Letters: Learning non-linear time-scales with kernel γ-filters
Neurocomputing(2009)
摘要
A family of kernel methods, based on the @c-filter structure, is presented for non-linear system identification and time series prediction. The kernel trick allows us to develop the natural non-linear extension of the (linear) support vector machine (SVM) @c-filter [G. Camps-Valls, M. Martinez-Ramon, J.L. Rojo-Alvarez, E. Soria-Olivas, Robust @c-filter using support vector machines, Neurocomput. J. 62(12) (2004) 493-499.], but this approach yields a rigid system model without non-linear cross relation between time-scales. Several functional analysis properties allow us to develop a full, principled family of kernel @c-filters. The improved performance in several application examples suggests that a more appropriate representation of signal states is achieved.
更多查看译文
关键词
e. soria-olivas,principled family,support vector machine,non-linear cross relation,c-filter structure,natural non-linear extension,non-linear time-scales,kernel method,rigid system model,non-linear system identification,kernel trick,kernel
AI 理解论文
溯源树
样例
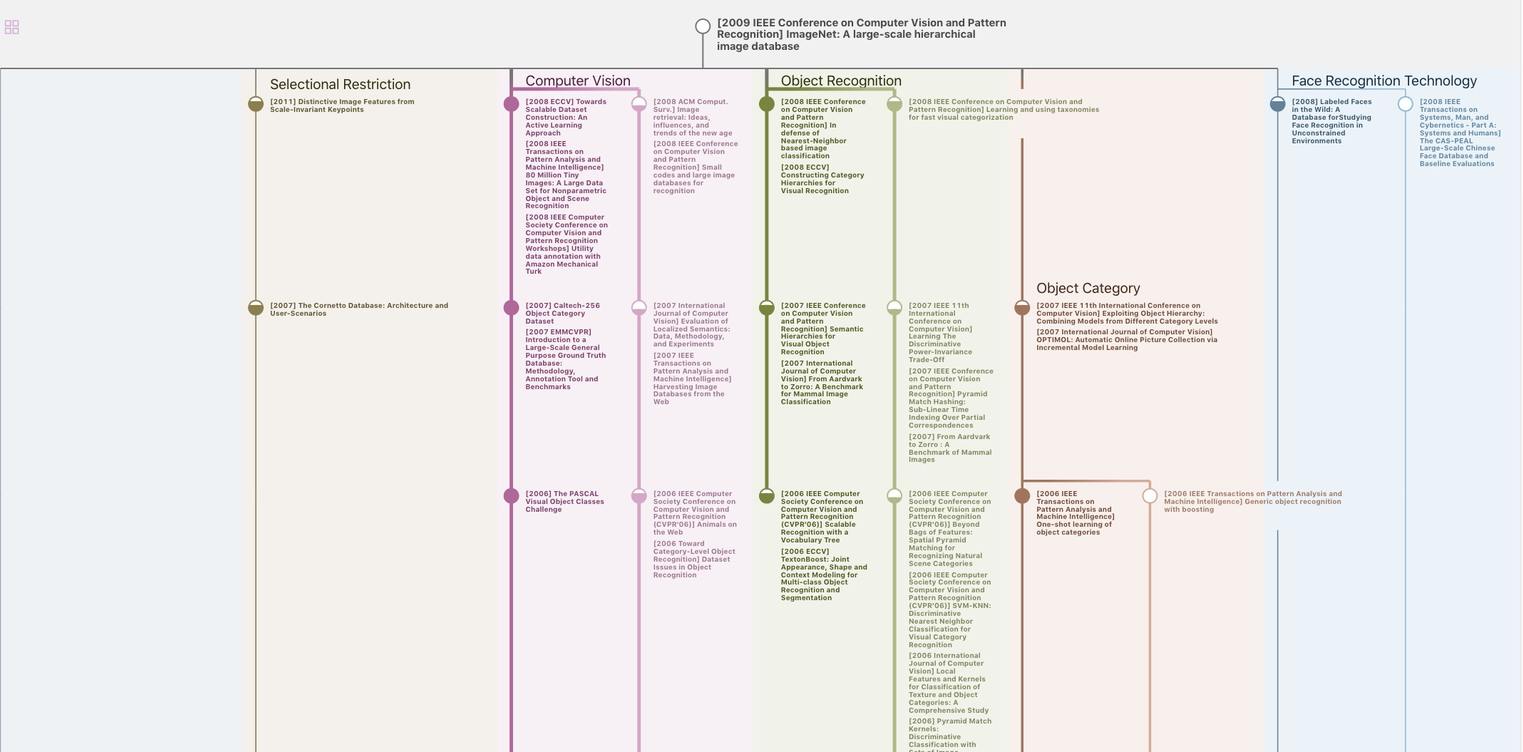
生成溯源树,研究论文发展脉络
Chat Paper
正在生成论文摘要