Circuit timing and leakage power analysis under process variations
Circuit timing and leakage power analysis under process variations(2006)
摘要
In this thesis, we focus on the statistical analysis of timing and leakage power of VLSI circuit under inter-die and intra-die process variations. The effect of spatial correlation in intra-die variation is also considered by proposing a grid-based model. We demonstrate concrete numbers to show that it is essential to account for spatial correlations, in order to predict the circuit performance correctly. In the statistical static timing analysis (SSTA) method, all process variations are approximated as Gaussians, and linear sensitivities of delays to process variations are assumed. To handle spatial correlations, principal component analysis is used to rotate the set of spatially correlated process variables into an independent set. A PERT-like traversal of the circuit graph is then performed using sum and max functions defined analytically on Gaussian random variables. The computational complexity is equivalent to a constant number of deterministic static timing analysis, where the constant is related to number of process variables and spatial grids. The general framework for SSTA is then extended to incorporate non-Gaussian-distributed process variations and/or nonlinear delay sensitivities. Instead of using linear functions of delays, delay is expressed in a generalized form by introducing a "nonlinear non-Gaussian term". The sum and max functions are defined on random variables in generalized forms. The method achieves higher accuracy in predicting circuit timing performance especially at high timing yield levels, while fully preserves its computational efficiency in processing Gaussian process variations and linear delay functions. In statistical analysis of full-chip leakage power, subthreshold and gate-tunneling leakage power are considered. With process variations, each leakage component is approximated by a lognormal distribution, and the total chip leakage is computed as a sum of the lognormals that have complicated correlation structures due to spatial correlations and correlations among different leakage terms. An efficient method is proposed to reduce the number of correlated lognormals for summation by identifying dominant states of leakage currents and taking advantage of the spatial correlation model and input states at gates. An improved approach utilizing principal components of correlated process parameters is also proposed to further improve run-time efficiency.
更多查看译文
关键词
non-Gaussian-distributed process variation,process variation,leakage power analysis,intra-die process variation,statistical analysis,leakage power,spatially correlated process variable,correlated process parameter,circuit timing,Gaussian process variation,process variable,spatial correlation
AI 理解论文
溯源树
样例
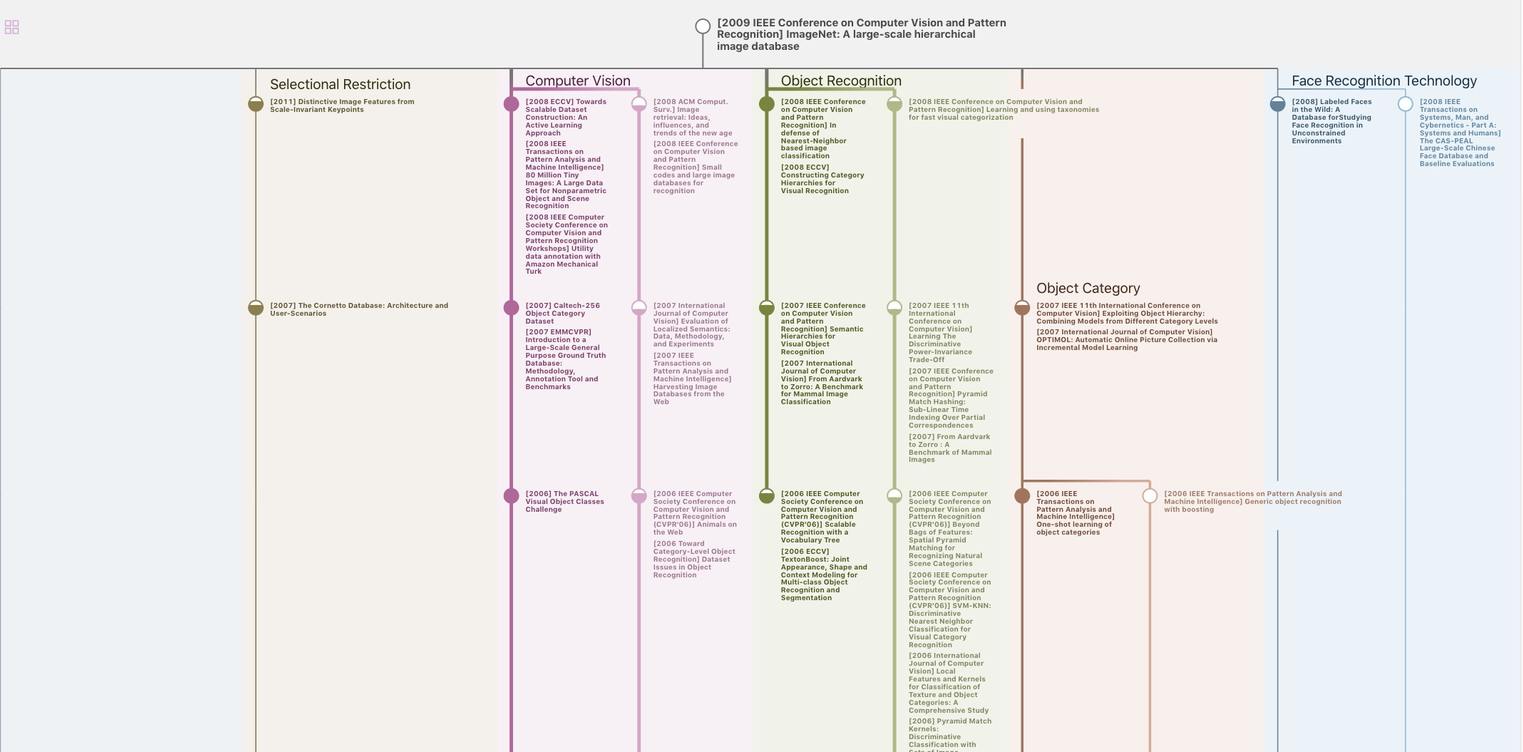
生成溯源树,研究论文发展脉络
Chat Paper
正在生成论文摘要