Diagnosis code assignment: models and evaluation metrics.
JOURNAL OF THE AMERICAN MEDICAL INFORMATICS ASSOCIATION(2014)
摘要
Background and objective The volume of healthcare data is growing rapidly with the adoption of health information technology. We focus on automated ICD9 code assignment from discharge summary content and methods for evaluating such assignments. Methods We study ICD9 diagnosis codes and discharge summaries from the publicly available Multiparameter Intelligent Monitoring in Intensive Care II (MIMIC II) repository. We experiment with two coding approaches: one that treats each ICD9 code independently of each other (flat classifier), and one that leverages the hierarchical nature of ICD9 codes into its modeling (hierarchy-based classifier). We propose novel evaluation metrics, which reflect the distances among gold-standard and predicted codes and their locations in the ICD9 tree. Experimental setup, code for modeling, and evaluation scripts are made available to the research community. Results The hierarchy-based classifier outperforms the flat classifier with F-measures of 39.5% and 27.6%, respectively, when trained on 20533 documents and tested on 2282 documents. While recall is improved at the expense of precision, our novel evaluation metrics show a more refined assessment: for instance, the hierarchy-based classifier identifies the correct sub-tree of gold-standard codes more often than the flat classifier. Error analysis reveals that gold-standard codes are not perfect, and as such the recall and precision are likely underestimated. Conclusions Hierarchy-based classification yields better ICD9 coding than flat classification for MIMIC patients. Automated ICD9 coding is an example of a task for which data and tools can be shared and for which the research community can work together to build on shared models and advance the state of the art.
更多查看译文
关键词
Machine Learning,Electronic Health Records,Clinical Coding,ICD Codes,Medical Informatics
AI 理解论文
溯源树
样例
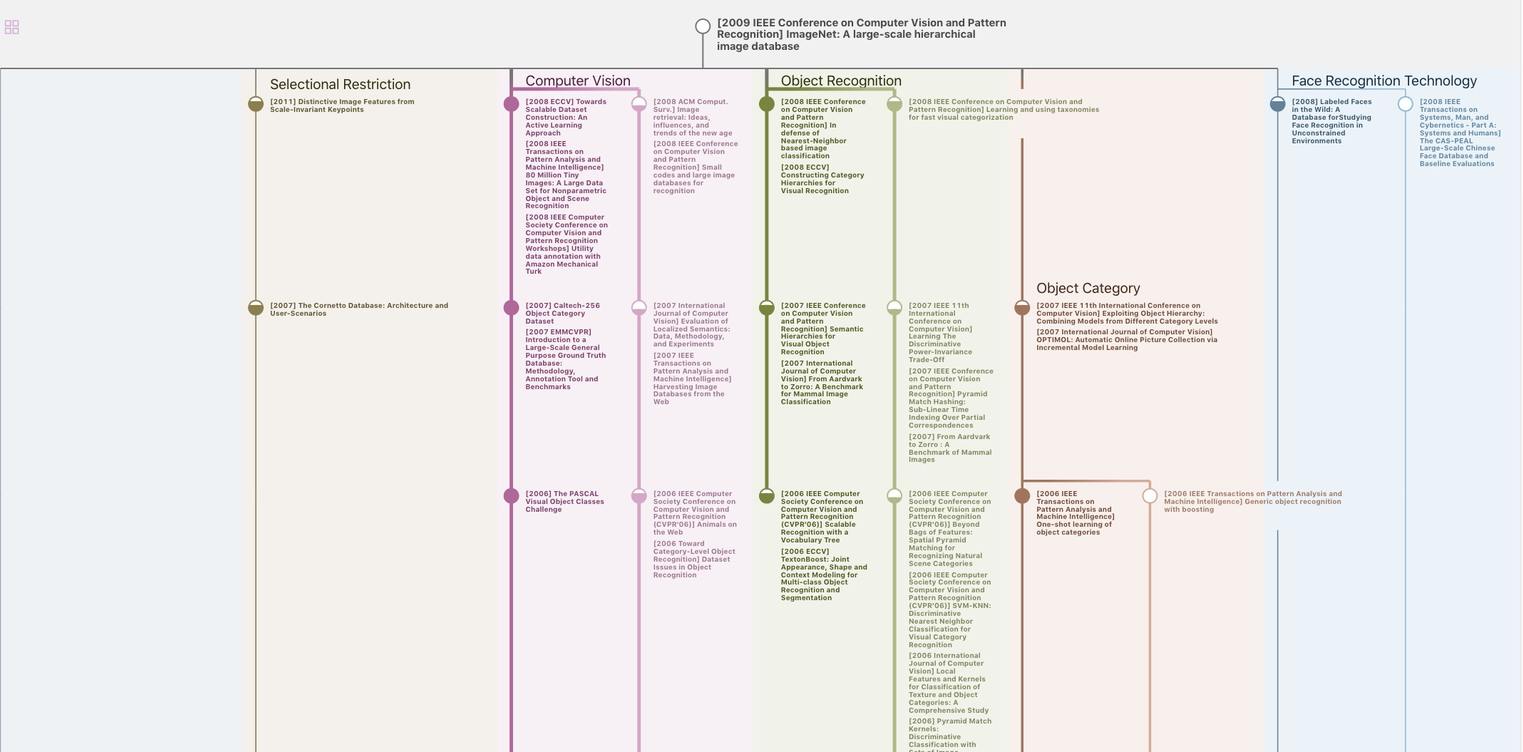
生成溯源树,研究论文发展脉络
Chat Paper
正在生成论文摘要