How about utilizing ordinal information from the distribution of unlabeled data.
CIKM '10: International Conference on Information and Knowledge Management Toronto ON Canada October, 2010(2010)
摘要
Problems of ordinal regression arise in many fields such as information retrieval, data mining and knowledge management. In this paper, we consider ordinal regression in a semi-supervised scenario, i.e., we try to utilize the ordinal information from the distribution of unlabeled data. Semi-supervised ordinal regression is more applicable than traditional supervised ordinal regression, because nowadays labeled data is expensive and time-consuming as it needs human labor, whereas a large amount of unlabeled data are far accessible with the development of internet technology. We construct a general semi-supervised ordinal regression framework to formulate this problem. Based on the framework, we then propose a semi-supervised ordinal regression method called Semi-supervised Ordinal SVM (SOSVM). Additionally, in order to make our proposed method more applicable to problems with large scaled labeled data, we put forward a kernel based dual coordinate descent algorithm to efficiently solve SOSVM. Both rigorous theoretical analysis and promising experimental evaluations on real world datasets show the great performance and remarkable efficiency of SOSVM.
更多查看译文
AI 理解论文
溯源树
样例
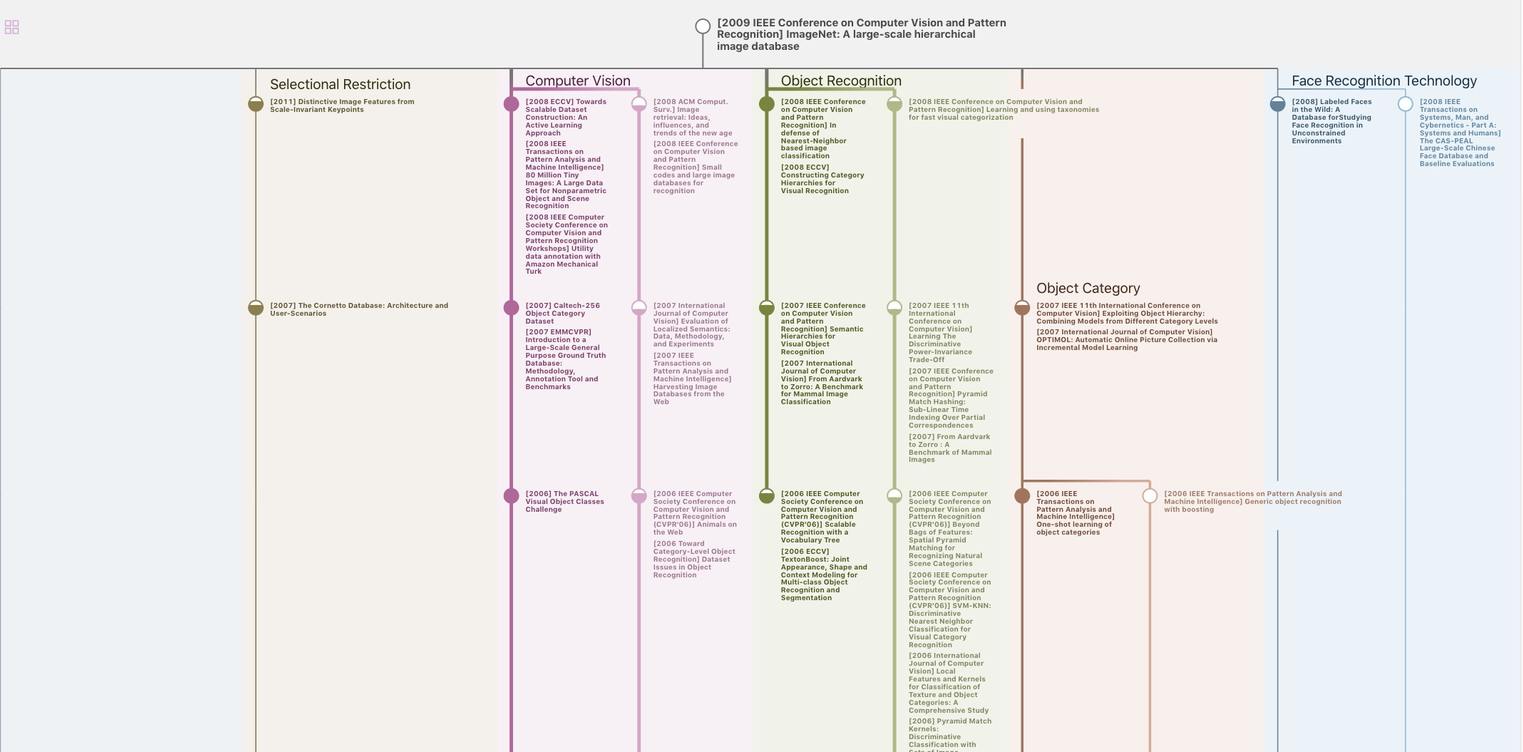
生成溯源树,研究论文发展脉络
Chat Paper
正在生成论文摘要