Good Appearance And 3d Shape Descriptors For Object Category Recognition
Proceedings, Part I, of the 9th International Symposium on Advances in Visual Computing - Volume 8033(2015)
摘要
For the problem of object category recognition, we have studied different families of descriptors exploiting RGB and 3D information. We have proven practically that 3D shape-based descriptors are more suitable for this type of recognition due to low shape intra-class variance, as opposed to texture-based. Performance evaluation on training-set subsampling, suggests that the viewpoint invariance characteristics of 3D descriptors, favors significantly these descriptors while invariant SIFT descriptors can be ambiguous. In addition, we have also shown how an efficient Naive Bayes Nearest Neighbor (NBNN) classifier can scale to a large hierarchical RGB-D Object Dataset and achieve, with a single descriptor type, an accuracy close to state-of-the-art learning-based approaches using combined descriptors.
更多查看译文
关键词
NBNN, 3D surface descriptors, RGB-D, feature matching, object category recognition
AI 理解论文
溯源树
样例
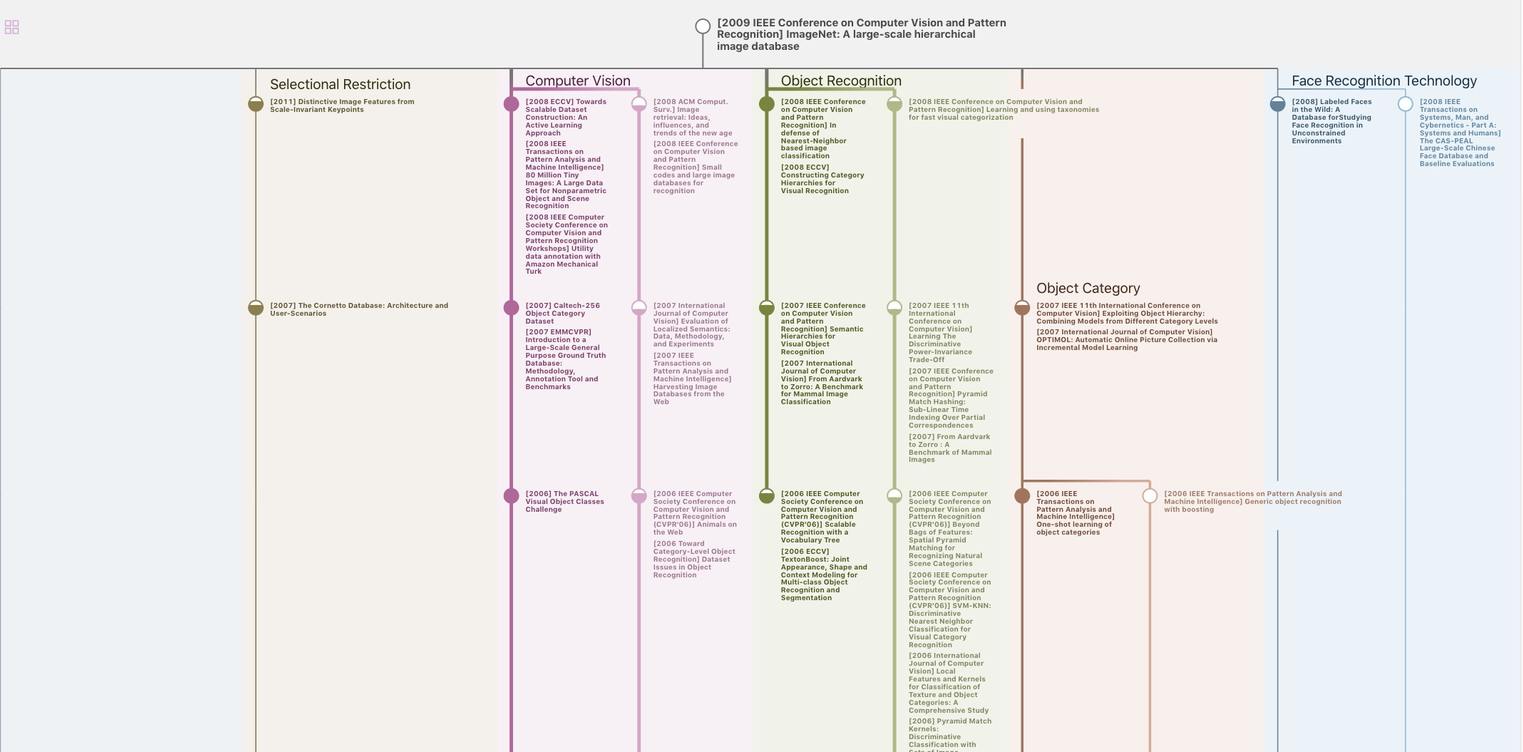
生成溯源树,研究论文发展脉络
Chat Paper
正在生成论文摘要