TH-D-332-03: Optimization of Landmark Detection for Auto-Contouring in Radiation Treatment Planning for Head and Neck Cancer
MEDICAL PHYSICS(2008)
摘要
Purpose: To improve the performance of the automatic detection of landmarks, used for 3‐D deformable model initialization, for automatic contouring of the head and neck region in radiation treatment planning. Method and Materials: An automatic landmark detection method has previously been reported by our group. The method uses principal component analysis (PCA) of landmark distributions and 10 nonlinearly registered patient datasets to generate a patient atlas. A controlled random search algorithm (CRS) seeks the optimal transformation between the atlas and the patient dataset to be contoured. The optimal transformation parameters are then used to propagate the atlas landmarks to the patient dataset. In this work, we propose an improved landmark detection method by optimizing the impact of various parameters on the performance of our method and present the results. The parameters involved include varying the number of eigenmodes, increasing the number of landmarks used for nonlinear registration of the atlas datasets, and reducing the constraints imposed by principal component analysis (PCA), by allowing a locally unconstrained search for each landmark. Results: The method has been validated using 10 atlases, each tested on 10 datasets not included in the atlas. Optimization of our automatic landmark identification parameters for the 7 landmarks identified as having the poorest performance, led to a mean rms‐distance to ground truth of 11.2 ± 1.5 mm (range: 7.7 – 18.2 mm), an improvement of 5.9 mm (30%) compared with previously reported results. Conclusion: We optimized the impact of 14 parameters on the performance of automatic landmark identification. As a result, we have reduced the mean rms‐distance to ground truth by 5.9 mm. Improved model initialization through optimized landmark detection, will result in more robust model adaptation and thereby improved automated contouring of the organs at risk. Conflict of Interest: This work is supported in part by Philips Healthcare.
更多查看译文
关键词
treatment planning
AI 理解论文
溯源树
样例
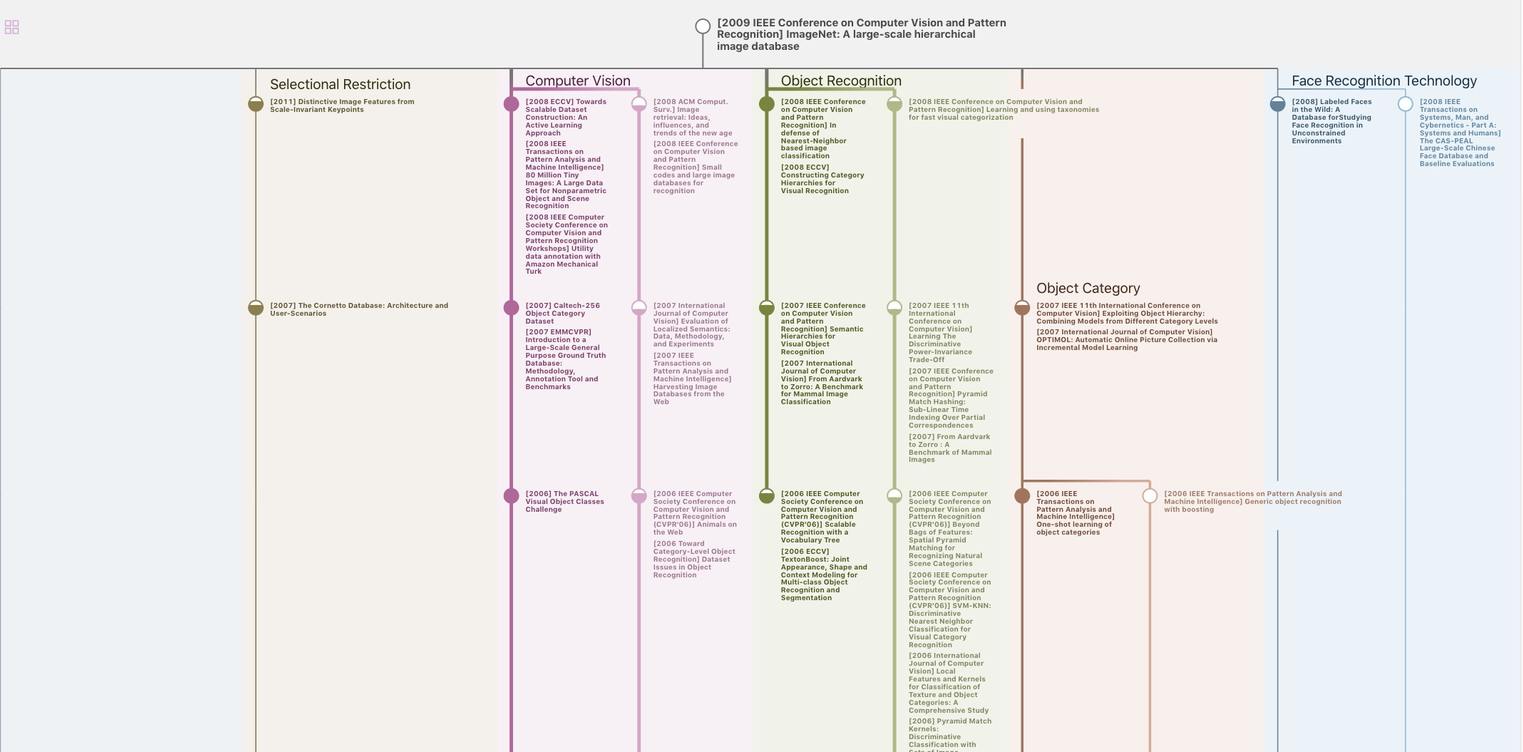
生成溯源树,研究论文发展脉络
Chat Paper
正在生成论文摘要