Business email classification using incremental subspace learning
ICPR(2012)
摘要
We consider a new text classification task: classifying enterprise email messages into sensitive business topics. The identification of sensitive topics in email messages is important for enterprises to safeguard their critical data such as intellectual properties and trade secrets. We introduce the incremental PCA (Principal Component Analysis) to email representation, which can learn a feature subspace incrementally and effectively to reduce the feature dimensionality. Linear SVM (Support Vector Machine) is then adopted to learn the classification models. We validate our approaches with 5,000 emails extracted from the Enron Email set. Experimental results show that SVM outperforms other classification methods, and the incremental PCA produces a substantial reduction in the processing time and a slight increase in the classification accuracy compared to SVM with all the features.
更多查看译文
关键词
incremental pca,text classification task,classification models,linear support vector machine,sensitive business topics,email extraction,sensitive topic identification,learning (artificial intelligence),pattern classification,linear svm,trade secrets,feature extraction,business email classification,intellectual properties,feature dimensionality,incremental principal component analysis,incremental subspace learning,electronic mail,feature subspace,text analysis,principal component analysis,substantial reduction,email representation,support vector machines,enterprise email message classification,security of data,enron email set,learning artificial intelligence
AI 理解论文
溯源树
样例
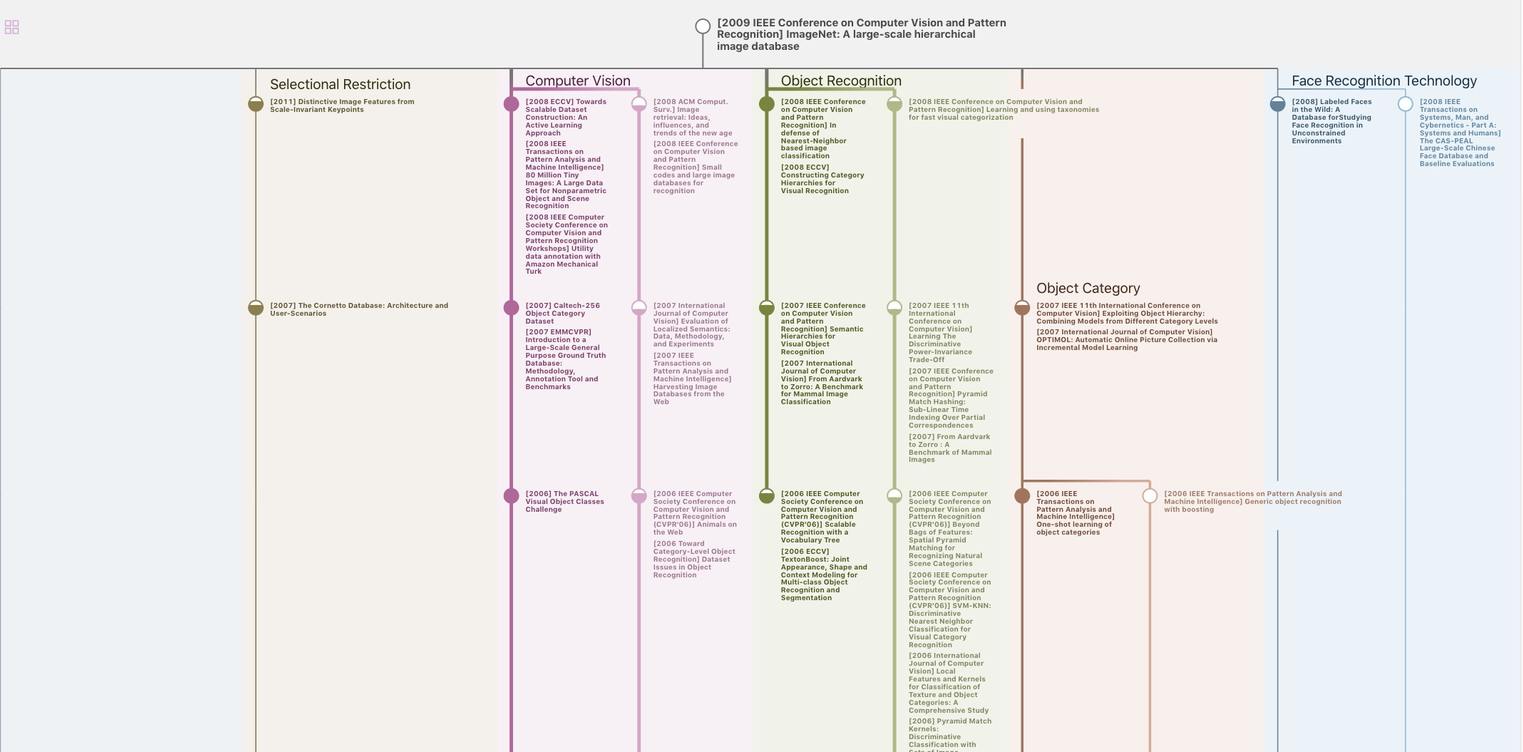
生成溯源树,研究论文发展脉络
Chat Paper
正在生成论文摘要