Robust Optimization for Hybrid MDPs with State-Dependent Noise.
IJCAI '13: Proceedings of the Twenty-Third international joint conference on Artificial Intelligence(2013)
摘要
Recent advances in solutions to Hybrid MDPs with discrete and continuous state and action spaces have significantly extended the class of MDPs for which exact solutions can be derived, albeit at the expense of a restricted transition noise model. In this paper, we work around limitations of previous solutions by adopting a robust optimization approach in which Nature is allowed to adversarially determine transition noise within pre-specified confidence intervals. This allows one to derive an optimal policy with an arbitrary (user-specified) level of success probability and significantly extends the class of transition noise models for which Hybrid MDPs can be solved. This work also significantly extends results for the related "chance-constrained" approach in stochastic hybrid control to accommodate state-dependent noise. We demonstrate our approach working on a variety of hybrid MDPs taken from AI planning, operations research, and control theory, noting that this is the first time robust solutions with strong guarantees over all states have been automatically derived for such problems.
更多查看译文
关键词
Hybrid MDPs,restricted transition noise model,state-dependent noise,transition noise,transition noise model,robust optimization approach,control theory,stochastic hybrid control,time robust solution,AI planning,hybrid MDPs
AI 理解论文
溯源树
样例
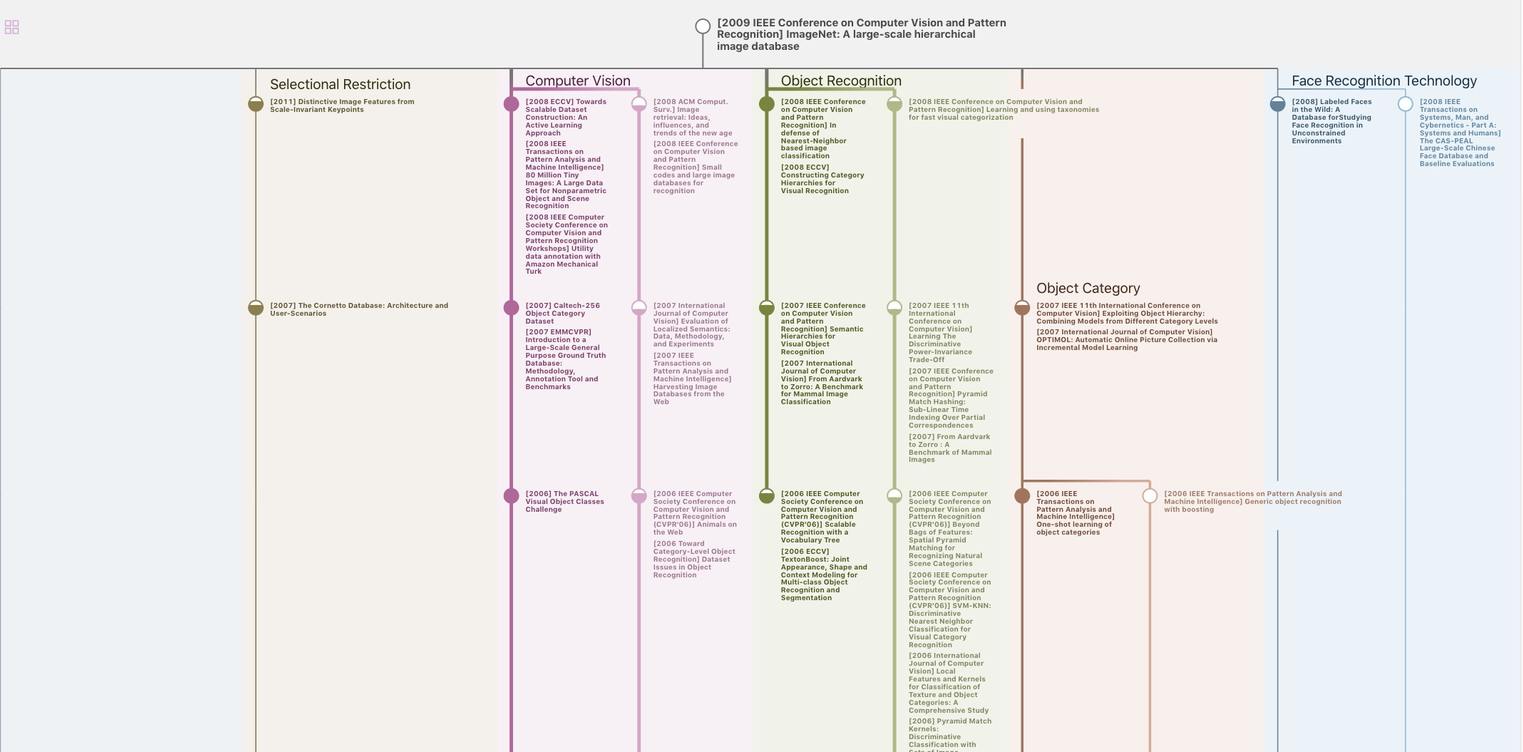
生成溯源树,研究论文发展脉络
Chat Paper
正在生成论文摘要