A Game Playing Robot That Can Learn A Tactical Knowledge Through Interacting With A Human
ICAART 2011: PROCEEDINGS OF THE 3RD INTERNATIONAL CONFERENCE ON AGENTS AND ARTIFICIAL INTELLIGENCE, VOL 1(2011)
摘要
We propose a new approach for teaching a humanoid-robot a task online without pre-set data provided in advance. In our approach, human acts as a collaborator and also as a teacher. The proposed approach enables the humanoid-robot to learn a task through multi-component interactive architecture. The components are designed with the respect to human methodology for learning a task through empirical interactions. For efficient performance, the components are isolated within one single API. Our approach can be divided into five main roles: perception, representation, state/knowledge-up-dating, decision making and expression. A conducted empirical experiment for the proposed approach is to be done by teaching a Fujitsu's humanoid-robot "Hoap-3" an X-O game strategy and its results are to be done and explained. Important component such as observation, structured interview, knowledge integration and decision making are described for teaching the robot the game strategy while conducting the experiment.
更多查看译文
关键词
Humanoid robot, Game strategy, Learning from observation, Structured interview, Long-term memory, Sensory memory, Working memory
AI 理解论文
溯源树
样例
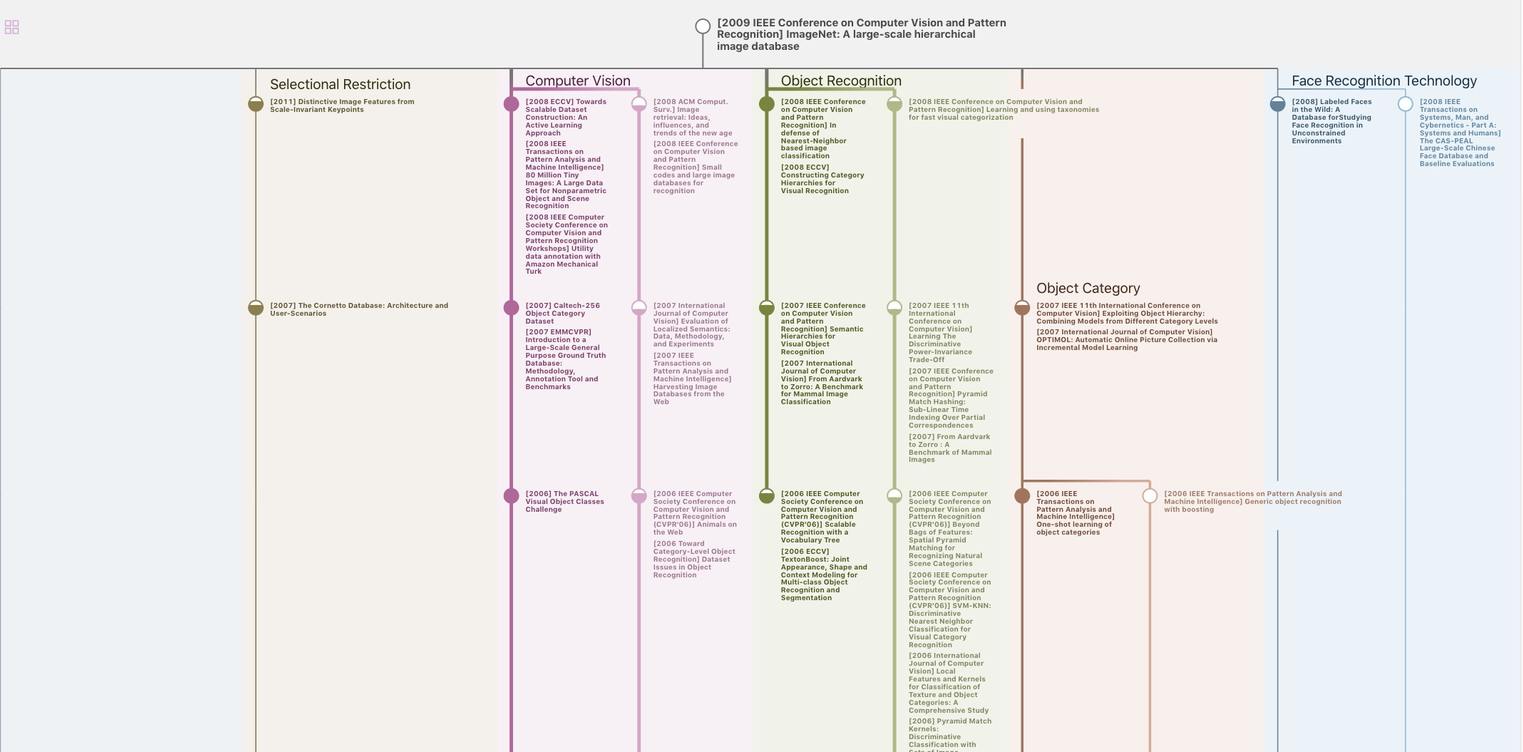
生成溯源树,研究论文发展脉络
Chat Paper
正在生成论文摘要