Generalized Expectation Criteria for Semi-Supervised Learning with Weakly Labeled Data
Journal of Machine Learning Research(2010)
摘要
In this paper, we present an overview of generalized expectation criteria (GE), a simple, robust, scalable method for semi-supervised training using weakly-labeled data. GE fits model parameters by favoring models that match certain expectation constraints, such as marginal label distributions, on the unlabeled data. This paper shows how to apply generalized expectation criteria to two classes of parametric models: maximum entropy models and conditional random fields. Experimental results demonstrate accuracy improvements over supervised training and a number of other state-of-the-art semi-supervised learning methods for these models.
更多查看译文
关键词
accuracy improvement,logistic regression,state-of-the-art semi-supervised learning method,generalized expectation criterion,condi- tional random fields,semi-supervised training,conditional random field,semi-supervised learn ing,supervised training,generalized expectation criteria,semi-supervised learning,certain expectation constraint,weakly-labeled data,weakly labeled data,unlabeled data,parametric model,semi supervised learning,random field,conditional random fields,maximum entropy model
AI 理解论文
溯源树
样例
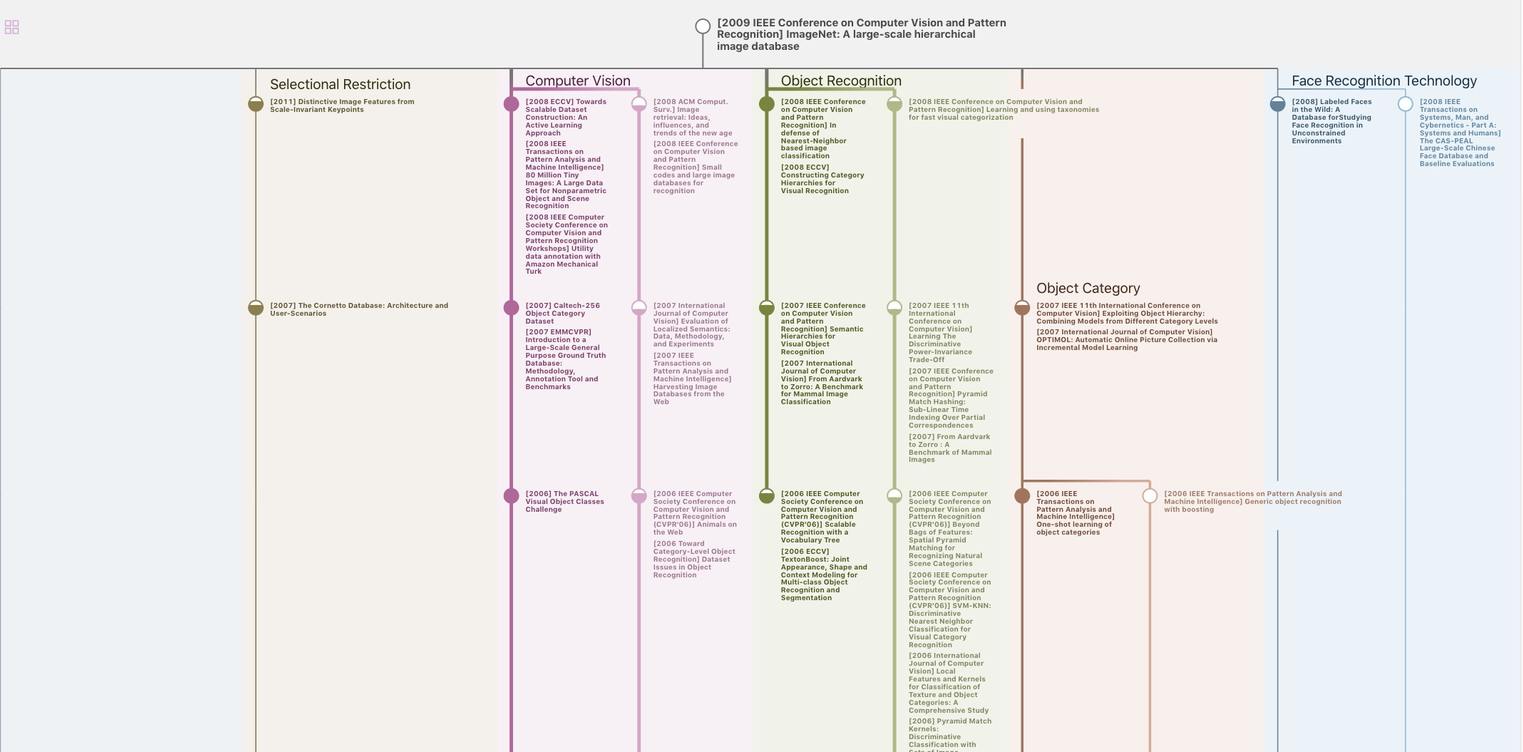
生成溯源树,研究论文发展脉络
Chat Paper
正在生成论文摘要