Listening to the City, Attentively: A Spatio-Temporal Attention-Boosted Autoencoder for the Short-Term Flow Prediction Problem.
Algorithms(2022)
摘要
In recent years, the importance of studying traffic flows and making predictions on alternative mobility (sharing services) has become increasingly important, as accurate and timely information on the travel flow is important for the successful implementation of systems that increase the quality of sharing services. This need has been accentuated by the current health crisis that requires alternative transport mobility such as electric bike and electric scooter sharing. Considering the new approaches in the world of deep learning and the difficulty due to the strong spatial and temporal dependence of this problem, we propose a framework, called STREED-Net, with multi-attention (Spatial and Temporal) able to better mining the high-level spatial and temporal features. We conduct experiments on three real datasets to predict the Inflow and Outflow of the different regions into which the city has been divided. The results indicate that the proposed STREED-Net model improves the state-of-the-art for this problem.
更多查看译文
关键词
spatial correlation,temporal correlation,autoencoder architecture,time-distributed convolutions,attention mechanism,vehicle flow prediction
AI 理解论文
溯源树
样例
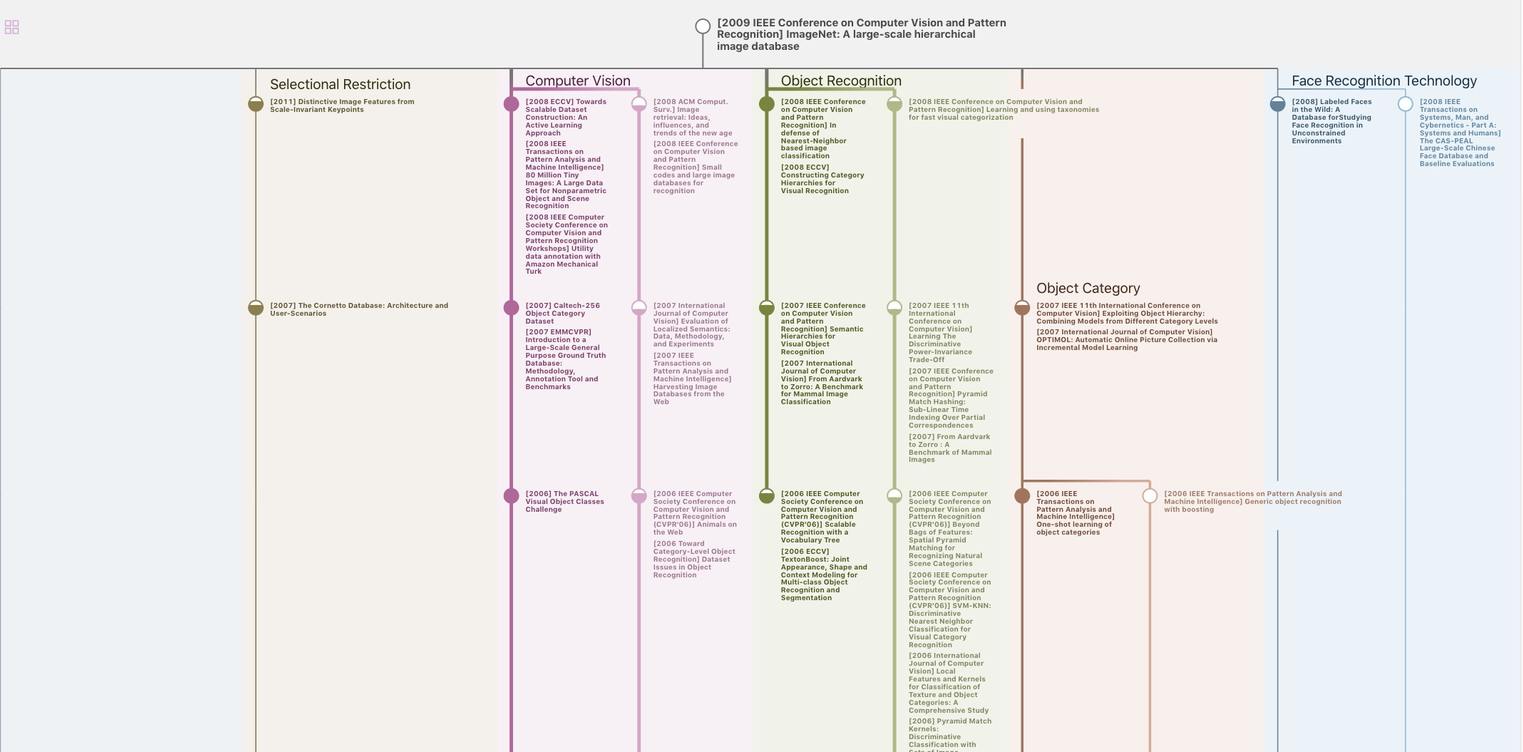
生成溯源树,研究论文发展脉络
Chat Paper
正在生成论文摘要