Personalized click shaping through lagrangian duality for online recommendation.
IR(2012)
摘要
ABSTRACTOnline content recommendation aims to identify trendy articles in a continuously changing dynamic content pool. Most of existing works rely on online user feedback, notably clicks, as the objective and maximize it by showing articles with highest click-through rates. Recently, click shaping was introduced to incorporate multiple objectives in a constrained optimization framework. The work showed that significant tradeoff among the competing objectives can be observed and thus it is important to consider multiple objectives. However, the proposed click shaping approach is segment-based and can only work with a few non-overlapping user segments. It remains a challenge of how to enable deep personalization in click shaping. In this paper, we tackle the challenge by proposing personalized click shaping. The main idea is to work with the Lagrangian duality formulation and explore strong convexity to connect dual and primal solutions. We show that our formulation not only allows efficient conversion from dual to primal for online personalized serving, but also enables us to solve the optimization faster by approximation. We conduct extensive experiments on a large real data set and our experimental results show that the personalized click shaping can significantly outperform the segmented one, while achieving the same ability to balance competing objectives.
更多查看译文
AI 理解论文
溯源树
样例
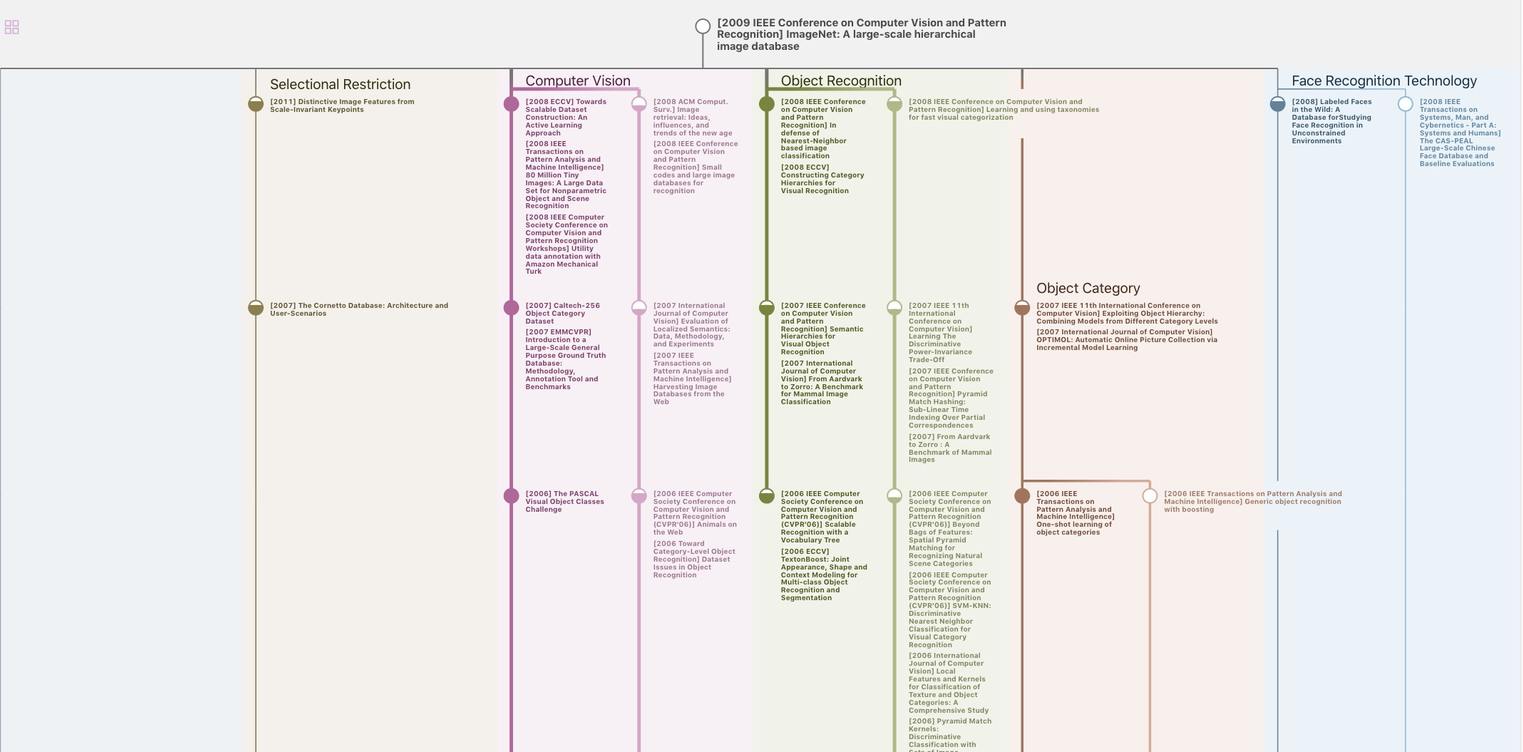
生成溯源树,研究论文发展脉络
Chat Paper
正在生成论文摘要