Optimal and minimax three-stage designs for phase II oncology clinical trials.
Contemporary clinical trials(2008)
摘要
The common objective of oncology phase II trials is to evaluate the anti-tumor activity of a new agent and to determine whether the new drug warrants further investigation. For cancer drugs that significantly shrink tumors, response (CR and PR) rate is usually the primary endpoint in cancer phase II trials for testing H(0): Por=P(1), where P(0) and P(1) are response rates which does not or does warrant further investigation given the rate of false positive (alpha) and false negative (beta). Multiple-stage designs including two-stage and three-stage have been developed by several authors. For example, Simon's optimal two-stage design [Simon R. Optimal two-stage designs for phase II clinical trials. Control Clin Trials 1989;10:1-10], Ensign et al. optimal three-stage design with restriction at the first stage [Ensign LG, Gehan EA, Kamen DS, Thall PF. An optimal three-stage design for phase II clinical trials. Stat Med 1994;13:1727-1736], Chen's optimal three-stage design without any restriction [Chen TT. Optimal three-stage designs for phase II clinical trials. Stat Med 1997;16:2701-2711], etc. However, all the above designs only early terminate a trial due to lack of activity of the study drug. Fleming's multiple-stage design [Fleming TR. One-sample multiple testing procedure for phase II clinical trials. Biometrics 1982;38:143-151] allows early stopping for either sufficient activity or lack of activity. But his design does not attempt to optimize its efficiency. We extend Chen's [Chen TT. Optimal three-stage designs for phase II clinical trials Stat Med 1997;16:2701-2711] design and propose an optimal and a minimax design for three-stage cancer phase II trials which allows early stopping under both hypotheses. The design is optimal in the sense that the average sample number (ASN) is minimized under P=P(0). The minimax design minimizes the maximal sample size (N) and then given this value of N minimizes the average sample number under P=P(0).
更多查看译文
AI 理解论文
溯源树
样例
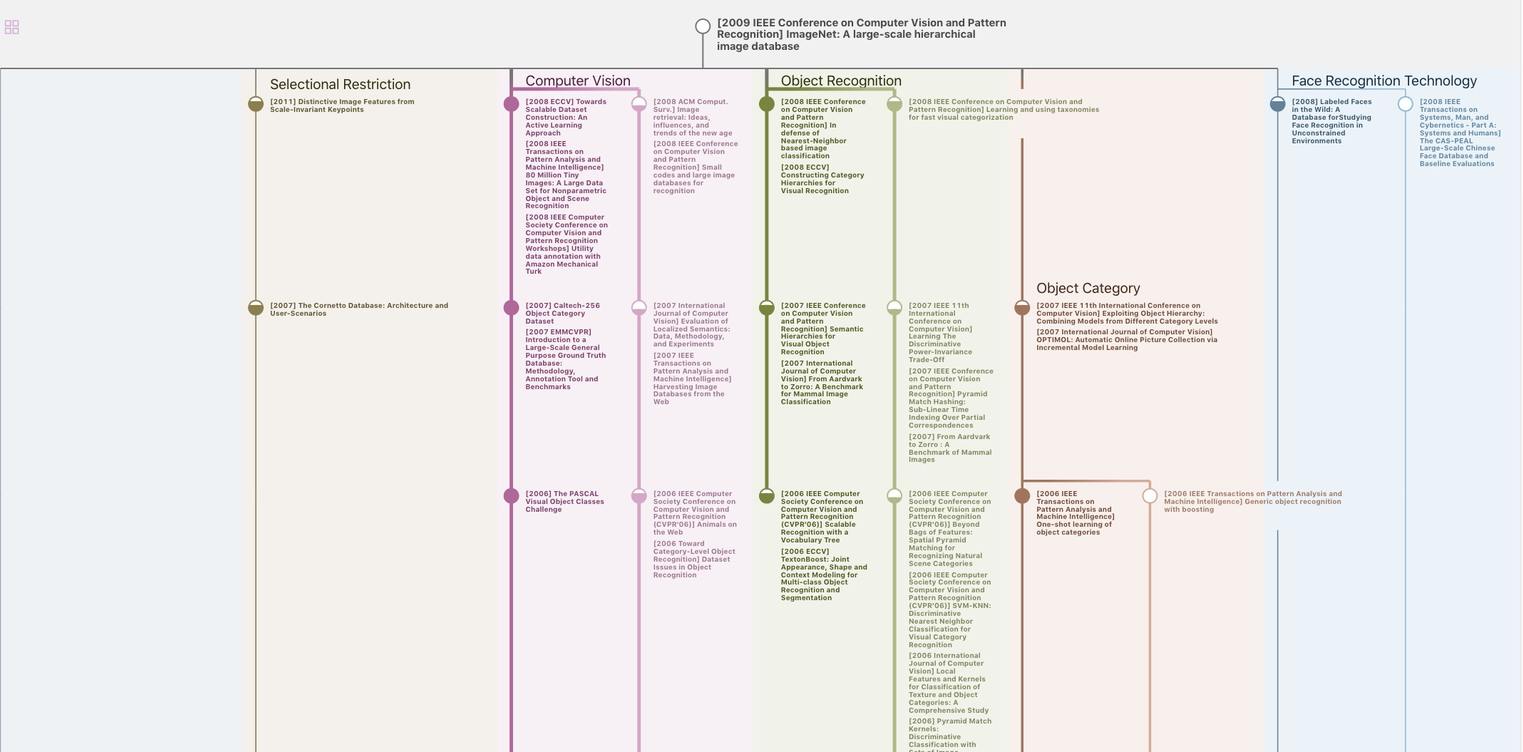
生成溯源树,研究论文发展脉络
Chat Paper
正在生成论文摘要