Using external aggregate ratings for improving individual recommendations
TWEB(2011)
摘要
This article describes an approach for incorporating externally specified aggregate ratings information into certain types of recommender systems, including two types of collaborating filtering and a hierarchical linear regression model. First, we present a framework for incorporating aggregate rating information and apply this framework to the aforementioned individual rating models. Then we formally show that this additional aggregate rating information provides more accurate recommendations of individual items to individual users. Further, we experimentally confirm this theoretical finding by demonstrating on several datasets that the aggregate rating information indeed leads to better predictions of unknown ratings. We also propose scalable methods for incorporating this aggregate information and test our approaches on large datasets. Finally, we demonstrate that the aggregate rating information can also be used as a solution to the cold start problem of recommender systems.
更多查看译文
关键词
unknown rating,recommender system,large datasets,additional aggregate rating information,aggregate information,aforementioned individual rating model,collaborative filtering,individual item,external aggregate rating,aggregate rating information,aggregate ratings information,hierarchical linear models,individual recommendation,cold-start problem,recommender systems,predictive models,aggregate ratings,individual user,prediction model,cold start problem,linear regression model,hierarchical linear model
AI 理解论文
溯源树
样例
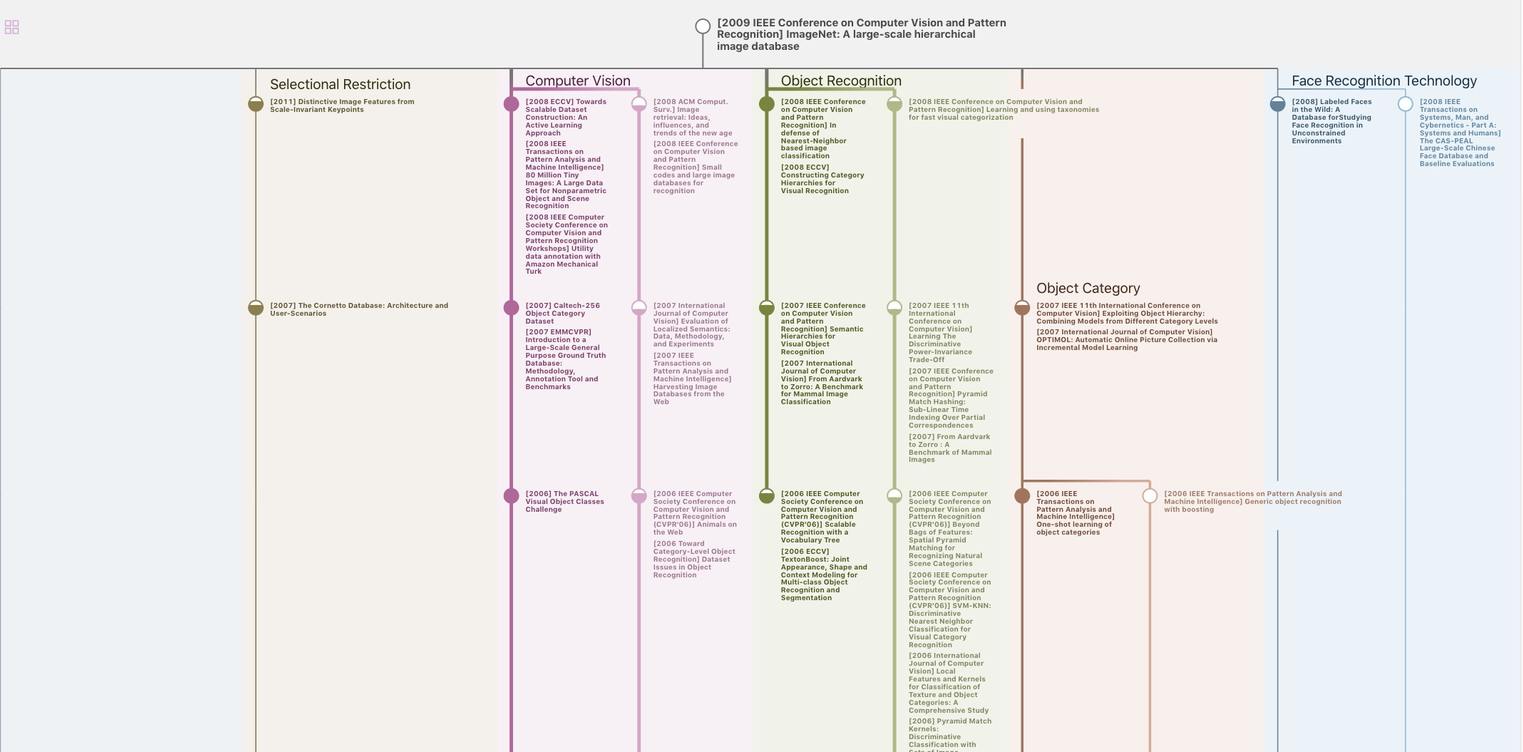
生成溯源树,研究论文发展脉络
Chat Paper
正在生成论文摘要