Compressed sensing of EEG using a random sampling ADC in 90nm CMOS.
BSN(2013)
摘要
Wireless physiological sensors are often limited by energy consumption of the hardware. Power consumption is typically related to the amount of data being transmitted, conventionally the Nyquist rate which is twice the bandwidth of the signal. However, if the signals are sparse in a known basis, compressed sensing facilitates accurate reconstruction of data when sampled below the Nyquist rate. Thus, power consumption at the sensor node could be improved, which would allow long-term use of wireless physiological sensors. We have implemented a random sampling based compressed analog to information converter (AIC) in 90nm CMOS technology. Sufficiently sparse signals were reconstructed using the ℓ1-minimization algorithm. Here we present experimental results that demonstrate reconstruction of non-sparse signals, in this case EEG, by using an ℓ1, 2 regularization algorithm exploiting group sparsity. These results demonstrate the performance achievable by physical compressed sensing AIC systems for brain computer interface applications.
更多查看译文
关键词
wireless sensor networks,wireless communication,sensors,electroencephalography,compressed sensing,signal to noise ratio
AI 理解论文
溯源树
样例
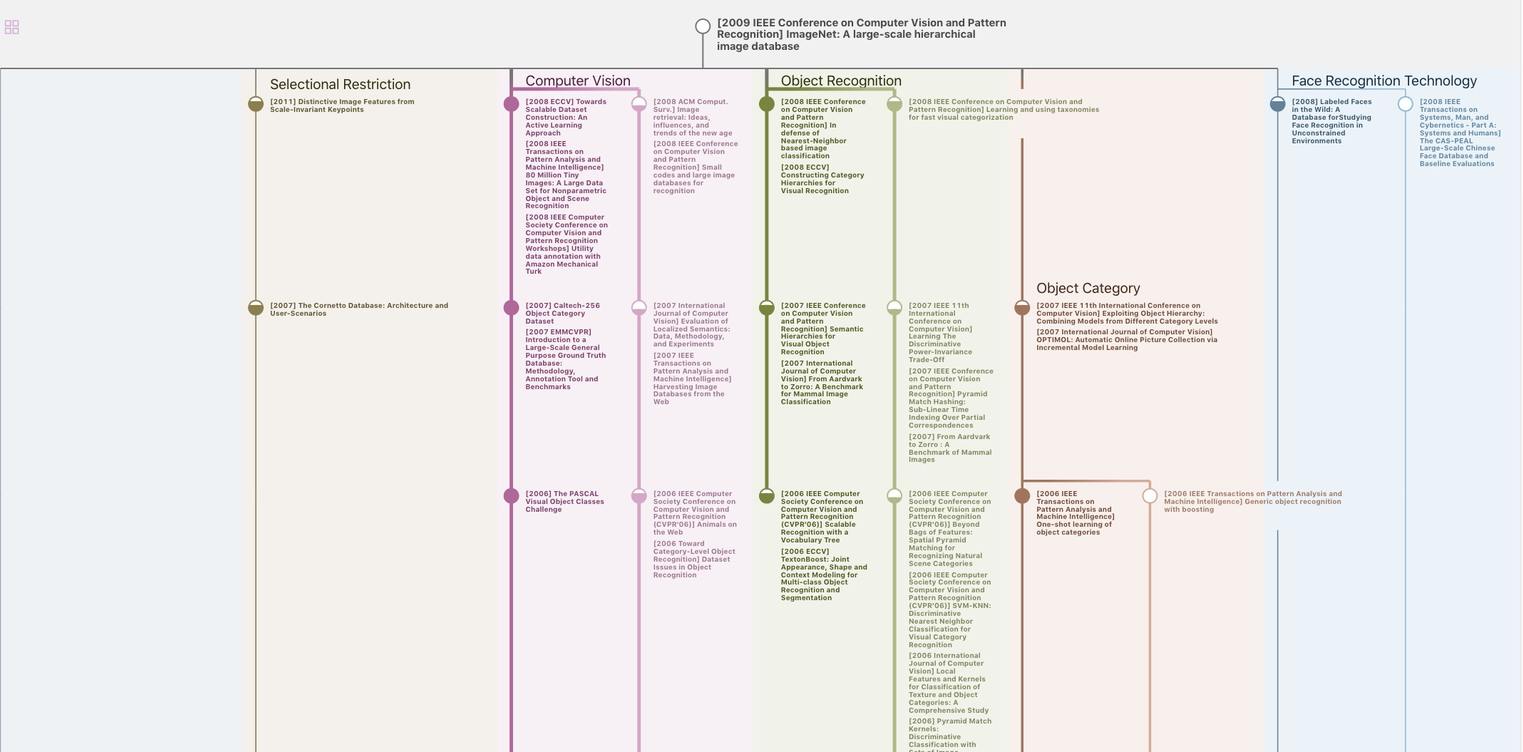
生成溯源树,研究论文发展脉络
Chat Paper
正在生成论文摘要