Learning to extract cross-session search tasks
WWW(2013)
摘要
Search tasks, comprising a series of search queries serving the same information need, have recently been recognized as an accurate atomic unit for modeling user search intent. Most prior research in this area has focused on short-term search tasks within a single search session, and heavily depend on human annotations for supervised classification model learning. In this work, we target the identification of long-term, or cross-session, search tasks (transcending session boundaries) by investigating inter-query dependencies learned from users' searching behaviors. A semi-supervised clustering model is proposed based on the latent structural SVM framework, and a set of effective automatic annotation rules are proposed as weak supervision to release the burden of manual annotation. Experimental results based on a large-scale search log collected from Bing.com confirms the effectiveness of the proposed model in identifying cross-session search tasks and the utility of the introduced weak supervision signals. Our learned model enables a more comprehensive understanding of users' search behaviors via search logs and facilitates the development of dedicated search-engine support for long-term tasks.
更多查看译文
关键词
semi-supervised clustering model,search log,user search intent,search behavior,search task,cross-session search task,large-scale search,single search session,short-term search task
AI 理解论文
溯源树
样例
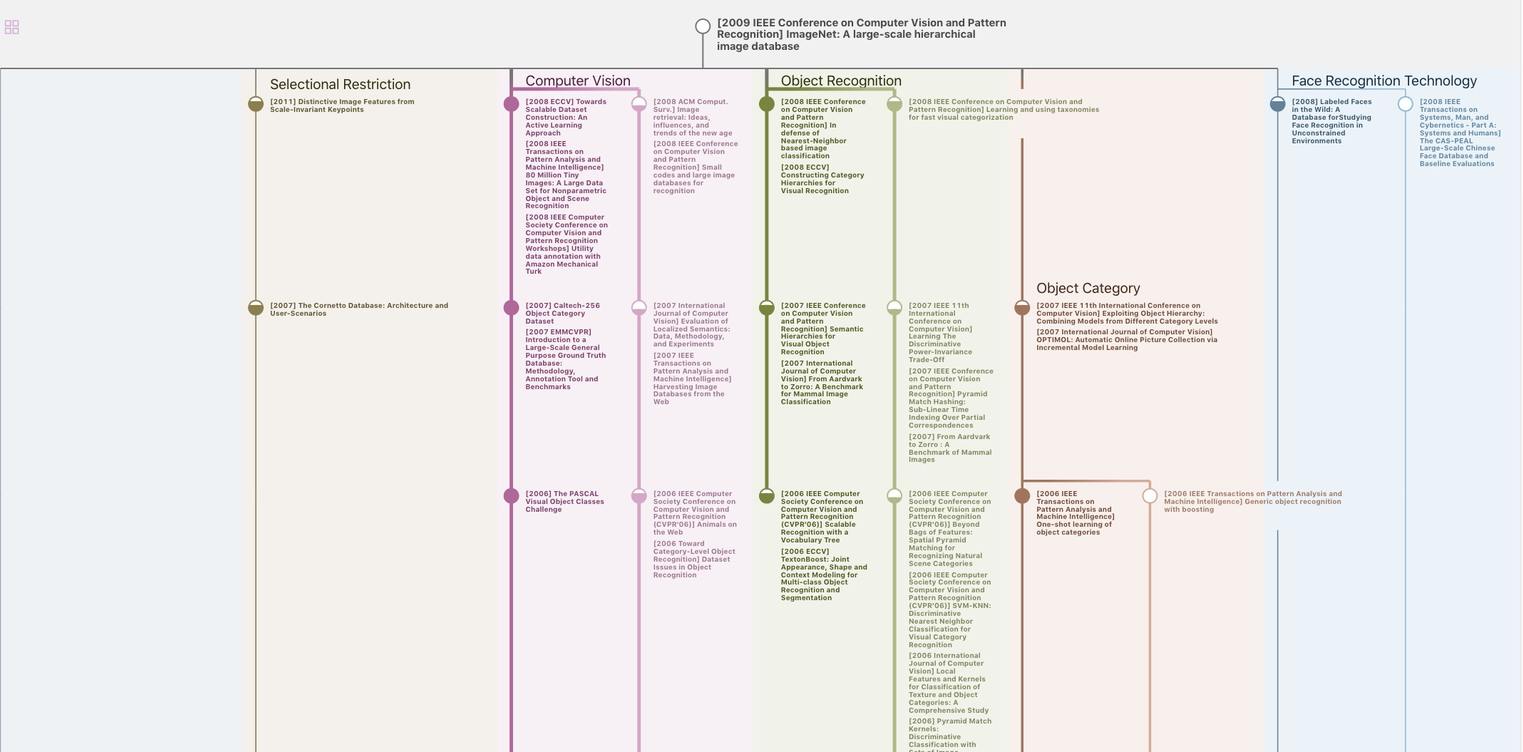
生成溯源树,研究论文发展脉络
Chat Paper
正在生成论文摘要