Retrieval and Discovery of Cell Cycle Literature and Proteins by Means of Machine Learning, Text Mining and Network Analysis.
8TH INTERNATIONAL CONFERENCE ON PRACTICAL APPLICATIONS OF COMPUTATIONAL BIOLOGY & BIOINFORMATICS (PACBB 2014)(2014)
摘要
The cell cycle is one of the most important biological processes, being studied intensely by experimental as well as bioinformatics means. A considerable amount of literature provides relevant descriptions of proteins involved in this complex process. These proteins are often key to understand cellular alterations encountered in pathological conditions such as abnormal cell growth. The authors explored the use of text mining strategies to improve the retrieval of relevant articles and individual sentences for this topic. Moreover information extraction and text mining was used to detect and rank automatically Arabidopsis proteins important for the cell cycle. The obtained results were evaluated using independent data collections and compared to keyword-based strategies. The obtained results indicate that the use of machine learning methods can improve the sensitivity compared to term-co-occurrence, although with considerable differences when using abstracts and full text articles as input. At the level of document triage the recall ranges for abstracts from around 16% for keyword indexing, 37% for a sentence SVM classifier to 57% for SVM abstract classifier. In case of full text data, keyword and cell cycle phrase indexing obtained a recall of 42% and 55% respectively compared to 94% reached by a sentence classifier. In case of the cell cycle protein detection, the cell cycle keyword-protein co-occurrence strategy had a recall of 52% for abstracts and 70% for full text while a protein mentioning sentence classifier obtained a recall of over 83% for abstracts and 79% for full text. The generated cell cycle term co-occurrence statistics and SVM confidence scores for each protein were explored to rank proteins and filter a protein network in order to derive a topic specific subnetwork.
更多查看译文
关键词
text mining,natural language processing,cell cycle,machine learning,protein ranking
AI 理解论文
溯源树
样例
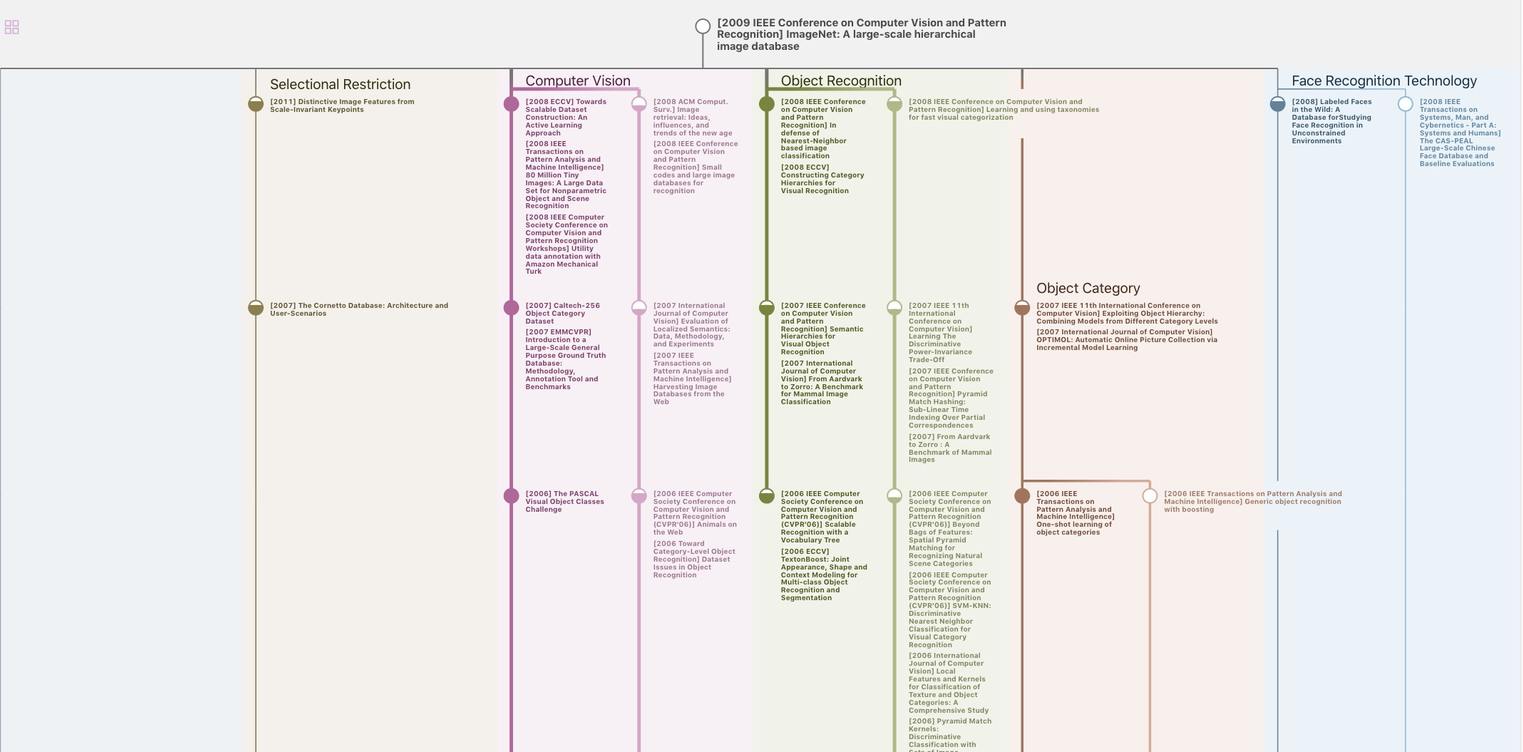
生成溯源树,研究论文发展脉络
Chat Paper
正在生成论文摘要