Evolutionary optimization of transition probability matrices for credit decision-making
European Journal of Operational Research(2010)
摘要
Statistical transition probability matrices (TPMs), which indicate the likelihood of obligor credit state migration over a certain time horizon, have been used in various credit decision-making applications. A standard approach of calculating TPMs is to form a one-year empirical TPM and then project it into the future based on Markovian and time-homogeneity assumptions. However, the one-year empirical TPM calculated from historical data generally does not satisfy desired properties. We propose an alternative methodology by formulating the problem as a constrained optimization problem requiring satisfaction of all the desired properties and minimization of the discrepancy between predicted multi-year TPMs and empirical evidence. The problem is high-dimensional, non-convex, and non-separable, and is not effectively solved by nonlinear programming methods. To address the difficulty, we investigated evolutionary algorithms (EAs) and problem representation schemas. A self-adaptive differential evolution algorithm JADE, together with a new representation schema that automates constraint satisfaction, is shown to be the most effective technique.
更多查看译文
关键词
Risk management,Finance,Evolutionary computations,Constraints satisfaction,Decision support systems
AI 理解论文
溯源树
样例
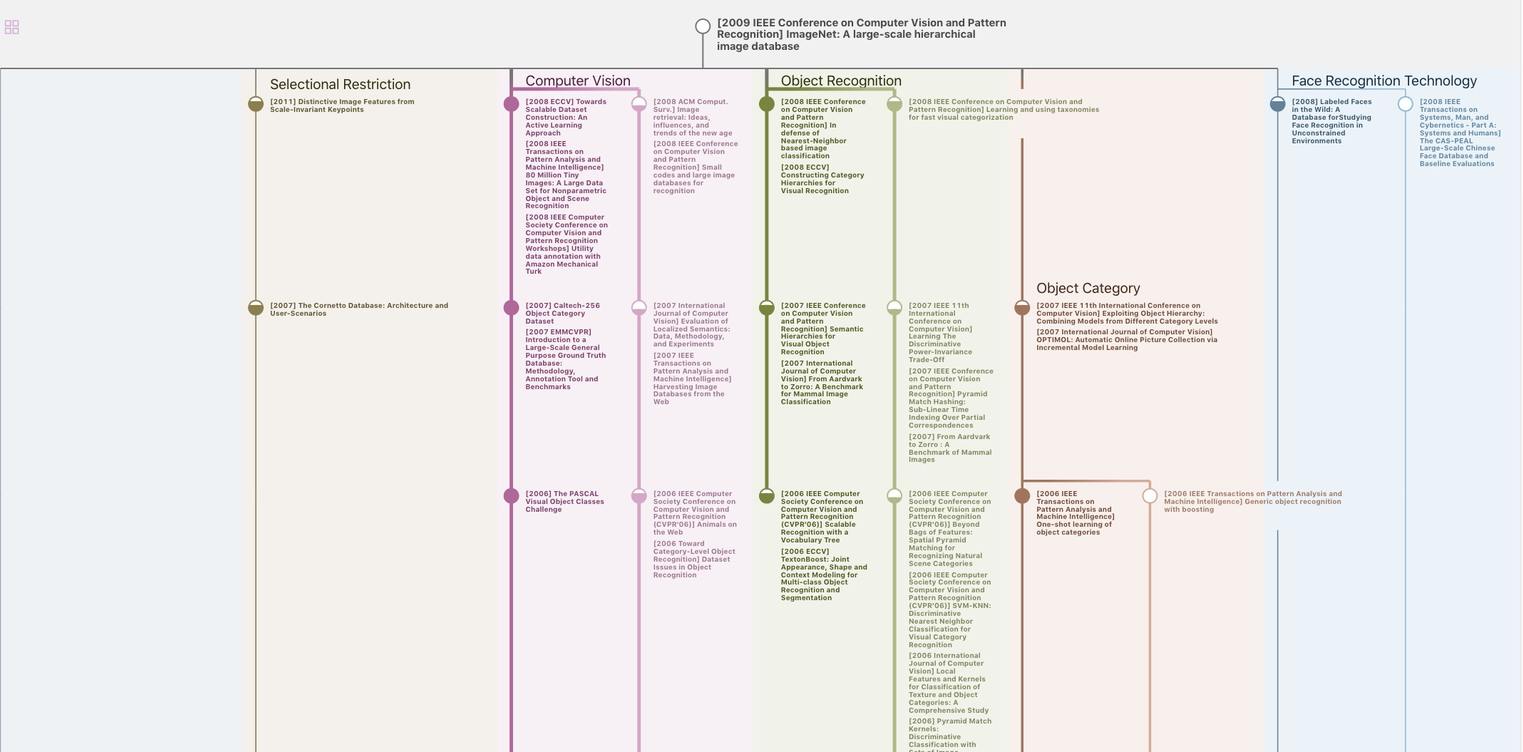
生成溯源树,研究论文发展脉络
Chat Paper
正在生成论文摘要