Pairwise preference regression for cold-start recommendation.
RECSYS(2009)
摘要
ABSTRACTRecommender systems are widely used in online e-commerce applications to improve user engagement and then to increase revenue. A key challenge for recommender systems is providing high quality recommendation to users in ``cold-start" situations. We consider three types of cold-start problems: 1) recommendation on existing items for new users; 2) recommendation on new items for existing users; 3) recommendation on new items for new users. We propose predictive feature-based regression models that leverage all available information of users and items, such as user demographic information and item content features, to tackle cold-start problems. The resulting algorithms scale efficiently as a linear function of the number of observations. We verify the usefulness of our approach in three cold-start settings on the MovieLens and EachMovie datasets, by comparing with five alternatives including random, most popular, segmented most popular, and two variations of Vibes affinity algorithm widely used at Yahoo! for recommendation.
更多查看译文
AI 理解论文
溯源树
样例
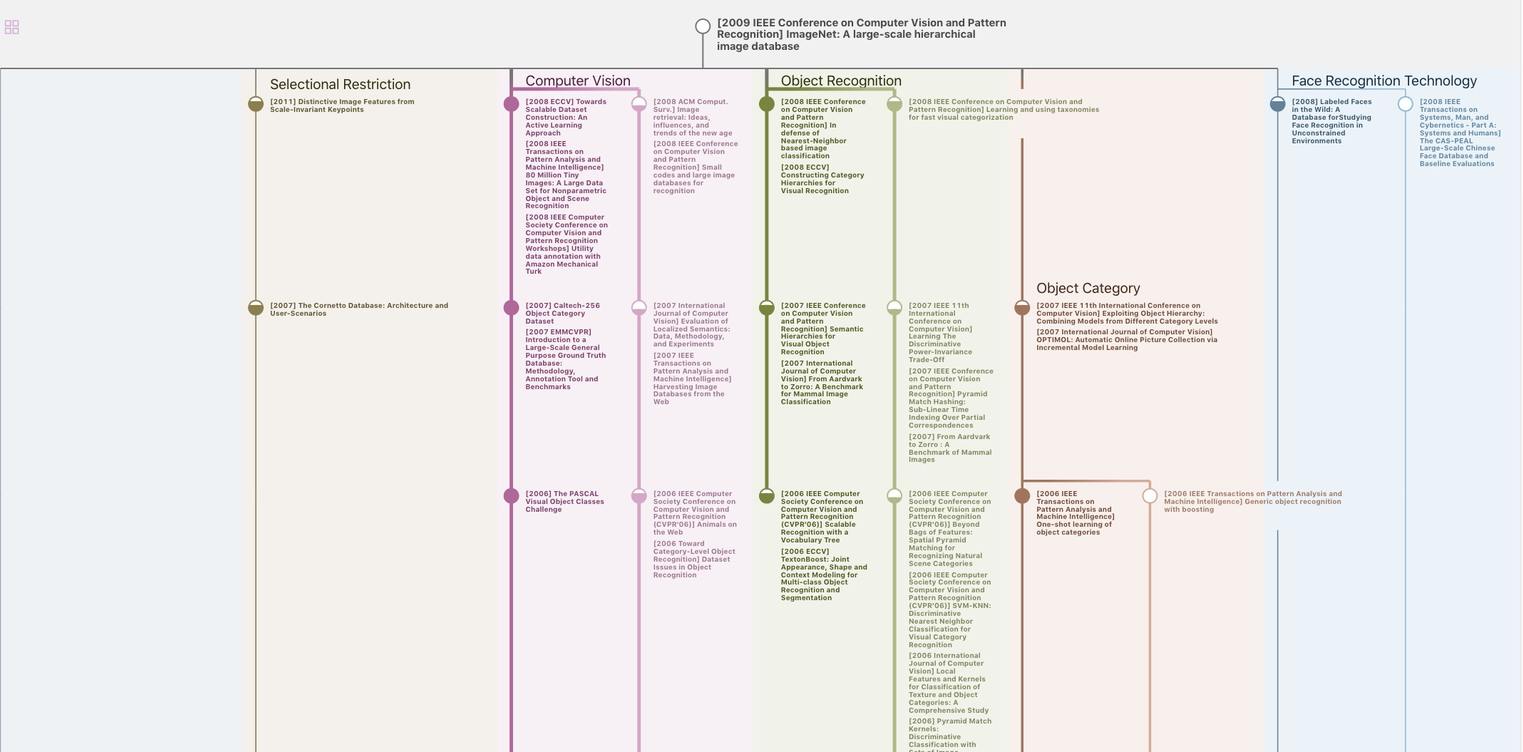
生成溯源树,研究论文发展脉络
Chat Paper
正在生成论文摘要