Combining Video, Audio and Lexical Indicators of Affect in Spontaneous Conversation via Particle Filtering.
ICMI-MLMI(2012)
摘要
We present experiments on fusing facial video, audio and lexical indicators for affect estimation during dyadic conversations. We use temporal statistics of texture descriptors extracted from facial video, a combination of various acoustic features, and lexical features to create regression based affect estimators for each modality. The single modality regressors are then combined using particle filtering, by treating these independent regression outputs as measurements of the affect states in a Bayesian filtering framework, where previous observations provide prediction about the current state by means of learned affect dynamics. Tested on the Audio-visual Emotion Recognition Challenge dataset, our single modality estimators achieve substantially higher scores than the official baseline method for every dimension of affect. Our filtering-based multi-modality fusion achieves correlation performance of 0.344 (baseline: 0.136) and 0.280 (baseline: 0.096) for the fully continuous and word level sub challenges, respectively.
更多查看译文
关键词
adaboost,affective computing,class-spectral features,emotion dynamics,emotion recognition,lexical analysis,local binary patterns,multi-modality fusion,particle filtering,svm
AI 理解论文
溯源树
样例
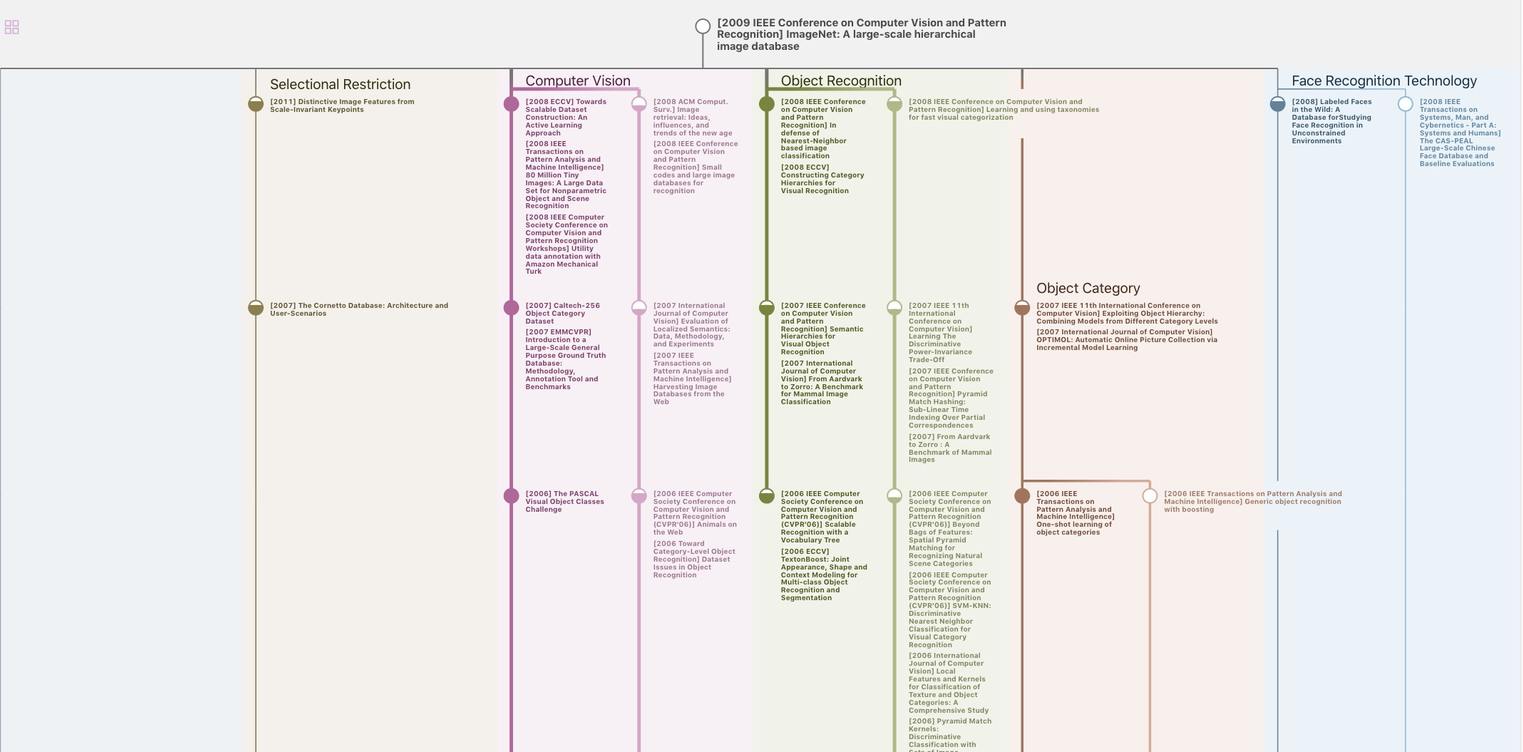
生成溯源树,研究论文发展脉络
Chat Paper
正在生成论文摘要