Towards A Scalable Social Recommender Engine For Online Marketplaces: The Case Of Apache Solr
WWW '14: 23rd International World Wide Web Conference Seoul Korea April, 2014(2014)
摘要
Recent research has unveiled the importance of online social networks for improving the quality of recommenders in several domains, what has encouraged the research community to investigate ways to better exploit the social information for recommendations. However, there is a lack of work that offers details of frameworks that allow an easy integration of social data with traditional recommendation algorithms in order to yield a straight-forward and scalable implementation of new and existing systems. Furthermore, it is rare to find details of performance evaluations of recommender systems such as hardware and software specifications or bench marking results of server loading tests.In this paper we intend to bridge this gap by presenting the details of a social recommender engine for online marketplaces built upon the well-known search engine Apache Solr. We describe our architecture and also share implementation details to facilitate the re-use of our approach by people implementing recommender systems. In addition, we evaluate our framework from two perspectives: (a) recommendation algorithms and data sources, and (b) system performance under server stress tests. Using a dataset from the SecondLife virtual world that has both trading and social interactions, we contribute to research in social recommenders by showing how certain social features allow to improve recommendations in online marketplaces. On the platform implementation side, our evaluation results can serve as a baseline to people searching for performance references in terms of scalability, model training and testing tradeoffs, real-time server performance and the impact of model updates in a production system.
更多查看译文
关键词
social recommender engine,scalability,online marketplaces,Apache Solr
AI 理解论文
溯源树
样例
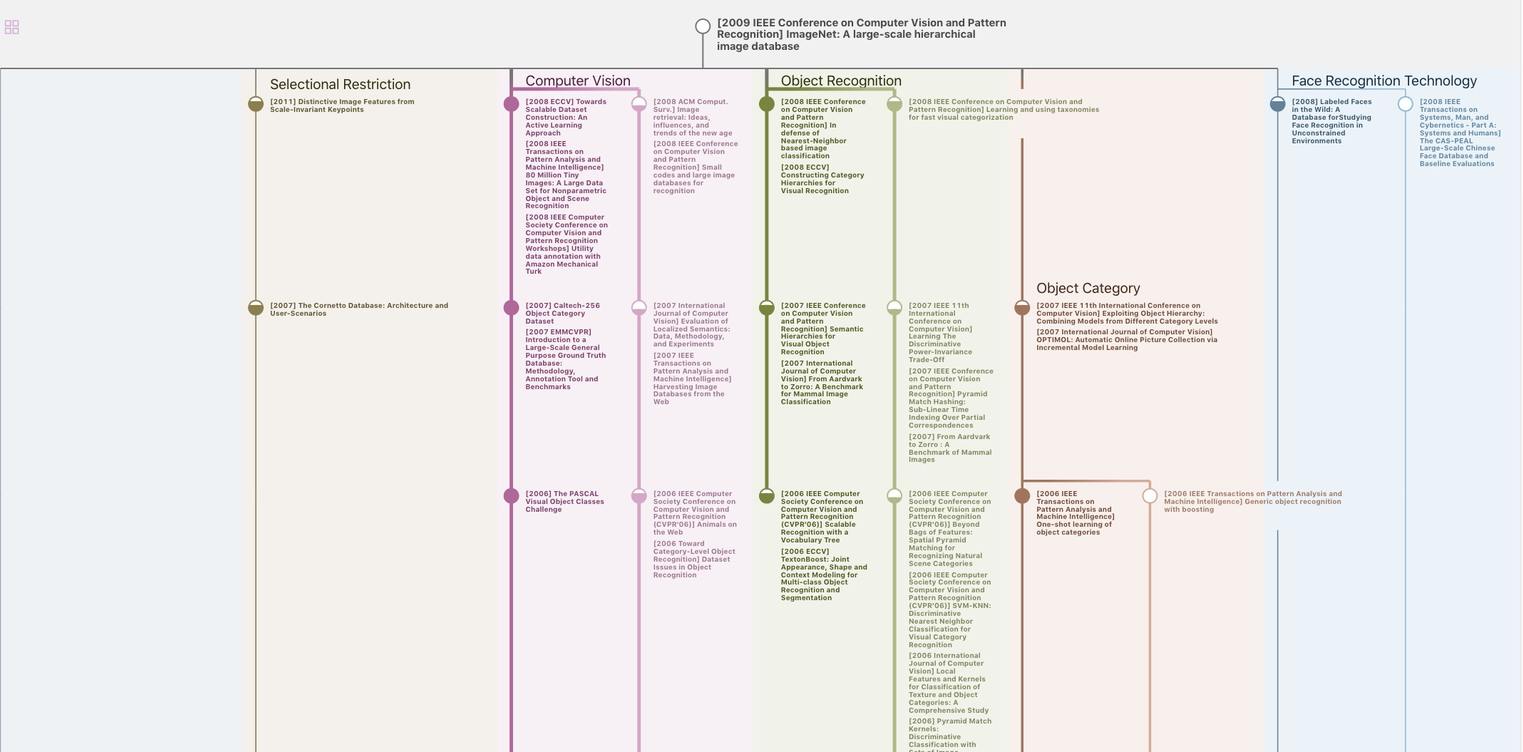
生成溯源树,研究论文发展脉络
Chat Paper
正在生成论文摘要