Problem classification method to enhance the ITIL incident and problem
Integrated Network Management(2009)
摘要
Problem determination and resolution PDR is the process of detecting anomalies in a monitored system, locating the problems responsible for the issue, determining the root cause and fixing the cause of the problem. The cost of PDR represents a substantial part of operational costs, and faster, more effective PDR can contribute to a substantial reduction in system administration costs. In this paper, we propose to automate the process of PDR by leveraging machine learning methods. The main focus is to effectively categorize the problem a user experiences by recognizing the problem specificity leveraging all available training data such like the performance data and the logs data. Specifically, we transform the structure of the problem into a hierarchy which can be determined by existing taxonomy in advance. We then propose an efficient hierarchical incremental learning algorithm which is capable of adjusting its internal local classifier parameters in real-time. Comparing to the traditional batch learning algorithms, this online learning framework can significantly decrease the computational complexity of the training process by learning from new instances on an incremental fashion. In the same time this reduces the amount of memory required to store the training instances. We demonstrate the efficiency of our approach by learning hierarchical problem patterns for several issues occurring in distributed web applications. Experimental results show that our approach substantially outperforms previous methods.
更多查看译文
关键词
problem determination,training instance,resolution pdr,performance data,problem specificity,available training data,logs data,hierarchical problem pattern,itil incident,problem classification method,effective pdr,efficient hierarchical incremental learning,learning artificial intelligence,prediction algorithms,decision trees,classification algorithms,system monitoring,computational complexity,real time,machine learning,computer science,databases,training data,user experience,taxonomy,system performance,vectors,distributed processing
AI 理解论文
溯源树
样例
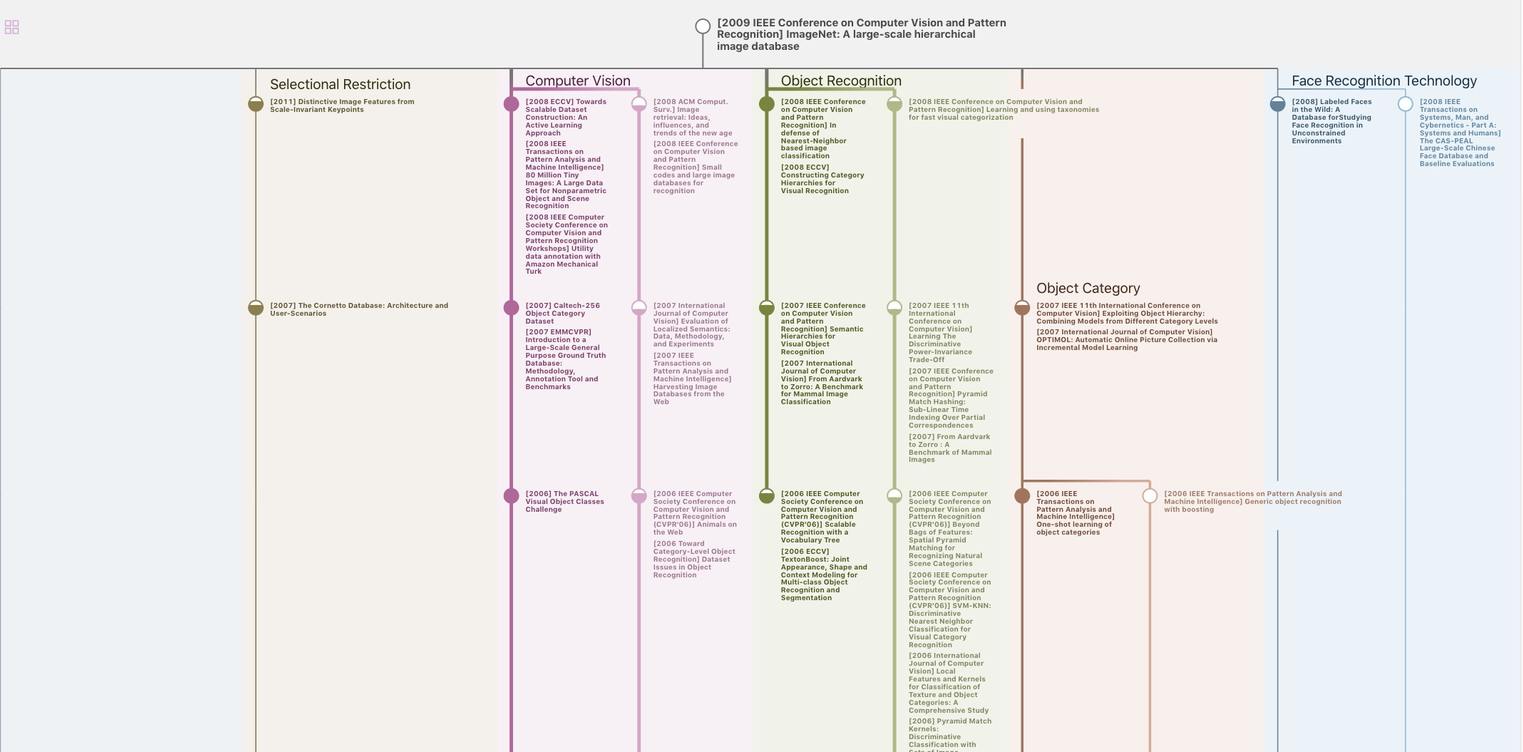
生成溯源树,研究论文发展脉络
Chat Paper
正在生成论文摘要