Cross-validation based decision tree clustering for HMM-based TTS
ICASSP(2010)
摘要
In HMM-based speech synthesis, we usually use complex, context dependent models to characterize prosodically and linguistically rich speech units. It is therefore difficult to prepare training data which can cover all combinatorial possibilities of contexts. A common approach to cope with this insufficient training data problem is to build a clustered tree via the MDL criterion. However, an MDL-based tree still tends to be inadequate in its power to predict unseen data. In this paper, we adopt the cross-validation principle to build such a decision tree to minimize the generation error of unseen contexts. An efficient training algorithm is implemented by exploiting the sufficient statistics. Experimental results show that the proposed method can achieve better speech synthesis results, both objectively and subjectively, than the baseline results of the MDL-based decision tree.
更多查看译文
关键词
decision tree clustering,pattern clustering,statistics,statistical analysis,generation error,training algorithm,contexts,hmm-based tts,speech synthesis,hmm-based speech synthesis,mdl,linguistically rich speech units,cross validation,cross-validation,hidden markov models,decision trees,mdl criterion,context clustering,predictive models,sufficient statistic,stress,data models,context modeling,generalization error,decision tree,clustering algorithms,context dependent,speech,training data
AI 理解论文
溯源树
样例
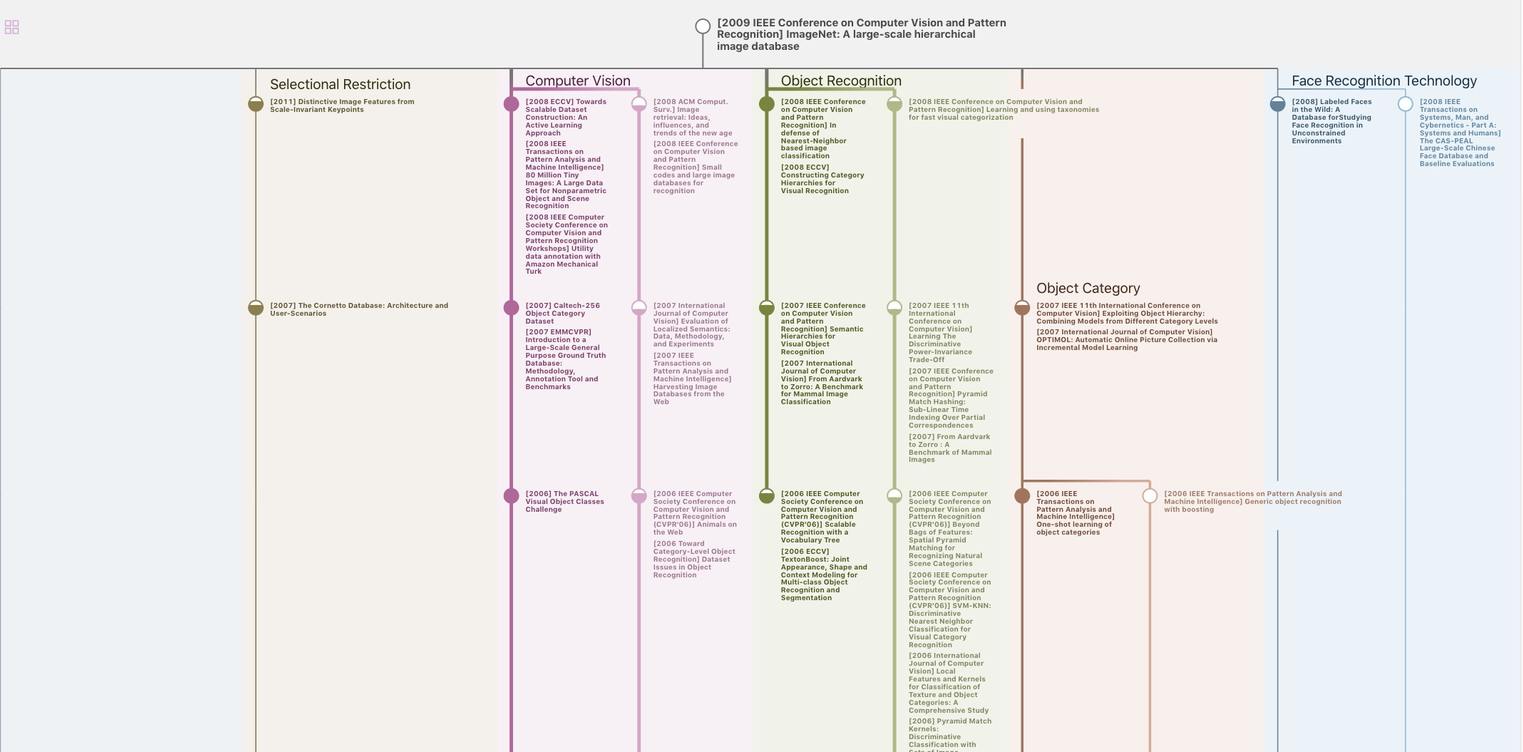
生成溯源树,研究论文发展脉络
Chat Paper
正在生成论文摘要