Comprehensible classification models: a position paper.
ACM SIGKDD Explorations Newsletter(2013)
摘要
The vast majority of the literature evaluates the performance of classification models using only the criterion of predictive accuracy. This paper reviews the case for considering also the comprehensibility (interpretability) of classification models, and discusses the interpretability of five types of classification models, namely decision trees, classification rules, decision tables, nearest neighbors and Bayesian network classifiers. We discuss both interpretability issues which are specific to each of those model types and more generic interpretability issues, namely the drawbacks of using model size as the only criterion to evaluate the comprehensibility of a model, and the use of monotonicity constraints to improve the comprehensibility and acceptance of classification models by users.
更多查看译文
关键词
Bayesian network classifiers,decision table,decision tree,monotonicity constraint,nearest neighbors,rule induction
AI 理解论文
溯源树
样例
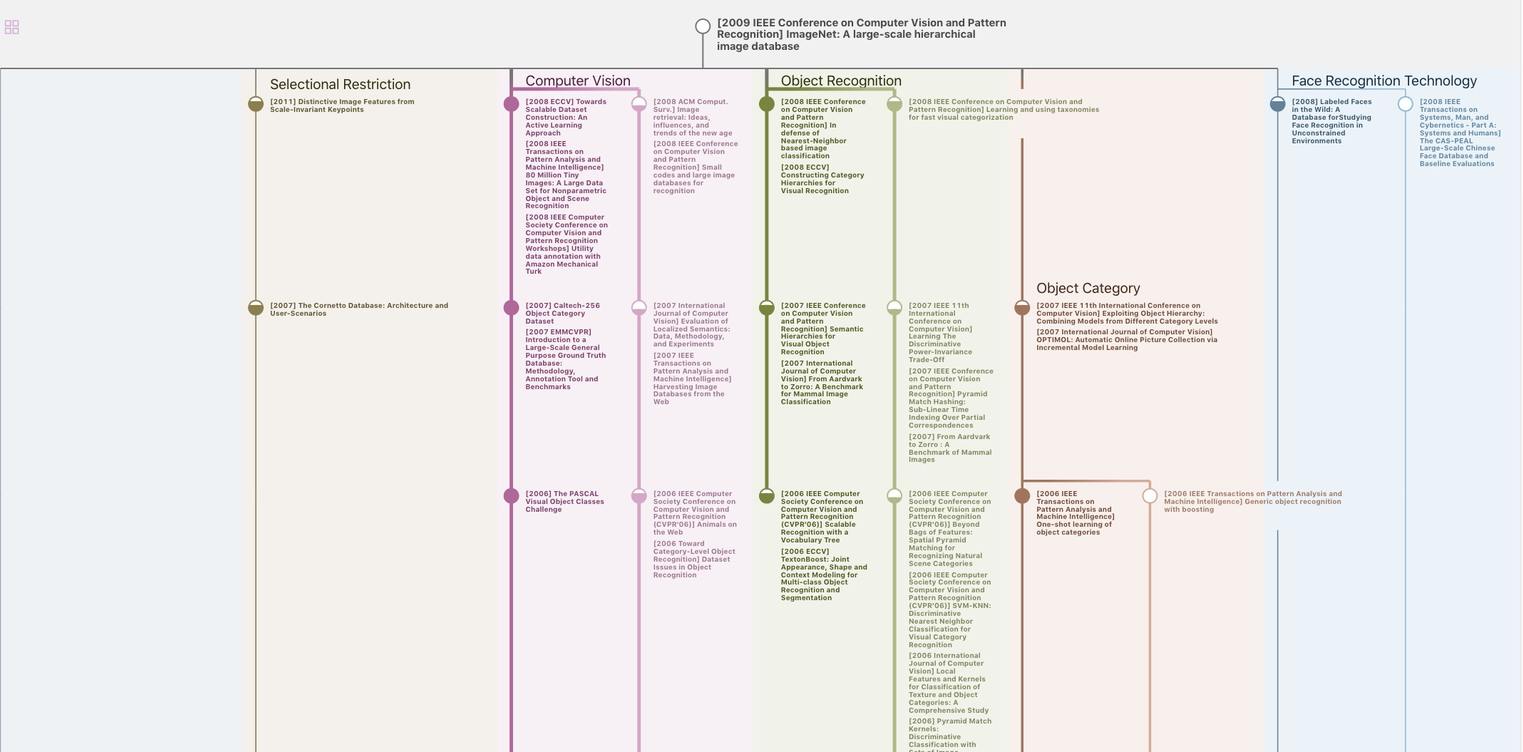
生成溯源树,研究论文发展脉络
Chat Paper
正在生成论文摘要