher homepages. We show experimentally that classifiers trained on existing datasets of academic homepages underperform on “non-homepages” present on current-day academic websites. As an alternative to obtaining labeled datasets to retrain classifiers for the new content, in this article we ask the following question: “How can we effectively use the unlabeled data readily available from academic websites to improve researcher homepage classification?” We design novel URL-based features and use them in conjunction with content-based features for representing homepages. Within the co-training framework, these sets of features can be treated as complementary views enabling us to effectively use unlabeled data and obtain remarkable improvements in homepage identification on the current-day academic websites. We also propose a novel technique for “learning a conforming pair of classifiers” that mimics co-training. Our algorithm seeks to minimize a loss (objective) function quantifying the difference in predictions from the two views afforded by co-training. We argue that this loss formulation provides insights for understanding co-training and can be used even in the absence of a validation dataset. Our next set of findings pertains to the evaluation of other state-of-the-art techniques for classifying homepages. First, we apply feature selection (FS) and feature hashing (FH) techniques independently and in conjunction with co-training to academic homepages. FS is a well-known technique for removing redundant and unnecessary features from the data representation, whereas FH is a technique that uses hash functions for efficient encoding of features. We show that FS can be effectively combined with co-training to obtain further improvements in identifying homepages. However, using hashed feature representations, a performance degradation is observed possibly due to feature collisions. Finally, we evaluate other semisupervised algorithms for homepage classification. We show that although several algorithms are effective in using information from the unlabeled instances, co-training that explicitly harnesses the feature split in the underlying instances outperforms approaches that combine content and URL features into a single view.
更多查看译文
关键词
Algorithms,Researcher homepage classification,co-training,conforming classifiers,unlabeled data
AI 理解论文
溯源树
样例
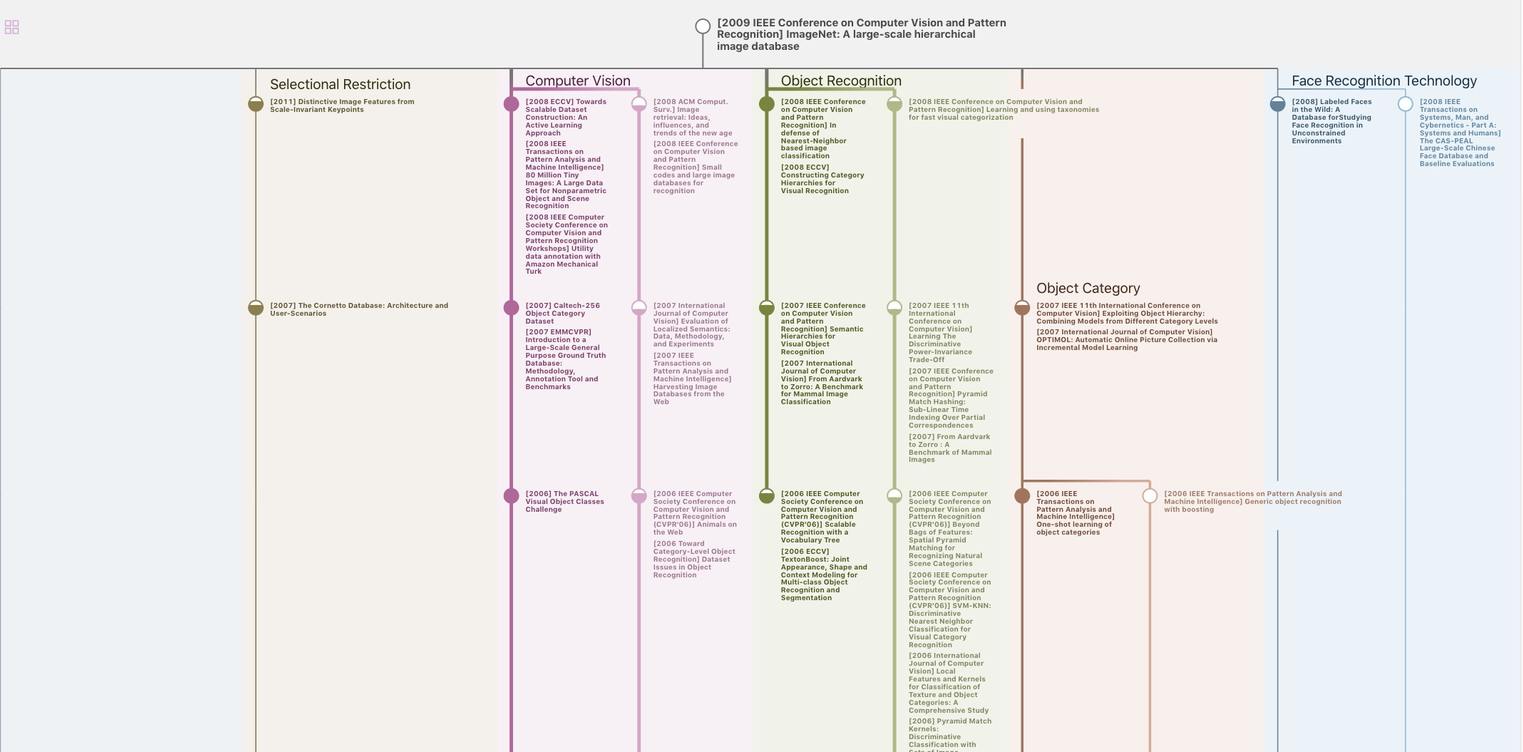
生成溯源树,研究论文发展脉络
Chat Paper
正在生成论文摘要