A Hierarchy Of Independence Assumptions For Multi-Relational Bayes Net Classifiers
2013 IEEE SYMPOSIUM ON COMPUTATIONAL INTELLIGENCE AND DATA MINING (CIDM)(2013)
摘要
Many databases store data in relational format, with different types of entities and information about their attributes and links between the entities. Link-based classification (LBC) is the problem of predicting the class attribute of a target entity given the attributes of entities linked to it. In this paper we propose a new relational Bayes net classifier method for LBC, which assumes that different links of an object are independently drawn from the same distribution, given attribute information from the linked tables. We show that this assumption allows very fast multi-relational Bayes net learning. We define three more independence assumptions for LBC to unify proposals from different researchers in a single novel hierarchy. Our proposed model is at the top and the well-known multi-relational Naive Bayes classifier is at the bottom of this hierarchy. The model in each level of the hierarchy uses a new independence assumption in addition to the assumptions used in the higher levels. In experiments on four benchmark datasets, our proposed link independence model has the best predictive accuracy compared to the hierarchy models and a variety of relational classifiers.
更多查看译文
关键词
Link-based Classification,Bayes net classifier,Statistical-relational learning,Knowledge Discovery in Databases
AI 理解论文
溯源树
样例
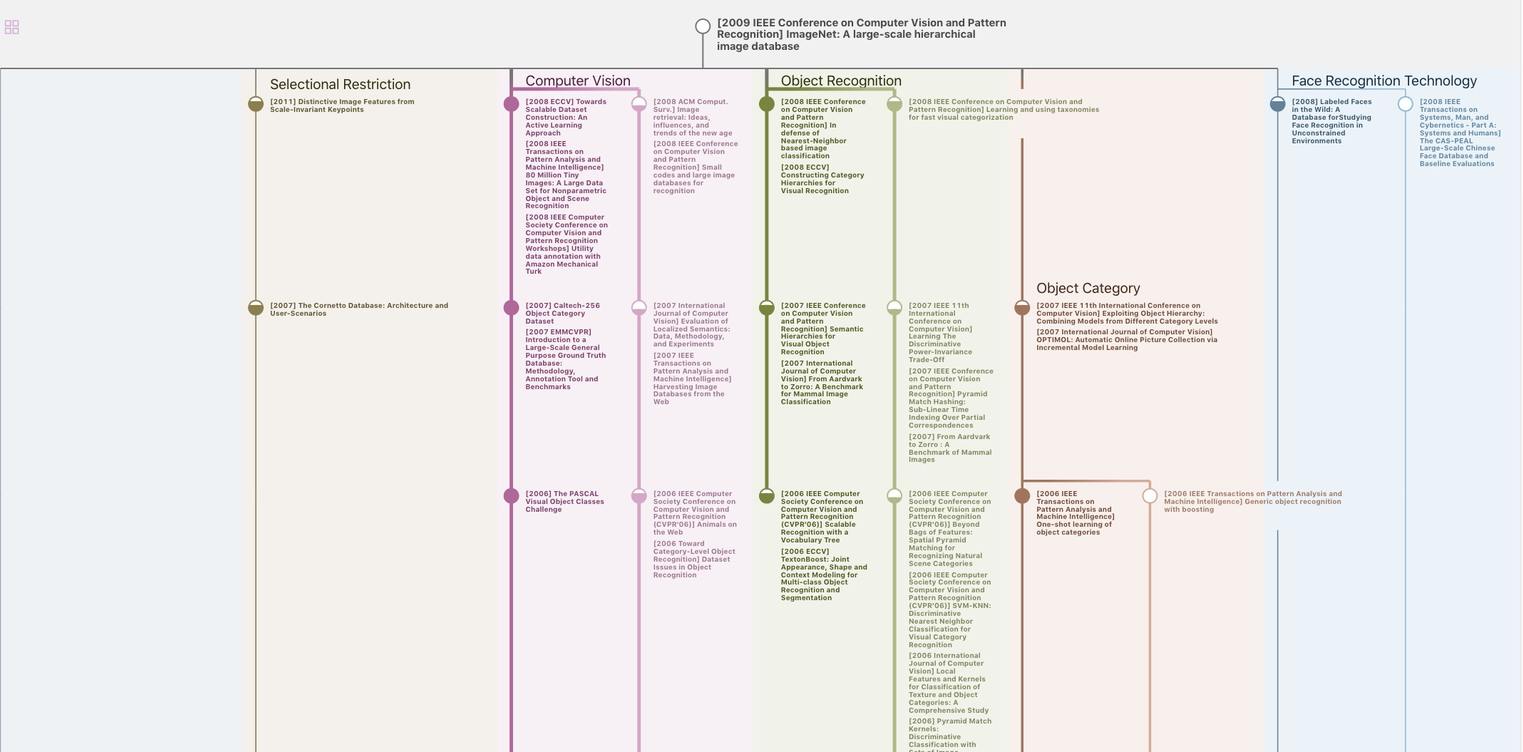
生成溯源树,研究论文发展脉络
Chat Paper
正在生成论文摘要