Discovering Novel Attack Strategies From Infosec Alerts
ESORICS(2004)
摘要
Correlating security alerts and discovering attack strategies are important and challenging tasks for security analysts. Recently, there have been several proposed techniques to analyze attack scenarios from security alerts. However, most of these approaches depend on a priori and hard-coded domain knowledge that lead to their limited capabilities of detecting new attack strategies. In this paper, we propose an approach to discover novel attack strategies. Our approach includes two complementary correlation mechanisms based on two hypotheses of attack step relationship. The first hypothesis is that attack steps are directly related because an earlier attack enables or positively affects the later one. For this type of attack relationship, we develop a Bayesian-based correlation engine to correlate attack steps based on security states of systems and networks. The second hypothesis is that for some related attack steps, even though they do not have obvious and direct relationship in terms of security and performance measures, they still have temporal and statistical patterns. For this category of relationship, we apply time series and statistical analysis to correlate attack steps. The security analysts are presented with aggregated information on attack strategies from these two correlation engines. We evaluate our approach using DARPA's Grand Challenge Problem (GCP) data sets. The results show that our approach can discover novel attack strategies and provide a quantitative analysis of attack scenarios.
更多查看译文
关键词
positive affect,statistical analysis,time series,quantitative analysis,domain knowledge
AI 理解论文
溯源树
样例
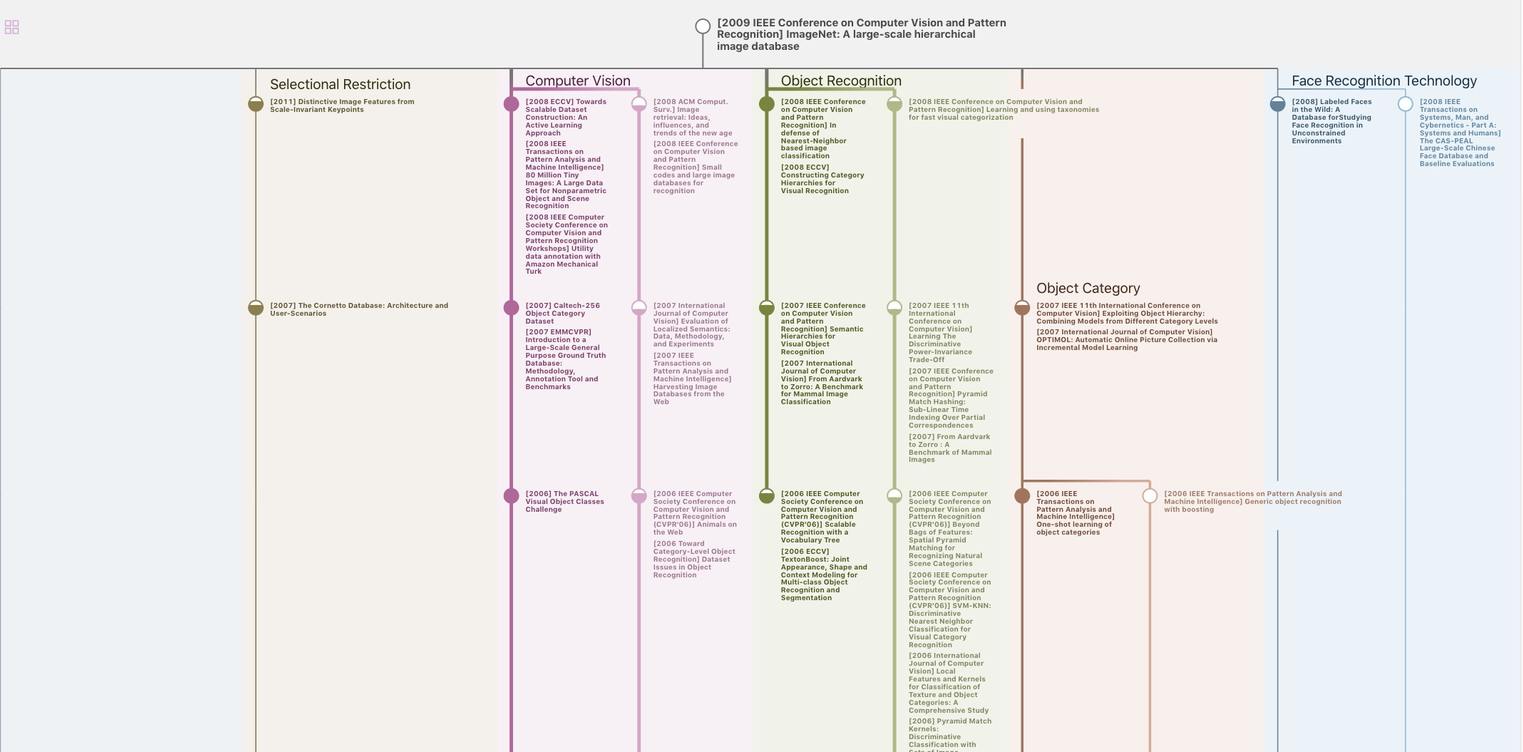
生成溯源树,研究论文发展脉络
Chat Paper
正在生成论文摘要