Score distributions for Pseudo Relevance Feedback.
Information Sciences(2014)
摘要
Relevance-Based Language Models, commonly known as Relevance Models, are successful approaches to explicitly introduce the concept of relevance in the statistical language modelling framework of Information Retrieval. These models achieve state-of-the-art retrieval performance in the Pseudo Relevance Feedback task. It is known that one of the factors that more affect to the Pseudo Relevance Feedback robustness is the selection for some queries of harmful expansion terms. In order to minimise this effect in these methods a crucial point is to reduce the number of non-relevant documents in the pseudo relevant set. In this paper, we propose an original approach to tackle this problem. We try to automatically determine for each query how many documents we should select as pseudo-relevant set. For achieving this objective we will study the score distributions of the initial retrieval and trying to discern in base of their distribution between relevant and non-relevant documents. Evaluation of our proposal showed important improvements in terms of robustness.
更多查看译文
关键词
Information Retrieval,Pseudo Relevance Feedback,Score distribution,Pseudo Relevance Feedback set,Relevance Model
AI 理解论文
溯源树
样例
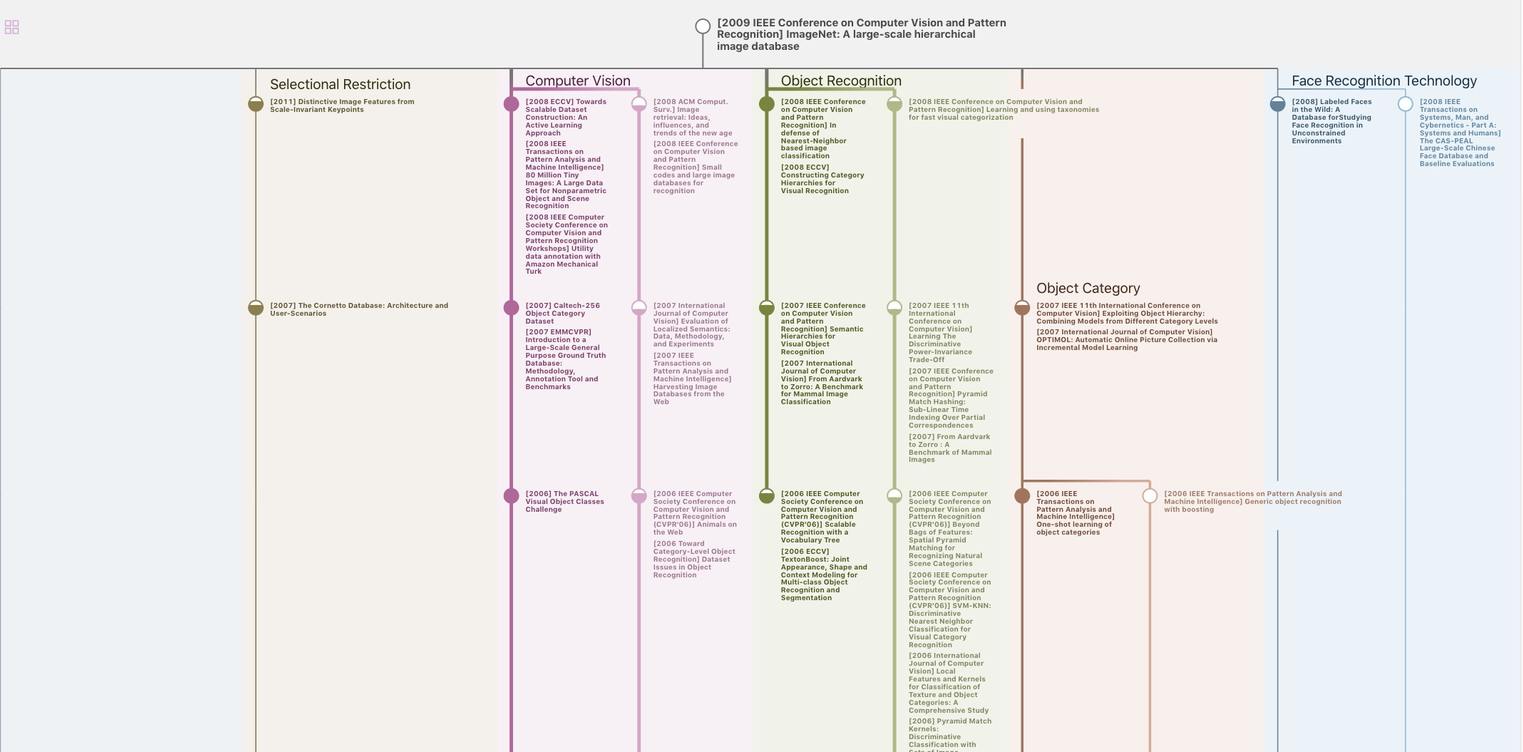
生成溯源树,研究论文发展脉络
Chat Paper
正在生成论文摘要