A Hidden Markov Model-based approach for recognizing swimmer's behaviors in swimming pool
ICMLC(2010)
摘要
This paper employs a HMM (Hidden Markov Model) methodology to recognize some specific-behaviors of a swimmer in the swimming pool. In this study, a swimmer's behavior is composed of a series of the static image-frames. For each frame, a Convexity-Structure is used to enclose the swimmer's shape after segmenting the swimmer-blob. A codebook is created for mapping a swimmer-blob into one of 6 feature blob-types during training and recognition stage. Consequently, the time-sequential blobs are converted to a feature-vector sequence and transformed into a symbolic-sequence by the existed codebook. Thus a learned HMM can be obtained by this symbolic-sequence from a given specific-behavior and used to recognize a swimmer's behavior which is normal or abnormal. Four different swimming styles (Backstroke, Breaststroke, Freestyle, and Butterfly) are examined. An average recognition rate of 90% is obtained.
更多查看译文
关键词
learning (artificial intelligence),video surveillance,image segmentation,swimming style,codebook,image recognition,behavioural sciences computing,convexity structure,abnormal behavior detection,swimmer-blob segmentation,hidden markov models,static image-frames,swimmer behavior recognition,hidden markov model (hmm),symbolic sequence,swimmer-blob mapping,hidden markov model,feature-vector sequence,time-sequential blobs,feature vector,feature extraction,shape,learning artificial intelligence,markov processes,probability,cybernetics,machine learning
AI 理解论文
溯源树
样例
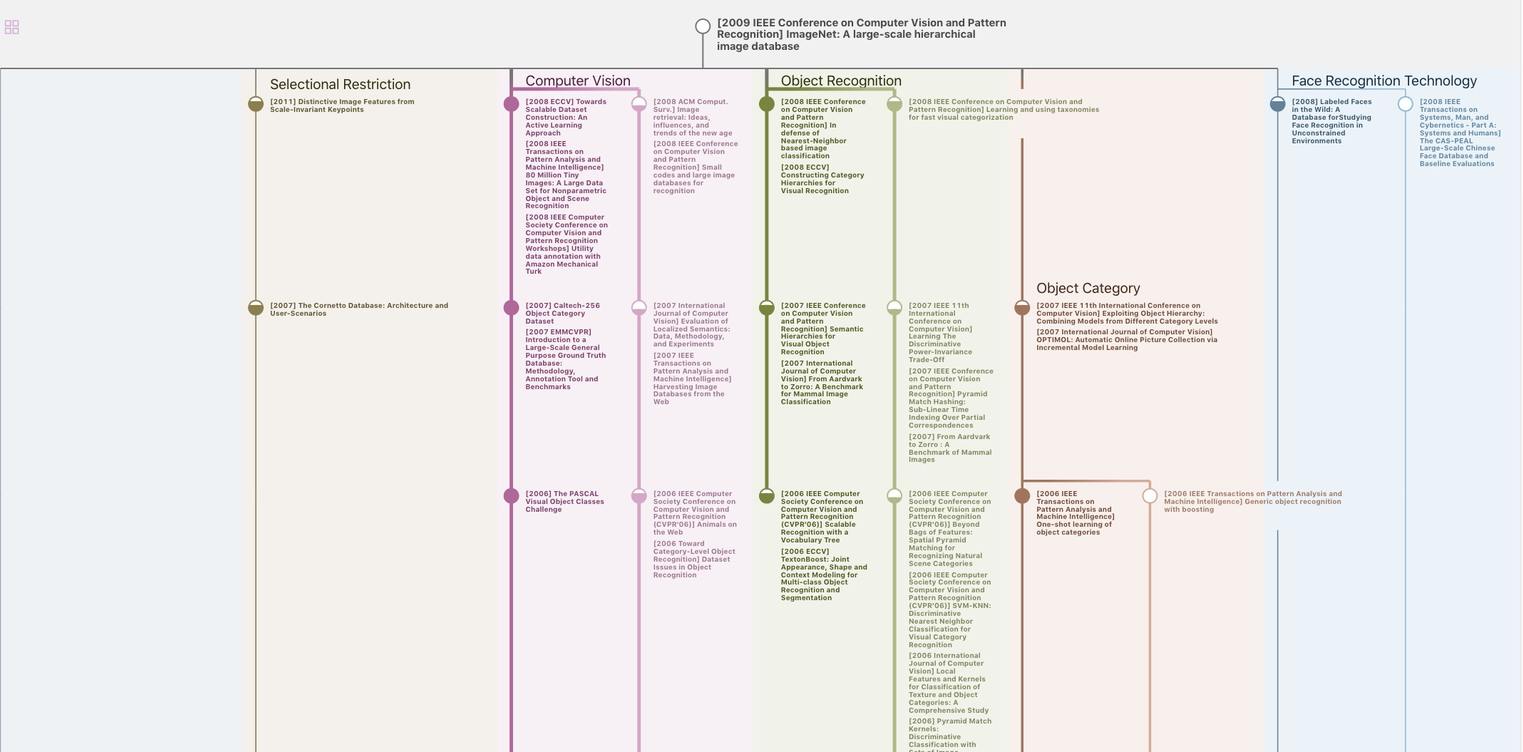
生成溯源树,研究论文发展脉络
Chat Paper
正在生成论文摘要