Query understanding enhanced by hierarchical parsing structures
ASRU(2013)
摘要
Query understanding has been well studied in the areas of information retrieval and spoken language understanding (SLU). There are generally three layers of query understanding: domain classification, user intent detection, and semantic tagging. Classifiers can be applied to domain and intent detection in real systems, and semantic tagging (or slot filling) is commonly defined as a sequence-labeling task - mapping a sequence of words to a sequence of labels. Various statistical features (e.g., n-grams) can be extracted from annotated queries for learning label prediction models; however, linguistic characteristics of queries, such as hierarchical structures and semantic relationships, are usually neglected in the feature extraction process. In this work, we propose an approach that leverages linguistic knowledge encoded in hierarchical parse trees for query understanding. Specifically, for natural language queries, we extract a set of syntactic structural features and semantic dependency features from query parse trees to enhance inference model learning. Experiments on real natural language queries show that augmenting sequence labeling models with linguistic knowledge can improve query understanding performance in various domains.
更多查看译文
关键词
computational linguistics,semantic tagging,sequence-labeling task,syntactic structural feature,semantic dependency feature,label prediction model,slu,natural language queries,hierarchical parse trees,linguistic characteristic,statistical analysis,user intent detection,hierarchical parsing structure,query parse trees,statistical feature,information retrieval,linguistic knowledge,feature extraction,inference model learning,linguistic parsing,grammars,domain classification,natural language processing,n-grams,slot filling,spoken language understanding,query understanding,query processing
AI 理解论文
溯源树
样例
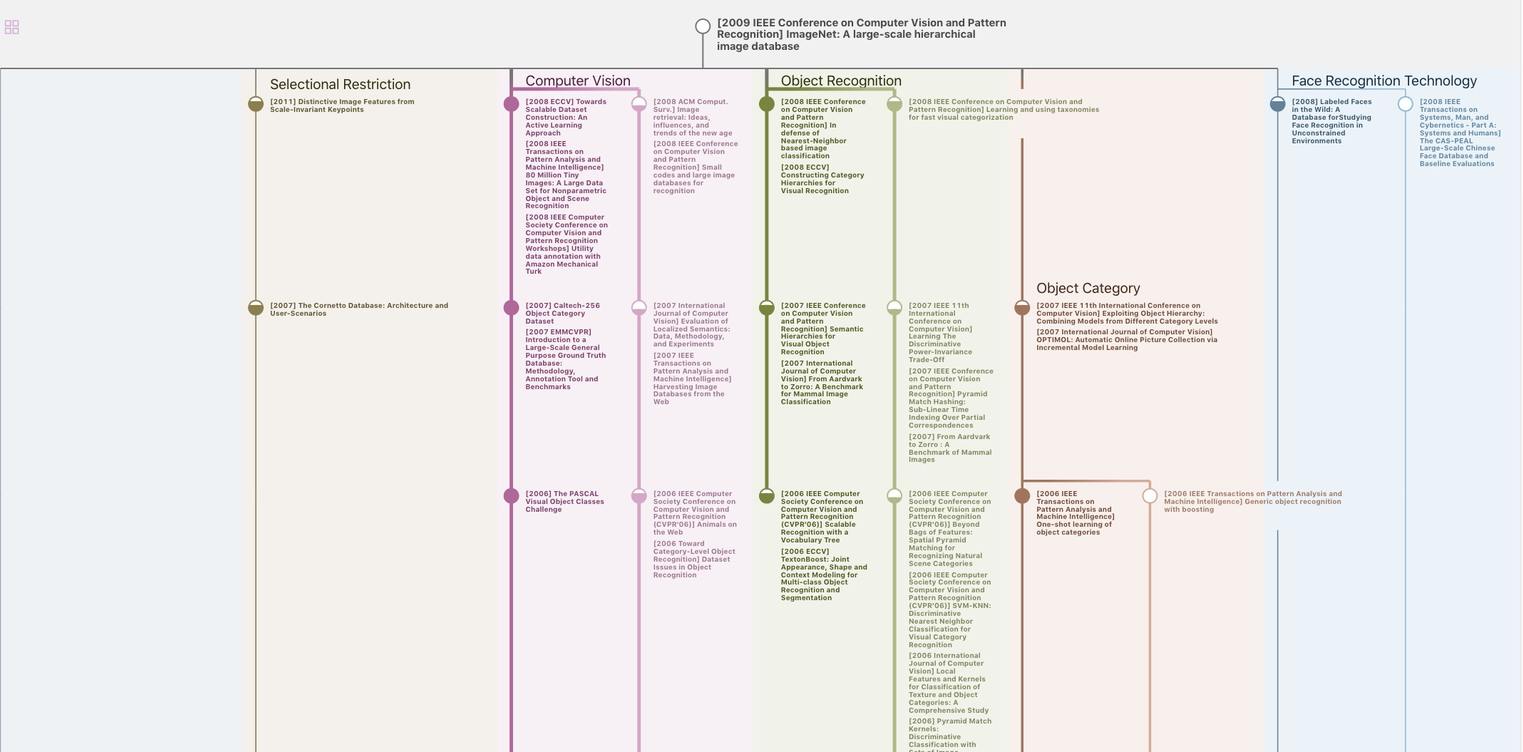
生成溯源树,研究论文发展脉络
Chat Paper
正在生成论文摘要