Parametric Bandits: The Generalized Linear Case (extended version)
Neural Information Processing Systems(2010)
摘要
We consider structured multi-armed bandit problems based on the Generalized Linear Model (GLM) framework of statistics.
For these bandits, we propose a new algorithm, called GLM-UCB.
We derive finite time, high probability bounds on the regret of the algorithm, extending previous analyses developed for the linear bandits to the non-linear case.
The analysis highlights a key difficulty in generalizing linear bandit algorithms to the non-linear case, which is
solved in GLM-UCB by focusing on the reward space rather than on the parameter space.
Moreover,
as the actual effectiveness of current parameterized bandit algorithms is often poor
in practice, we provide a tuning method based on asymptotic arguments,
which leads to significantly better practical performance.
We present two numerical experiments on real-world data that
illustrate the potential of the GLM-UCB approach.
更多查看译文
关键词
multi armed bandit problem,general linear model,parameter space
AI 理解论文
溯源树
样例
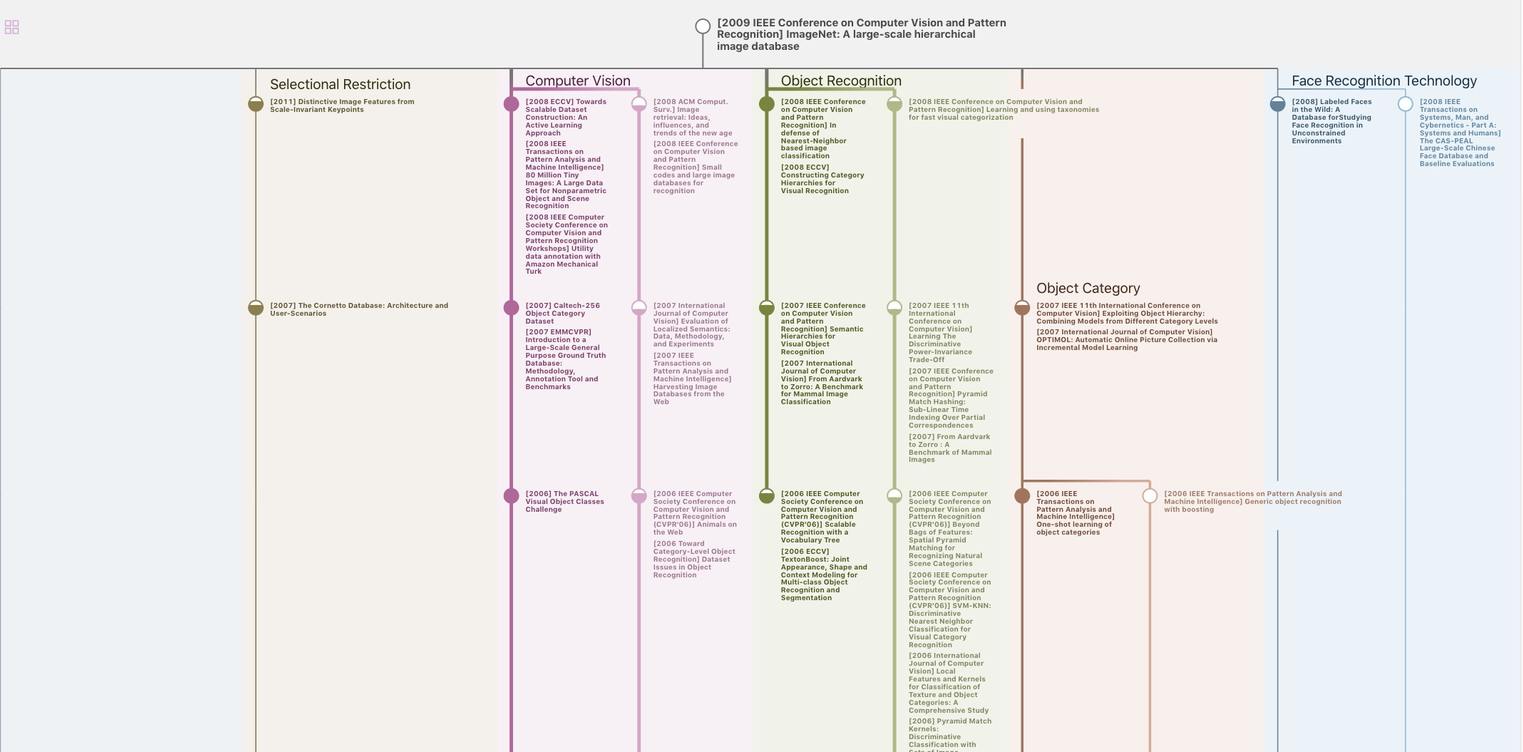
生成溯源树,研究论文发展脉络
Chat Paper
正在生成论文摘要