Development and validation of a novel blending machine learning model for hospital mortality prediction in ICU patients with Sepsis
BIODATA MINING(2021)
摘要
Background Early prediction of hospital mortality is crucial for ICU patients with sepsis. This study aimed to develop a novel blending machine learning (ML) model for hospital mortality prediction in ICU patients with sepsis. Methods Two ICU databases were employed: eICU Collaborative Research Database (eICU-CRD) and Medical Information Mart for Intensive Care III (MIMIC-III). All adult patients who fulfilled Sepsis-3 criteria were identified. Samples from eICU-CRD constituted training set and samples from MIMIC-III constituted test set. Stepwise logistic regression model was used for predictor selection. Blending ML model which integrated nine sorts of basic ML models was developed for hospital mortality prediction in ICU patients with sepsis. Model performance was evaluated by various measures related to discrimination or calibration. Results Twelve thousand five hundred fifty-eight patients from eICU-CRD were included as the training set, and 12,095 patients from MIMIC-III were included as the test set. Both the training set and the test set showed a hospital mortality of 17.9%. Maximum and minimum lactate, maximum and minimum albumin, minimum PaO2/FiO2 and age were important predictors identified by both random forest and extreme gradient boosting algorithm. Blending ML models based on corresponding set of predictors presented better discrimination than SAPS II (AUROC, 0.806 vs. 0.771; AUPRC 0.515 vs. 0.429) and SOFA (AUROC, 0.742 vs. 0.706; AUPRC 0.428 vs. 0.381) on the test set. In addition, calibration curves showed that blending ML models had better calibration than SAPS II. Conclusions The blending ML model is capable of integrating different sorts of basic ML models efficiently, and outperforms conventional severity scores in predicting hospital mortality among septic patients in ICU.
更多查看译文
关键词
Sepsis, Intensive care unit, Hospital mortality prediction, Machine learning, MIMIC-III, eICU-CRD
AI 理解论文
溯源树
样例
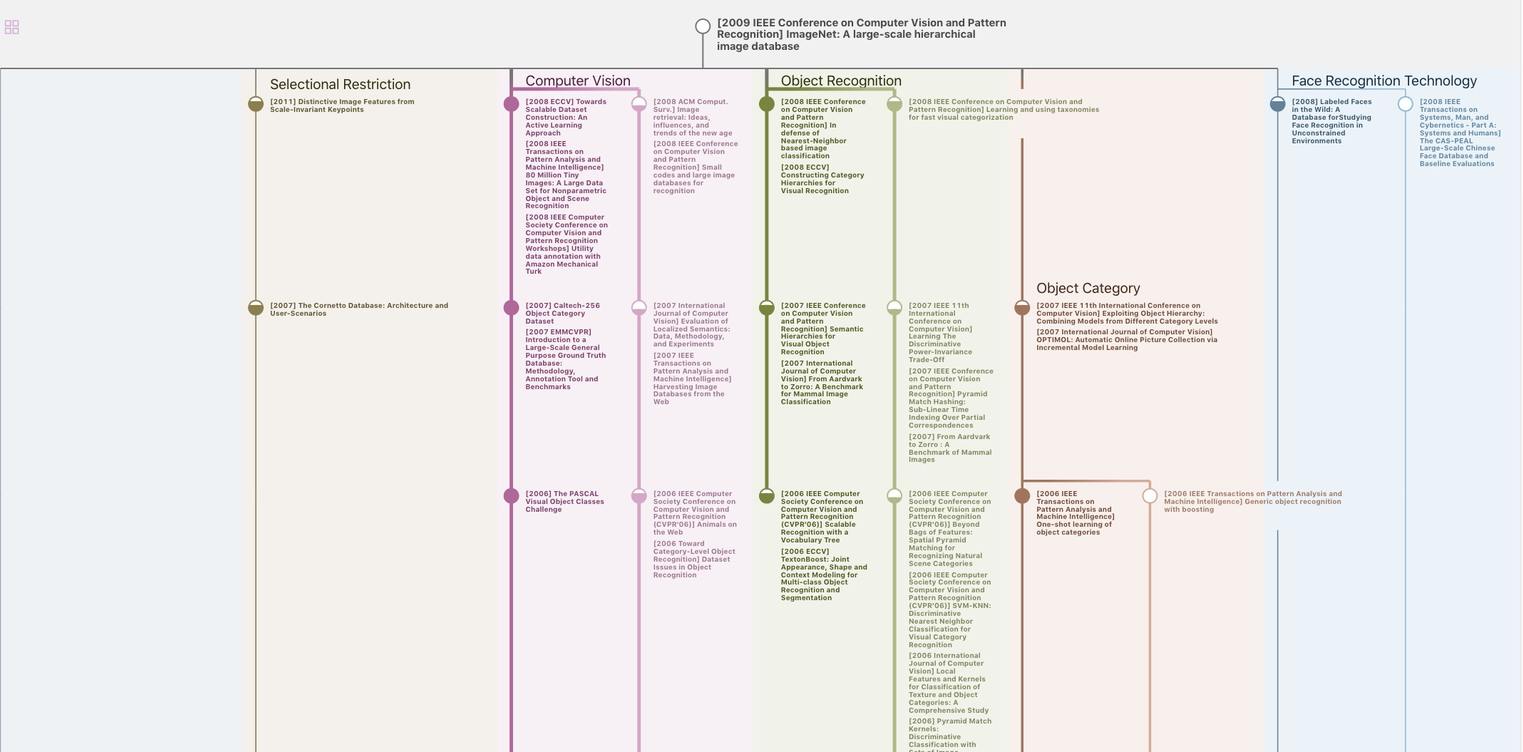
生成溯源树,研究论文发展脉络
Chat Paper
正在生成论文摘要