Efficient Spatial Pattern Analysis for Variation Decomposition Via Robust Sparse Regression
IEEE Transactions on Computer-Aided Design of Integrated Circuits and Systems(2013)
摘要
In this paper, we propose a new technique to achieve accurate decomposition of process variation by efficiently performing spatial pattern analysis. We demonstrate that the spatially correlated systematic variation can be accurately represented by the linear combination of a small number of templates. Based on this observation, an efficient sparse regression algorithm is developed to accurately extract the most adequate templates to represent spatially correlated variation. In addition, a robust sparse regression algorithm is proposed to automatically remove measurement outliers. We further develop a fast numerical algorithm that may reduce the computational time by several orders of magnitude over the traditional direct implementation. Our experimental results based on both synthetic and silicon data demonstrate that the proposed sparse regression technique can capture spatially correlated variation patterns with high accuracy and efficiency.
更多查看译文
关键词
process variation,spatial variation,spatially correlated variation patterns,regression analysis,process variation decomposition,variation decomposition,cmos integrated circuits,silicon,measurement outliers,integrated circuit design,integrated circuit,spatially correlated systematic variation,robust sparse regression,spatial pattern analysis
AI 理解论文
溯源树
样例
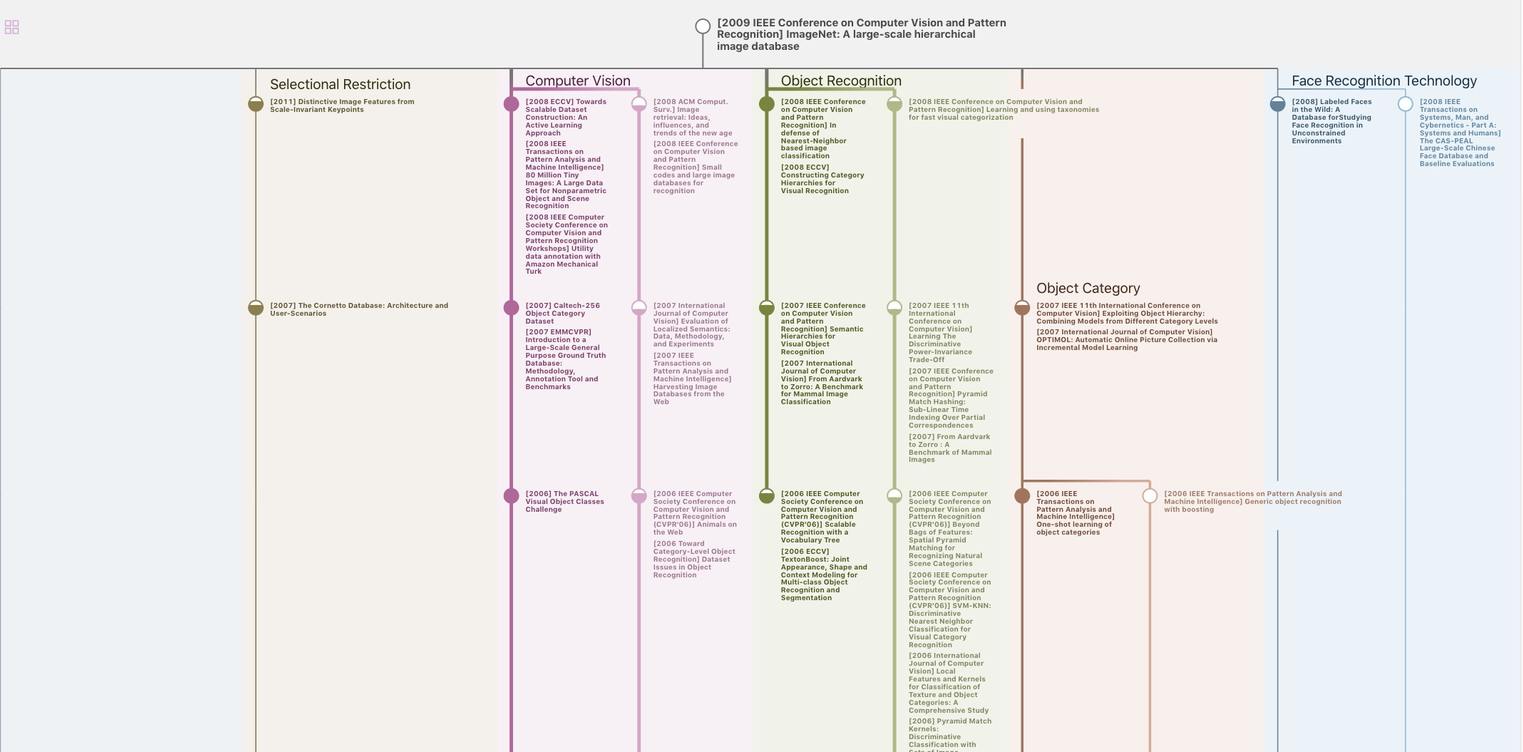
生成溯源树,研究论文发展脉络
Chat Paper
正在生成论文摘要