Texture Optimization For Example-Based Synthesis
ACM Transactions on Graphics(2005)
摘要
We present a novel technique for texture synthesis using optimization. We define a Markov Random Field (MRF)-based similarity metric for measuring the quality of synthesized texture with respect to a given input sample. This allows us to formulate the synthesis problem as minimization of an energy function, which is optimized using an Expectation Maximization (EM)-like algorithm. In contrast to most example-based techniques that do region-growing, ours is a joint optimization approach that progressively refines the entire texture. Additionally, our approach is ideally suited to allow for controllable synthesis of textures. Specifically, we demonstrate controllability by animating image textures using flow fields. We allow for general two-dimensional flow fields that may dynamically change over time. Applications of this technique include dynamic texturing of fluid animations and texture-based flow visualization.
更多查看译文
关键词
texture synthesis,energy minimization,flow visualization,texture animation,image-based rendering
AI 理解论文
溯源树
样例
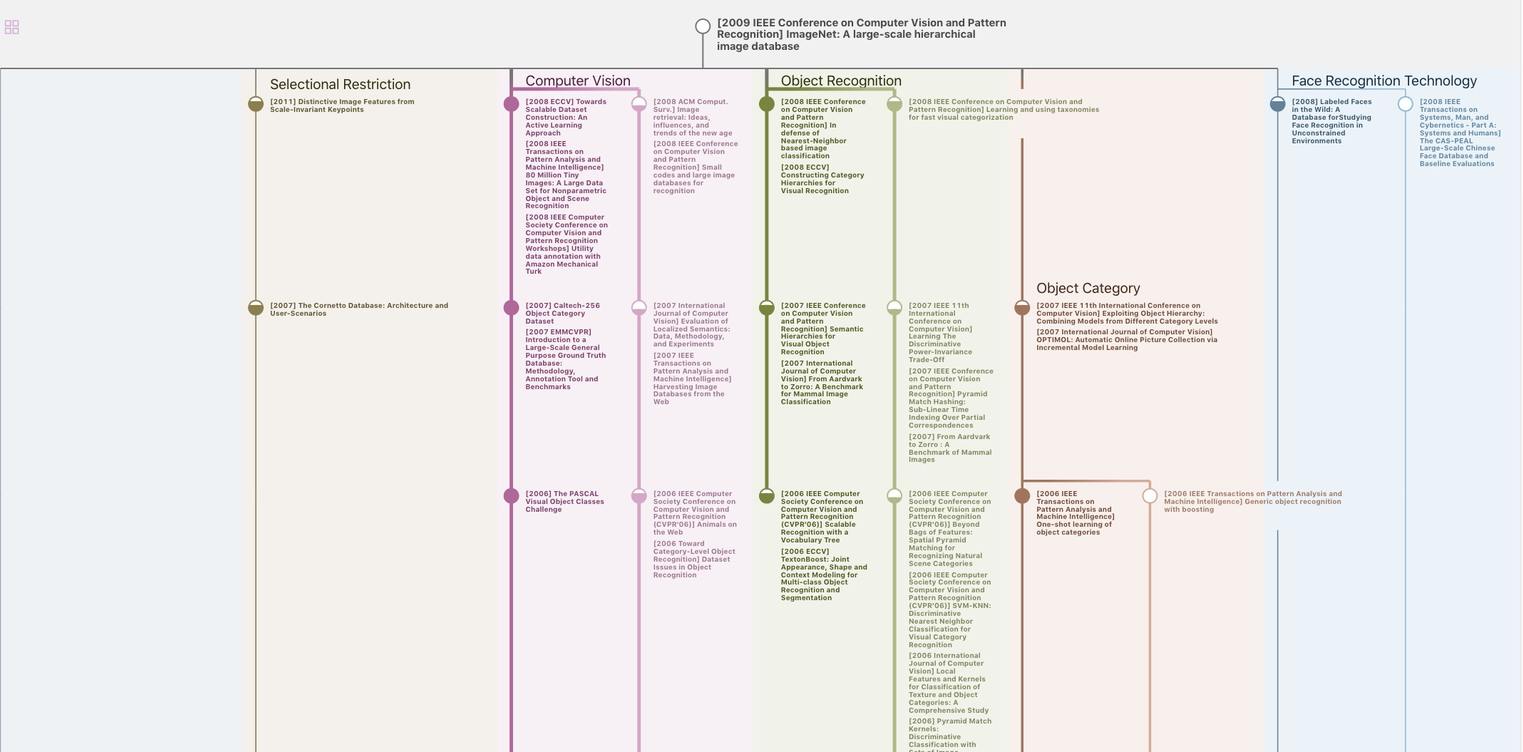
生成溯源树,研究论文发展脉络
Chat Paper
正在生成论文摘要