The Grouped Author-Topic Model For Unsupervised Entity Resolution
ICANN'11: Proceedings of the 21th international conference on Artificial neural networks - Volume Part I(2011)
摘要
This paper describes a generative approach for tackling the problem of identity resolution in a completely unsupervised context with no fixed assumption regarding the true number of identities. The problem of entity resolution involves associating different references to authors (in a paper's author list, for example) with real underlying identities. The references may be written in differing forms or may have errors, and identical references may refer to different real identities. The approach taken here uses a generative model of both the abstract of a document and its list of authors to resolve identities in a corpus of documents. In the model, authors and topics are associated with latent groups. For each document, an abstract and an author list are generated conditioned on a given group. Results are presented on real-world datasets, and outperform the best performing unsupervised methods.
更多查看译文
关键词
Bayesian nonparametrics,Dirichlet processes,nested Dirichlet processes,author disambiguation
AI 理解论文
溯源树
样例
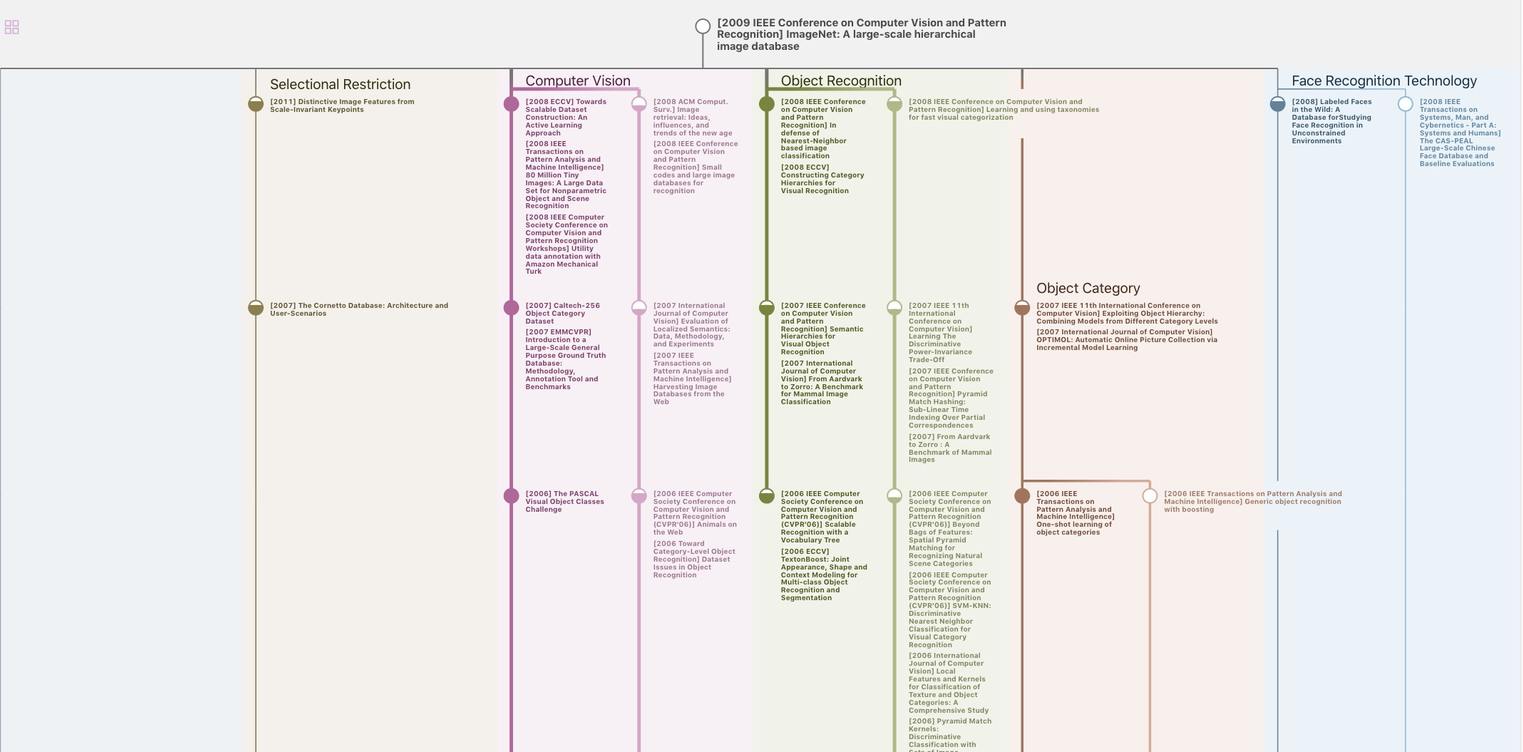
生成溯源树,研究论文发展脉络
Chat Paper
正在生成论文摘要