Different Ways Of Weakening Decision Trees And Their Impact On Classification Accuracy Of Dt Combination
MCS '00: Proceedings of the First International Workshop on Multiple Classifier Systems(2000)
摘要
Recent classifier combination frameworks have proposed several ways of weakening a learning set and have shown that these weakening methods improve prediction accuracy. In the present paper we focus on learning set sampling (Breiman's bagging) and random feature subset selections (Bay's Multiple Feature Subsets). We present a combination scheme labeled 'Bagfs', in which new learning sets are generated on the basis of both bootstrap replicates and selected feature subsets. The performances of the three methods (Bagging, MFS and Bagfs) are assessed by means of a decision-tree inducer (C4.5) and a majority voting rule. In addition, we also study whether the way in which weak classifiers are created has a significant influence on the performance of their combination. To answer this question, we undertook the strict application of the Cochran Q test. This test enabled us to compare the three weakening methods together on a given database, and to conclude whether or not these methods differ significantly. We also used the McNemar test to compare algorithms pair by pair. The first results, obtained on 14 conventional databases, show that on average, Bagfs exhibits the best agreement between prediction and supervision. The Cochran Q test indicated that the weak classifiers so created significantly influenced combination performance in the case of at least 4 of the 14 databases analyzed.
更多查看译文
关键词
Cochran Q test,weakening method,weak classifier,McNemar test,combination performance,combination scheme,recent classifier combination framework,new learning set,algorithms pair,conventional databases,Classification Accuracy,DT Combination,Different Ways,Weakening Decision Trees
AI 理解论文
溯源树
样例
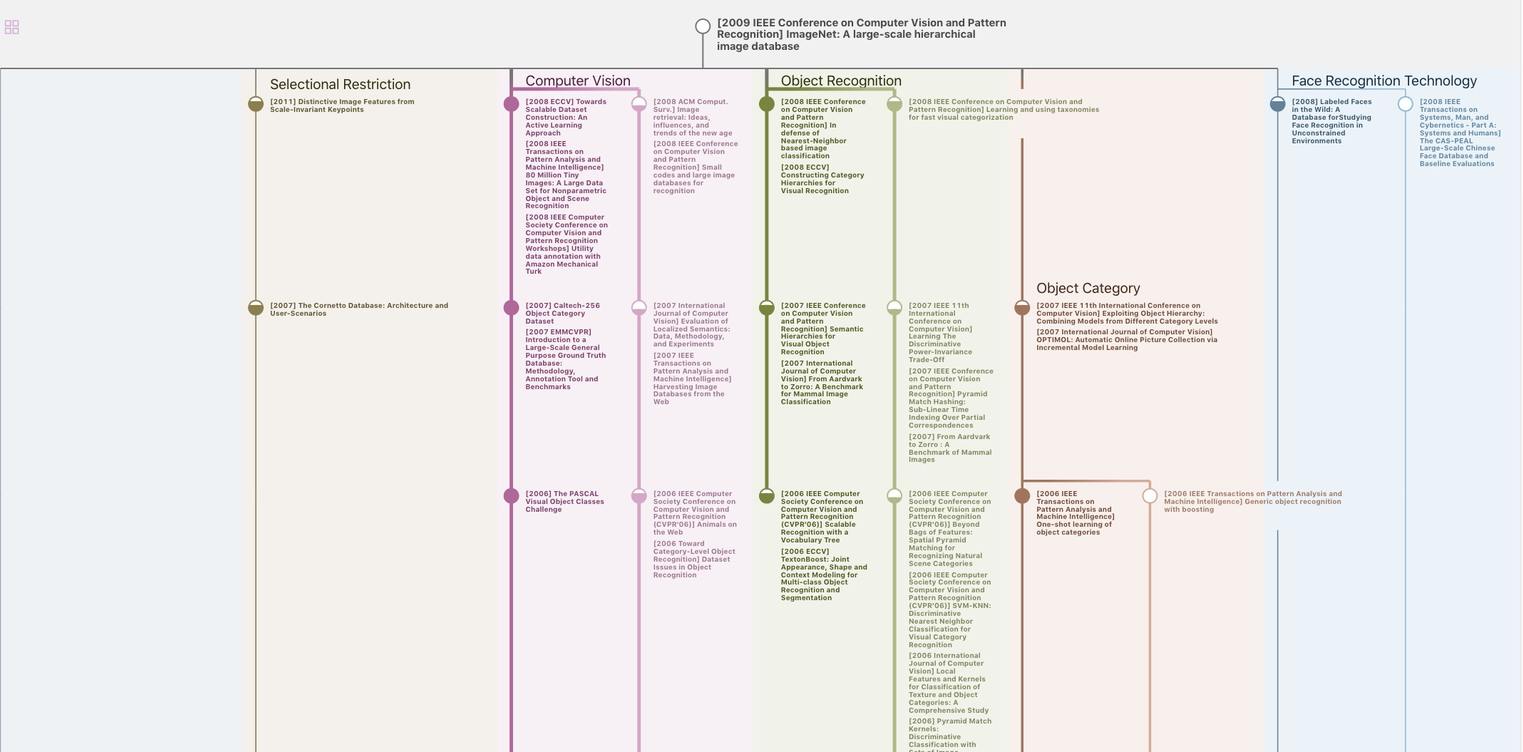
生成溯源树,研究论文发展脉络
Chat Paper
正在生成论文摘要