Restricted Deep Belief Networks For Multi-View Learning
ECML PKDD'11: Proceedings of the 2011 European conference on Machine learning and knowledge discovery in databases - Volume Part II(2011)
摘要
Deep belief network (DBN) is a probabilistic generative model with multiple layers of hidden nodes and a layer of visible nodes, where parameterizations between layers obey harmonium or restricted Boltzmann machines (RBMs). In this paper we present restricted deep belief network (RDBN) for multi-view learning, where each layer of hidden nodes is composed of view-specific and shared hidden nodes, in order to learn individual and shared hidden spaces from multiple views of data. View-specific hidden nodes are connected to corresponding view-specific hidden nodes in the lower-layer or visible nodes involving a specific view, whereas shared hidden nodes follow inter-layer connections without restrictions as in standard DBNs. RDBN is trained using layer-wise contrastive divergence learning. Numerical experiments on synthetic and real-world datasets demonstrate the useful behavior of the RDBN, compared to the multi-wing harmonium (MWH) which is a two-layer undirected model.
更多查看译文
关键词
hidden node,deep belief network,visible node,View-specific hidden node,corresponding view-specific hidden node,layer-wise contrastive divergence learning,layers obey harmonium,multi-view learning,multi-wing harmonium,multiple layer,restricted deep belief network
AI 理解论文
溯源树
样例
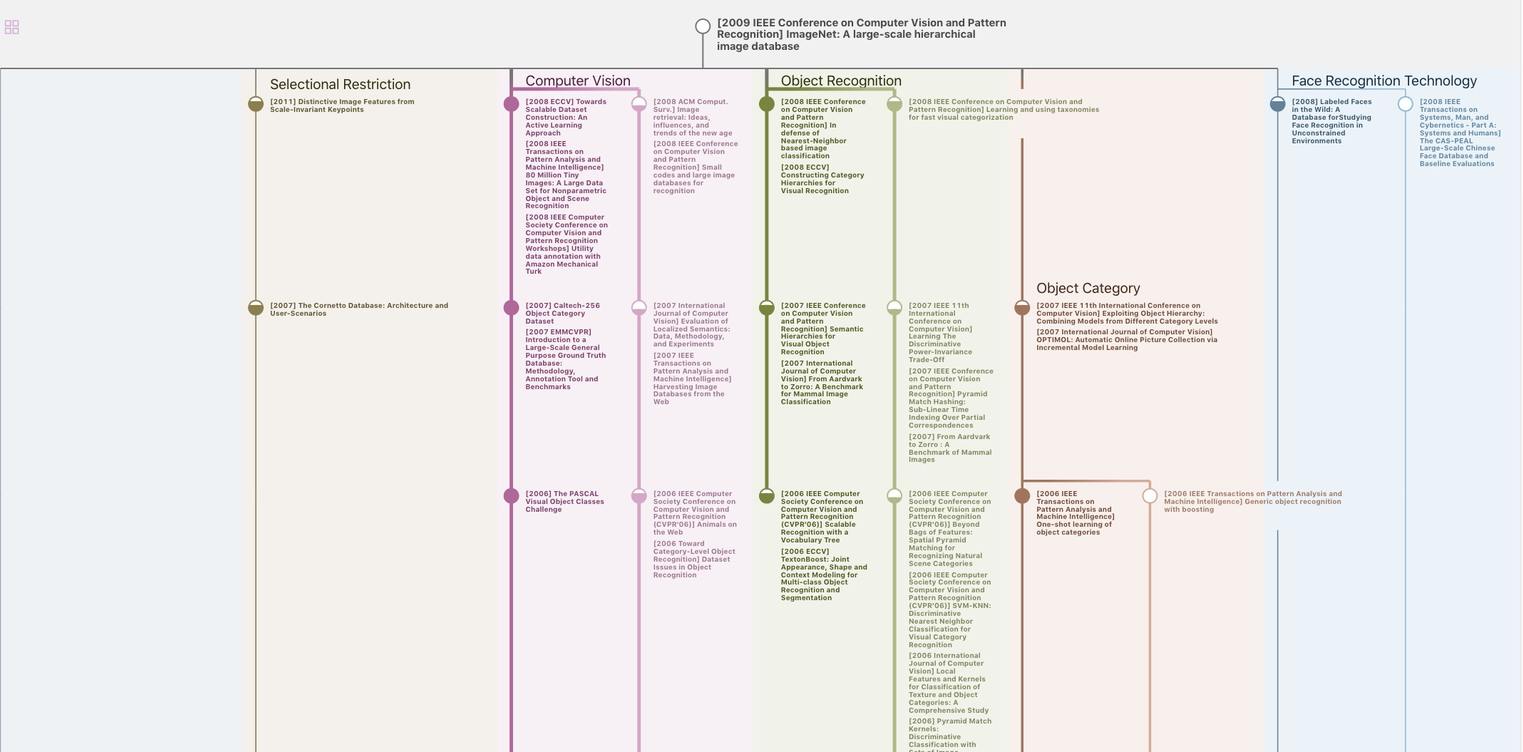
生成溯源树,研究论文发展脉络
Chat Paper
正在生成论文摘要