Kernels On Prolog Proof Trees: Statistical Learning In The Ilp Setting
The Journal of Machine Learning Research(2006)
摘要
We develop kernels for measuring the similarity between relational instances using background knowledge expressed in first-order logic. The method allows us to bridge the gap between traditional inductive logic programming (ILP) representations and statistical approaches to supervised learning. Logic programs are first used to generate proofs of given visitor programs that use predicates declared in the available background knowledge. A kernel is then defined over pairs of proof trees. The method can be used for supervised learning tasks and is suitable for classification as well as regression. We report positive empirical results on Bongard-like and M-of-N problems that are difficult or impossible to solve with traditional ILP techniques, as well as on real bioinformatics and chemoinformatics data sets.
更多查看译文
关键词
kernel methods,inductive logic programming,Prolog,learning from program traces
AI 理解论文
溯源树
样例
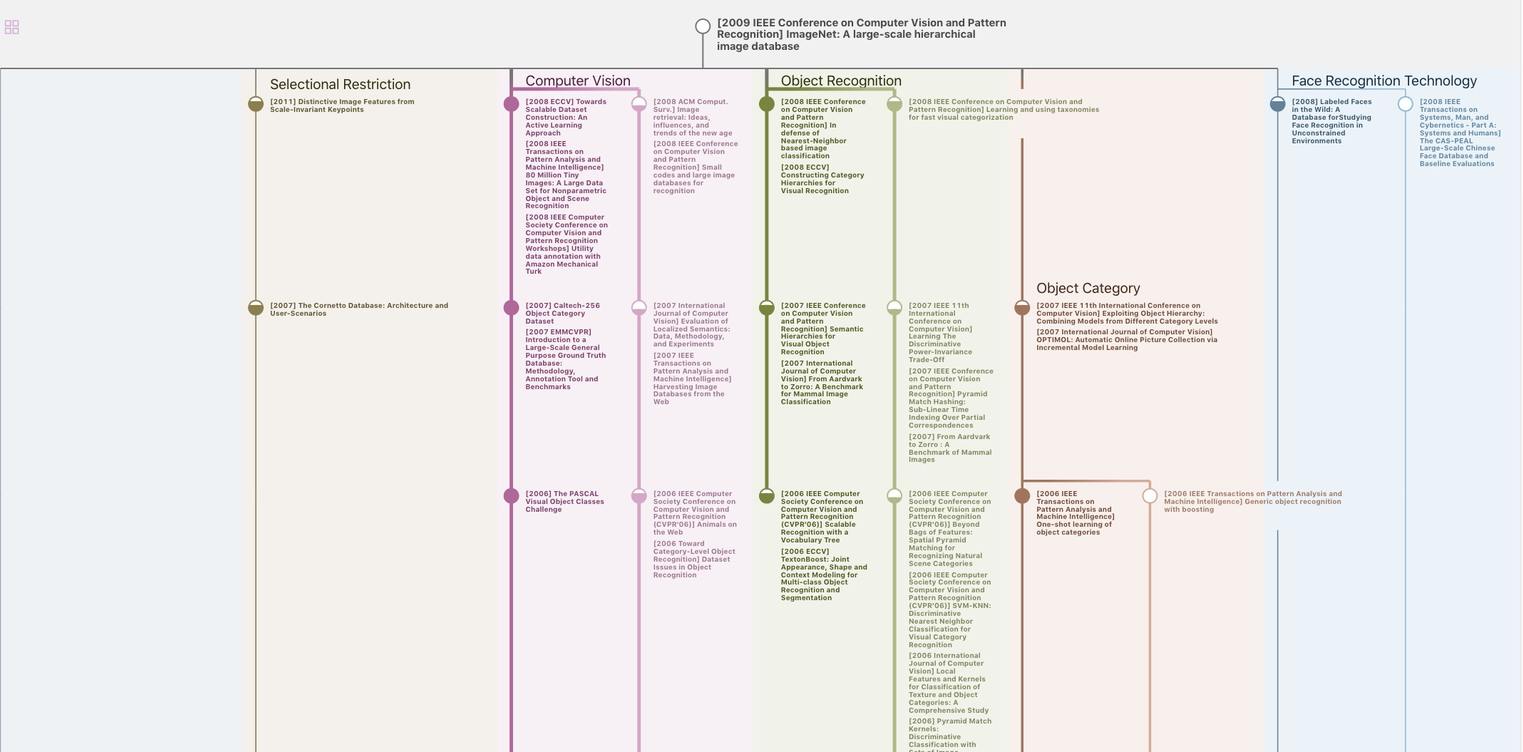
生成溯源树,研究论文发展脉络
Chat Paper
正在生成论文摘要