An approach to meta feature selection
CCECE(2013)
摘要
Many methods, such as mutual information (MI), document frequency (DF), information gain (IG) and χ2 statistics (CHI) algorithm, have been discussed and applied to the study of meta feature selection. This paper gives a brief review of the recent approaches on this topic. By summarizing and synthesizing these approaches, we propose a framework of the application of meta feature selections, where the classical algorithm on attribute reduction is used for data preprocessing and the support vector machine (SVM) algorithm is used for the text classification. The proposed framework has advantages in both effectiveness and accuracy, i.e., our approach decreases the dimension of the text feature space and, at the same time, improves the accuracy of text classification. The experimental results confirm this conclusion.
更多查看译文
关键词
document frequency,data preprocessing,rough set,learning (artificial intelligence),meta feature selection,text classification,support vector machine algorithm,svm algorithm,information gain,attribute reduction,text feature space,χ2 statistics algorithm,mutual information,text analysis,support vector machines,accuracy,learning artificial intelligence,classification algorithms,rough sets
AI 理解论文
溯源树
样例
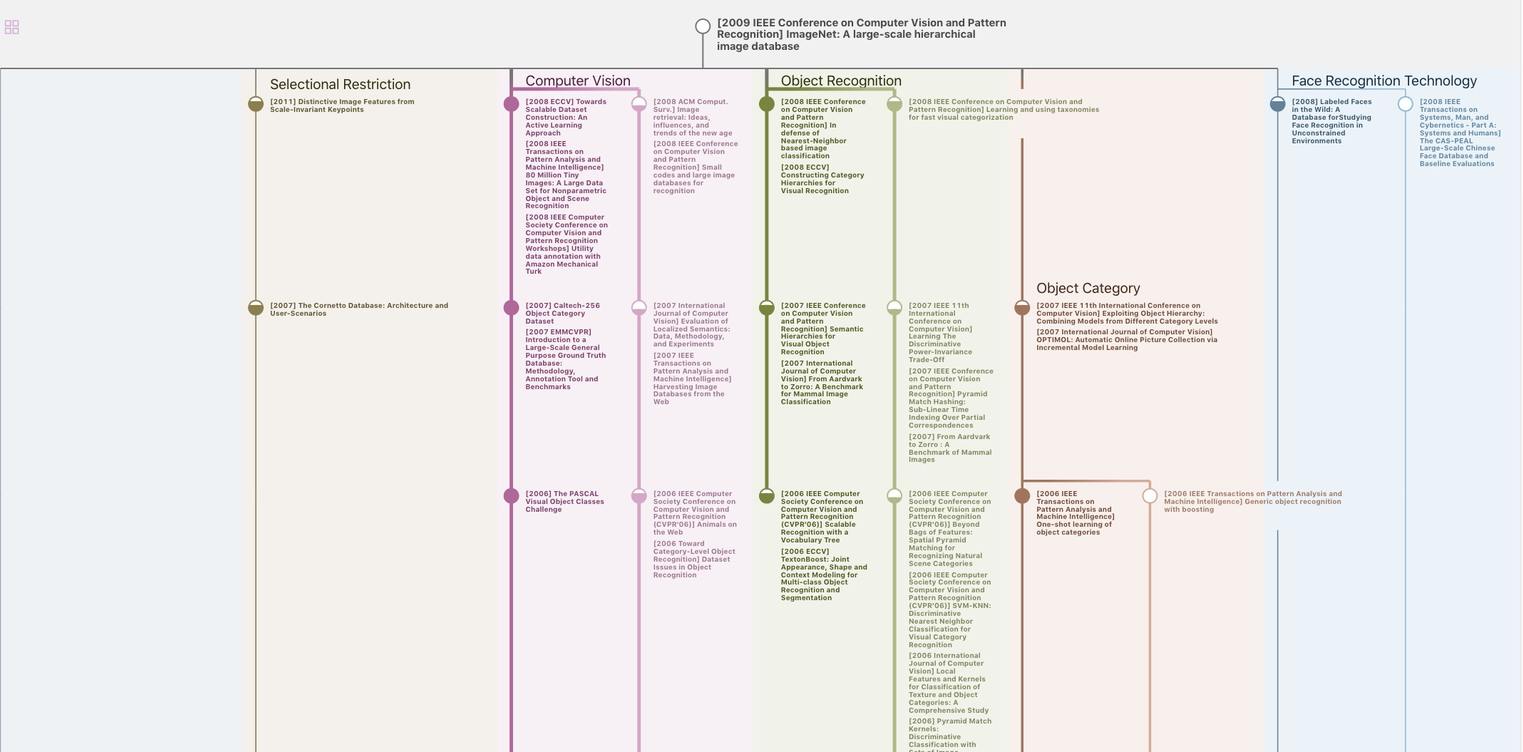
生成溯源树,研究论文发展脉络
Chat Paper
正在生成论文摘要